Posts Tagged Amazon Web Services
Streaming Data on AWS: Amazon Kinesis Data Streams or Amazon MSK?
Posted by Gary A. Stafford in Analytics, AWS, Big Data, Serverless on April 23, 2023
Given similar functionality, what differences make one AWS-managed streaming service a better choice over the other?

Data streaming has emerged as a powerful tool in the last few years thanks to its ability to quickly and efficiently process large volumes of data, provide real-time insights, and scale and adapt to meet changing needs. As IoT, social media, and mobile devices continue to generate vast amounts of data, it has become imperative to have platforms that can handle the real-time ingestion, processing, and analysis of this data.
Key Differentiators
Amazon Kinesis Data Streams and Amazon Managed Streaming for Apache Kafka (Amazon MSK) are two managed streaming services offered by AWS. While both platforms offer similar features, choosing the right service largely depends on your specific use cases and business requirements.
Amazon Kinesis Data Streams
- Simplicity: Kinesis Data Streams is generally considered a less complicated service than Amazon MSK, which requires you to manage more of the underlying infrastructure. This can make setting up and managing your streaming data pipeline easier, especially if you have limited experience with Apache Kafka. Amazon MSK Serverless, which went GA in April 2022, is a cluster type for Amazon MSK that allows you to run Apache Kafka without managing and scaling cluster capacity. Unlike Amazon MSK provisioned, Amazon MSK Serverless greatly reduces the effort required to use Amazon MSK, making ‘Simplicity’ less of a Kinesis differentiator.
- Integration with AWS services: Kinesis Data Streams integrates well with other AWS services, such as AWS Lambda, Amazon S3, and Amazon OpenSearch. This can make building end-to-end data processing pipelines easier using these services.
- Low latency: Kinesis Data Streams is designed to deliver low-latency processing of streaming data, which can be important for applications that require near real-time processing.
- Predictable pricing: Kinesis Data Streams is generally considered to have a more predictable pricing model than Amazon MSK, based on instance sizes and hourly usage. With Kinesis Data Streams, you pay for the data you process, making estimating and managing fees easier (additional fees may apply).

Amazon MSK
- Compatibility with Apache Kafka: Amazon MSK may be a better choice if you have an existing Apache Kafka deployment or are already familiar with Kafka. Amazon MSK is a fully managed version of Apache Kafka, which you can use with existing Kafka applications and tools.
- Customization: With Amazon MSK, you have more control over the underlying cluster infrastructure, configuration, deployment, and version of Kafka, which means you can customize the cluster to meet your needs. This can be important if you have specialized requirements or want to optimize performance (e.g., high-volume financial trading, real-time gaming).
- Larger ecosystem: Apache Kafka has a large ecosystem of tools and integrations compared to Kinesis Data Streams. This can provide flexibility and choice when building and managing your streaming data pipeline. Some common tools include MirrorMaker, Kafka Connect, LinkedIn’s Cruise Control, kcat (fka kafkacat), Lenses, Confluent Schema Registry, and Appicurio Registry.
- Preference for Open Source: You may prefer the flexibility, transparency, pace of innovation, and interoperability of employing open source software (OSS) over proprietary software and services for your streaming solution.

Ultimately, the choice between Amazon Kinesis Data Streams and Amazon MSK will depend on your specific needs and priorities. Kinesis Data Streams might be better if you prioritize simplicity, integration with other AWS services, and low latency. If you have an existing Kafka deployment, require more customization, or need access to a larger ecosystem of tools and integrations, Amazon MSK might be a better fit. In my opinion, the newer Amazon MSK Serverless option lessens several traditional differentiators between the two services.
Scaling Capabilities
Amazon Kinesis Data Streams and Amazon MSK are designed to be scalable streaming services that can handle large volumes of data. However, there are some differences in their scaling capabilities.
Amazon Kinesis Data Streams
- Scalability: Kinesis Data Streams has two capacity modes, on-demand and provisioned. With the on-demand mode, Kinesis Data Streams automatically manages the shards to provide the necessary throughput based on the amount of data you process. This means the service can automatically adjust the number of shards based on the incoming data volume, allowing you to handle increased traffic without manually adjusting the infrastructure.
- Limitations: Per the documentation, there is no upper quota on the number of streams with the provisioned mode you can have in an account. A shard can ingest up to 1 MB of data per second (including partition keys) or 1,000 records per second for writes. The maximum size of the data payload of a record before base64-encoding is up to 1 MB. GetRecords can retrieve up to 10 MB of data per call from a single shard and up to 10,000 records per call. Each call to GetRecords is counted as one read transaction. Each shard can support up to five read transactions per second. Each read transaction can provide up to 10,000 records with an upper quota of 10 MB per transaction. Each shard can support a maximum total data read rate of 2 MB per second via GetRecords. If a call to GetRecords returns 10 MB, subsequent calls made within the next 5 seconds throw an exception.
- Cost: Kinesis Data Streams has two capacity modes — on-demand and provisioned — with different pricing models. With on-demand capacity mode, you pay per GB of data written and read from your data streams. You do not need to specify how much read and write throughput you expect your application to perform. With provisioned capacity mode, you select the number of shards necessary for your application based on its write and read request rate. There are additional fees
PUT
Payload Units, enhanced fan-out, extended data retention, and retrieval of long-term retention data.
Amazon MSK
- Scalability: Amazon MSK is designed to be highly scalable and can handle millions of messages per second. With Amazon MSK provisioned, you can scale your Kafka cluster by adding or removing instances (brokers) and storage as needed. Amazon MSK can automatically rebalance partitions across instances. Alternately, Amazon MSK Serverless automatically provisions and scales capacity while managing the partitions in your topic, so you can stream data without thinking about right-sizing or scaling clusters.
- Flexibility: With Amazon MSK, you have more control over the underlying infrastructure, which means you can customize the deployment to meet your needs. This can be important if you have specialized requirements or want to optimize performance.
- Amazon MSK also offers multiple authentication methods. You can use IAM to authenticate clients and to allow or deny Apache Kafka actions. Alternatively, with Amazon MSK provisioned, you can use TLS or SASL/SCRAM to authenticate clients and Apache Kafka ACLs to allow or deny actions.
- Cost: Scaling up or down with Amazon MSK can impact the cost based on instance sizes and hourly usage. Therefore, adding more instances can increase the overall cost of the service. Pricing models for Amazon MSK and Amazon MSK Serverless vary.
Amazon Kinesis Data Streams and Amazon MSK are highly scalable services. Kinesis Data Streams can scale automatically based on the amount of data you process. At the same time, Amazon MSK allows you to scale your Kafka cluster by adding or removing instances and adding storage as needed. However, adding more shards with Kinesis can lead to a more manual process that can take some time to propagate and impact cost, while scaling up or down with Amazon MSK is based on instance sizes and hourly usage. Ultimately, the choice between the two will depend on your specific use case and requirements.
Throughput
Throughput can be measured in the maximum MB/s of data and the maximum number of records per second. The maximum throughput of both Amazon Kinesis Data Streams and Amazon MSK are not hard limits. Depending on the service, you can exceed these limits by adding more resources, including shards or brokers. Total maximum system throughput is affected by the maximum throughput of both upstream and downstream producing and consuming components.
Amazon Kinesis Data Streams
The maximum throughput of Kinesis Data Streams depends on the number of shards and the size of the data being processed. Each shard in a Kinesis stream can handle up to 1 MB/s of data input and up to 2 MB/s of data output, or up to 1,000 records per second for writes and up to 10,000 records per second for reads. When a consumer uses enhanced fan-out, it gets its own 2 MB/s allotment of read throughput, allowing multiple consumers to read data from the same stream in parallel without contending for read throughput with other consumers.
The maximum throughput of a Kinesis stream is determined by the number of shards you have multiplied by the maximum throughput per shard. For example, if you have a stream with 10 shards, the maximum throughput of the stream would be 10 MB/s for data input and 20 MB/s for data output, or up to 10,000 records per second for writes and up to 100,000 records per second for reads.
The maximum throughput is not a hard limit, and you can exceed these limits by adding more shards to your stream. However, adding more shards can impact the cost of the service, and you should consider the optimal shard count for your use case to ensure efficient and cost-effective processing of your data.
Amazon MSK
As discussed in the Amazon MSK best practices documentation, the maximum throughput of Amazon MSK depends on the number of brokers and the instance type of those brokers. Amazon MSK allows you to scale the number of instances in a Kafka cluster up or down based on your needs.
The maximum throughput of an Amazon MSK cluster depends on the number of brokers and the performance characteristics of the instance types you are using. Each broker in an Amazon MSK cluster can handle tens of thousands of messages per second, depending on the instance type and configuration. The actual throughput you can achieve will depend on your specific use case and the message size. The AWS blog post, Best practices for right-sizing your Apache Kafka clusters to optimize performance and cost, is an excellent reference.
The maximum throughput is not a hard limit, and you can exceed these limits by adding more brokers or upgrading to more powerful instances. However, adding more instances or upgrading to more powerful instances can impact the service’s cost. Therefore, consider your use case’s optimal instance count and type to ensure efficient and cost-effective data processing.
Writing Messages
Compatibility with multiple producers and consumers is essential when choosing a streaming technology. There are multiple ways to write messages to Amazon Kinesis Data Streams and Amazon MSK.
Amazon Kinesis Data Streams
- AWS SDK: Use the AWS SDK for your preferred programming language.
- Kinesis Producer Library (KPL): KPL is a high-performance library that allows you to write data to Kinesis Data Streams at a high rate. KPL handles all heavy lifting, including batching, retrying failed records, and load balancing across shards.
- Amazon Kinesis Data Firehose: Kinesis Data Firehose is a fully managed service that can ingest and transform streaming data in real-time. It can be used to write data to Kinesis Data Streams, as well as to other AWS services such as S3, Redshift, and Elasticsearch.
- Amazon Kinesis Data Analytics: Kinesis Data Analytics is a fully managed service that allows you to process and analyze streaming data in real-time. It can read data from Kinesis Data Streams, perform real-time analytics and transformations, and write the results to another Kinesis stream or an external data store.
- Kinesis Agent: Kinesis Agent is a standalone Java application that collects and sends data to Kinesis Data Streams. It can monitor log files or other data sources and automatically send data to Kinesis Data Streams as it is generated.
- Third-party libraries and tools: There are many third-party libraries and tools available for writing data to Kinesis Data Streams, including Apache Kafka Connect, Apache Storm, and Fluentd. These tools can integrate Kinesis Data Streams with existing data processing pipelines or build custom streaming applications.

Amazon MSK
- Kafka command line tools: The Kafka command line tools (e.g.,
kafka-console-producer.sh
) can be used to write messages to a Kafka topic in an Amazon MSK cluster. These tools are part of the Kafka distribution and are pre-installed on the Amazon MSK broker nodes. - Kafka client libraries: You can use Kafka client libraries in your preferred programming language (e.g., Java, Python, C#) to write messages to an Amazon MSK cluster. These libraries provide a more flexible and customizable way to produce messages to Kafka topics.
- AWS SDKs: You can use AWS SDKs (e.g., AWS SDK for Java, AWS SDK for Python) to interact with Amazon MSK and write messages to Kafka topics. These SDKs provide a higher-level abstraction over the Kafka client libraries, making integrating Amazon MSK into your AWS infrastructure easier.
- Third-party libraries and tools: There are many third-party tools and frameworks, including Apache NiFi, Apache Camel, and Apache Beam. They provide Kafka connectors and producers, which can be used to write messages to Kafka topics in Amazon MSK. These tools can simplify the process of writing messages and provide additional features such as data transformation and routing.
Schema Registry
You can use AWS Glue Schema Registry with Amazon Kinesis Data Streams and Amazon MSK. AWS Glue Schema Registry is a fully managed service that provides a central schema repository for organizing, validating, and tracking the evolution of your data schemas. It enables you to store, manage, and discover schemas for your data in a single, centralized location.
With AWS Glue Schema Registry, you can define and register schemas for your data in the registry. You can then use these schemas to validate the data being ingested into your streaming applications, ensuring that the data conforms to the expected structure and format.
Both Kinesis Data Streams and Amazon MSK support the use of AWS Glue Schema Registry through the use of Apache Avro schemas. Avro is a compact, fast, binary data format that can improve the performance of your streaming applications. You can configure your streaming applications to use the registry to validate incoming data, ensuring that it conforms to the schema before processing.
Using AWS Glue Schema Registry can help ensure the consistency and quality of your data across your streaming applications and provide a centralized location for managing and tracking schema changes. Amazon MSK is also compatible with popular alternative schema registries, such as Confluent Schema Registry and RedHat’s open-source Apicurio Registry.

Stream Processing
According to TechTarget, Stream processing is a data management technique that involves ingesting a continuous data stream to quickly analyze, filter, transform, or enhance the data in real-time. Several leading stream processing tools are available, compatible with Amazon Kinesis Data Streams and Amazon MSK. Each tool with its own strengths and use cases. Some of the more popular tools include:
- Apache Flink: Apache Flink is a distributed stream processing framework that provides fast, scalable, and fault-tolerant data processing for real-time and batch data streams. It supports a variety of data sources and sinks and provides a powerful stream processing API and SQL interface. In addition, Amazon offers its managed version of Apache Flink, Amazon Kinesis Data Analytics (KDA), which is compatible with both Amazon Kinesis Data Streams and Amazon MSK.
- Apache Spark Structured Streaming: Apache Spark Structured Streaming is a stream processing framework that allows developers to build real-time stream processing applications using the familiar Spark API. It provides high-level APIs for processing data streams and supports integration with various data sources and sinks. Apache Spark is compatible with both Amazon Kinesis Data Streams and Amazon MSK. Spark Streaming is available as a managed service on AWS via AWS Glue Studio and Amazon EMR.
- Apache NiFi: Apache NiFi is an open-source data integration and processing tool that provides a web-based UI for building data pipelines. It supports batch and stream processing and offers a variety of processors for data ingestion, transformation, and delivery. Apache NiFi is compatible with both Amazon Kinesis Data Streams and Amazon MSK.
- Amazon Kinesis Data Firehose (KDA): Kinesis Data Firehose is a fully managed service that can ingest and transform streaming data in real time. It can be used to write data to Kinesis Data Streams, as well as to other AWS services such as S3, Redshift, and Elasticsearch. Kinesis Data Firehose is compatible with Amazon Kinesis Data Streams and Amazon MSK.
- Apache Kafka Streams (aka KStream): Apache Kafka Streams is a lightweight stream processing library that allows developers to build scalable and fault-tolerant real-time applications and microservices. KStreams integrates seamlessly with Amazon MSK and provides a high-level DSL for stream processing.
- ksqlDB: ksqlDB is a database for building stream processing applications on top of Apache Kafka. It is distributed, scalable, reliable, and real-time. ksqlDB combines the power of real-time stream processing with the approachable feel of a relational database through a familiar, lightweight SQL syntax. ksqlDB is compatible with Amazon MSK.
Several stream-processing tools are detailed in my recent two-part blog post, Exploring Popular Open-source Stream Processing Technologies.
Conclusion
Amazon Kinesis Data Streams and Amazon Managed Streaming for Apache Kafka (Amazon MSK) are managed streaming services. While they offer similar functionality, some differences might make one a better choice, depending on your use cases and experience. Ensure you understand your streaming requirements and each service’s capabilities before making a final architectural decision.
🔔 To keep up with future content, follow Gary Stafford on LinkedIn.
This blog represents my viewpoints and not those of my employer, Amazon Web Services (AWS). All product names, logos, and brands are the property of their respective owners.
Ten Ways to Leverage Generative AI for Development on AWS
Posted by Gary A. Stafford in AI/ML, AWS, Bash Scripting, Big Data, Build Automation, Client-Side Development, Cloud, DevOps, Enterprise Software Development, Kubernetes, Python, Serverless, Software Development, SQL on April 3, 2023
Explore ten ways you can use Generative AI coding tools to accelerate development and increase your productivity on AWS
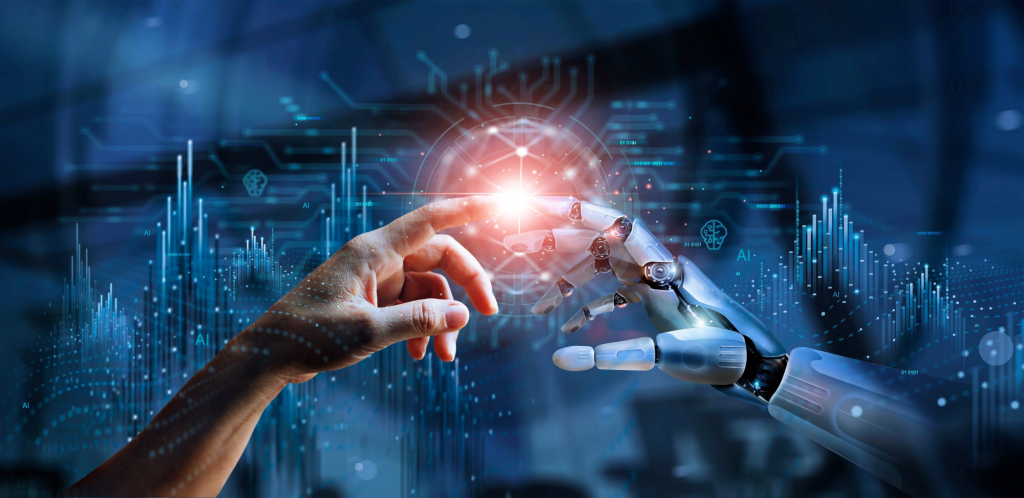
Generative AI coding tools are a new class of software development tools that leverage machine learning algorithms to assist developers in writing code. These tools use AI models trained on vast amounts of code to offer suggestions for completing code snippets, writing functions, and even entire blocks of code.
Quote generated by OpenAI ChatGPT
Introduction
Combining the latest Generative AI coding tools with a feature-rich and extensible IDE and your coding skills will accelerate development and increase your productivity. In this post, we will look at ten examples of how you can use Generative AI coding tools on AWS:
- Application Development: Code, unit tests, and documentation
- Infrastructure as Code (IaC): AWS CloudFormation, AWS CDK, Terraform, and Ansible
- AWS Lambda: Serverless, event-driven functions
- IAM Policies: AWS IAM policies and Amazon S3 bucket policies
- Structured Query Language (SQL): Amazon RDS, Amazon Redshift, Amazon Athena, and Amazon EMR
- Big Data: Apache Spark and Flink on Amazon EMR, AWS Glue, and Kinesis Data Analytics
- Configuration and Properties files: Amazon MSK, Amazon EMR, and Amazon OpenSearch
- Apache Airflow DAGs: Amazon MWAA
- Containerization: Kubernetes resources, Helm Charts, Dockerfiles for Amazon EKS
- Utility Scripts: PowerShell, Bash, Shell, and Python
Choosing a Generative AI Coding Tool
In my recent post, Accelerating Development with Generative AI-Powered Coding Tools, I reviewed six popular tools: ChatGPT, Copilot, CodeWhisperer, Tabnine, Bing, and ChatSonic.
For this post, we will use GitHub Copilot, powered by OpenAI Codex, a new AI system created by OpenAI. Copilot suggests code and entire functions in real-time, right from your IDE. Copilot is trained in all languages that appear in GitHub’s public repositories. GitHub points out that the quality of suggestions you receive may depend on the volume and diversity of training data for that language. Similar tools in this category are limited in the number of languages they support compared to Copilot.

Copilot is currently available as an extension for Visual Studio Code, Visual Studio, Neovim, and JetBrains suite of IDEs. The GitHub Copilot extension for Visual Studio Code (VS Code) already has 4.8 million downloads, and the GitHub Copilot Nightly extension, used for this post, has almost 280,000 downloads. I am also using the GitHub Copilot Labs extension in this post.

Ten Ways to Leverage Generative AI
Take a look at ten examples of how you can use Generative AI coding tools to increase your development productivity on AWS. All the code samples in this post can be found on GitHub.
1. Application Development
According to GitHub, trained on billions of lines of code, GitHub Copilot turns natural language prompts into coding suggestions across dozens of languages. These features make Copilot ideal for developing applications, writing unit tests, and authoring documentation. You can use GitHub Copilot to assist with writing software applications in nearly any popular language, including Go.
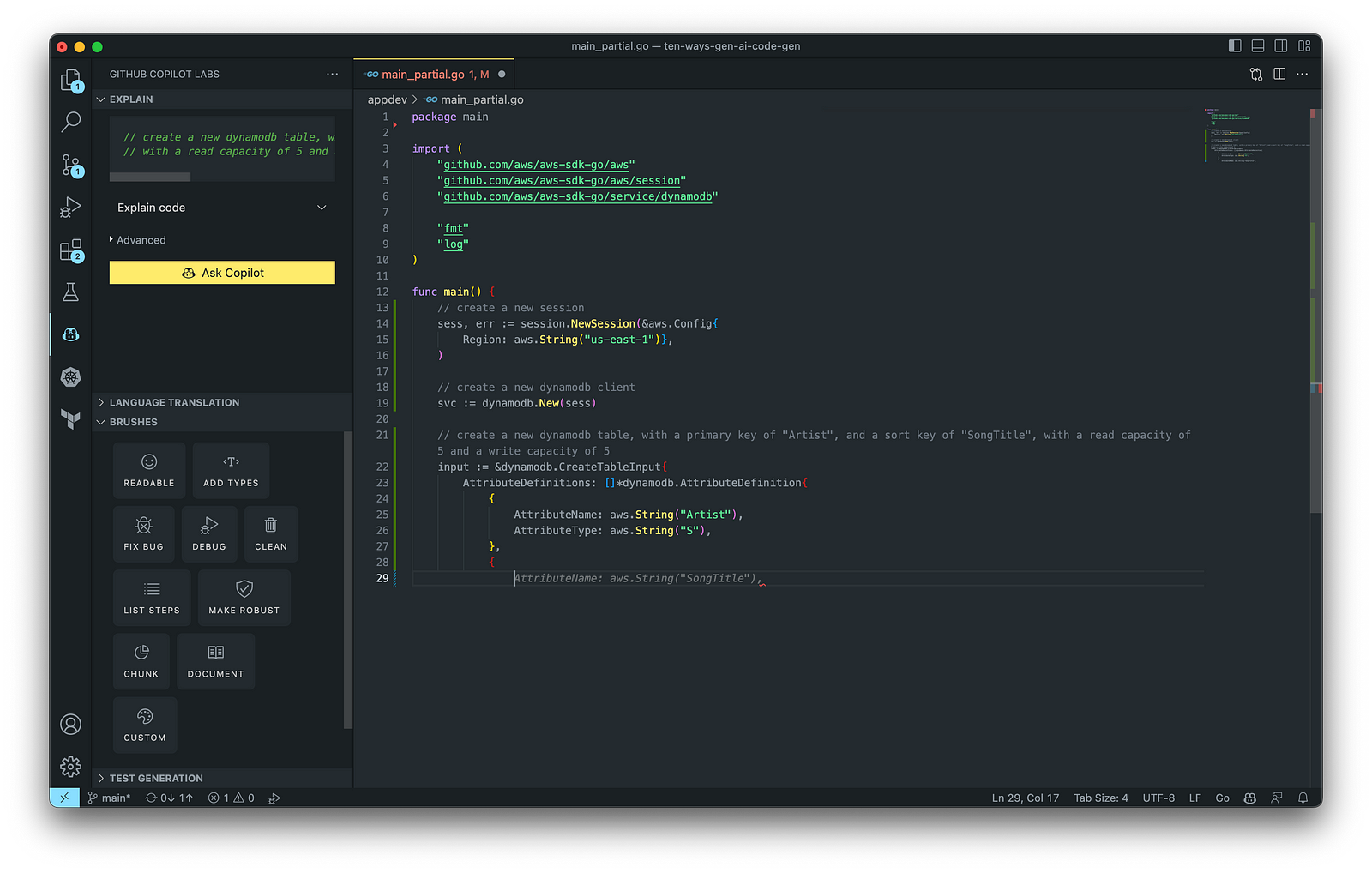
The final application, which uses the AWS SDK for Go to create an Amazon DynamoDB table, shown below, was formatted using the Go extension by Google and optimized using the ‘Readable,’ ‘Make Robust,’ and ‘Fix Bug’ GitHub Code Brushes.

Generating Unit Tests
Using JavaScript and TypeScript, you can take advantage of TestPilot to generate unit tests based on your existing code and documentation. TestPilot, part of GitHub Copilot Labs, uses GitHub Copilot’s AI technology.

2. Infrastructure as Code (IaC)
Widespread Infrastructure as Code (IaC) tools include Pulumi, AWS CloudFormation, Azure ARM Templates, Google Deployment Manager, AWS Cloud Development Kit (AWS CDK), Microsoft Bicep, and Ansible. Many IaC tools, except AWS CDK, use JSON- or YAML-based domain-specific languages (DSLs).
AWS CloudFormation
AWS CloudFormation is an Infrastructure as Code (IaC) service that allows you to easily model, provision, and manage AWS and third-party resources. The CloudFormation template is a JSON or YAML formatted text file. You can use GitHub Copilot to assist with writing IaC, including AWS CloudFormation in either JSON or YAML.

You can use the YAML Language Support by Red Hat extension to write YAML in VS Code.

VS Code has native JSON support with JSON Schema Store, which includes AWS CloudFormation. VS Code uses the CloudFormation schema for IntelliSense and flag schema errors in templates.

HashiCorp Terraform
In addition to AWS CloudFormation, HashiCorp Terraform is an extremely popular IaC tool. According to HashiCorp, Terraform lets you define resources and infrastructure in human-readable, declarative configuration files and manages your infrastructure’s lifecycle. Using Terraform has several advantages over manually managing your infrastructure.
Terraform plugins called providers let Terraform interact with cloud platforms and other services via their application programming interfaces (APIs). You can use the AWS Provider to interact with the many resources supported by AWS.

3. AWS Lambda
Lambda, according to AWS, is a serverless, event-driven compute service that lets you run code for virtually any application or backend service without provisioning or managing servers. You can trigger Lambda from over 200 AWS services and software as a service (SaaS) applications and only pay for what you use. AWS Lambda natively supports Java, Go, PowerShell, Node.js, C#, Python, and Ruby. AWS Lambda also provides a Runtime API allowing you to use additional programming languages to author your functions.
You can use GitHub Copilot to assist with writing AWS Lambda functions in any of the natively supported languages. You can further optimize the resulting Lambda code with GitHub’s Code Brushes.

The final Python-based AWS Lambda, below, was formatted using the Black Formatter and Flake8 extensions and optimized using the ‘Readable,’ ‘Debug,’ ‘Make Robust,’ and ‘Fix Bug’ GitHub Code Brushes.

You can easily convert the Python-based AWS Lambda to Java using GitHub Copilot Lab’s ability to translate code between languages. Install the GitHub Copilot Labs extension for VS Code to try out language translation.

4. IAM Policies
AWS Identity and Access Management (AWS IAM) is a web service that helps you securely control access to AWS resources. According to AWS, you manage access in AWS by creating policies and attaching them to IAM identities (users, groups of users, or roles) or AWS resources. A policy is an object in AWS that defines its permissions when associated with an identity or resource. IAM policies are stored on AWS as JSON documents. You can use GitHub Copilot to assist in writing IAM Policies.

The final AWS IAM Policy, below, was formatted using VS Code’s built-in JSON support.

5. Structured Query Language (SQL)
SQL has many use cases on AWS, including Amazon Relational Database Service (RDS) for MySQL, PostgreSQL, MariaDB, Oracle, and SQL Server databases. SQL is also used with Amazon Aurora, Amazon Redshift, Amazon Athena, Apache Presto, Trino (PrestoSQL), and Apache Hive on Amazon EMR.
You can use IDEs like VS Code with its SQL dialect-specific language support and formatted extensions. You can further optimize the resulting SQL statements with GitHub’s Code Brushes.

The final PostgreSQL script, below, was formatted using the Sql Formatter extension and optimized using the ‘Readable’ and ‘Fix Bug’ GitHub Code Brushes.

6. Big Data
Big Data, according to AWS, can be described in terms of data management challenges that — due to increasing volume, velocity, and variety of data — cannot be solved with traditional databases. AWS offers managed versions of Apache Spark, Apache Flink, Apache Zepplin, and Jupyter Notebooks on Amazon EMR, AWS Glue, and Amazon Kinesis Data Analytics (KDA).
Apache Spark
According to their website, Apache Spark is a multi-language engine for executing data engineering, data science, and machine learning on single-node machines or clusters. Spark jobs can be written in various languages, including Python (PySpark), SQL, Scala, Java, and R. Apache Spark is available on a growing number of AWS services, including Amazon EMR and AWS Glue.

The final Python-based Apache Spark job, below, was formatted using the Black Formatter extension and optimized using the ‘Readable,’ ‘Document,’ ‘Make Robust,’ and ‘Fix Bug’ GitHub Code Brushes.

7. Configuration and Properties Files
According to TechTarget, a configuration file (aka config) defines the parameters, options, settings, and preferences applied to operating systems, infrastructure devices, and applications. There are many examples of configuration and properties files on AWS, including Amazon MSK Connect (Kafka Connect Source/Sink Connectors), Amazon OpenSearch (Filebeat, Logstash), and Amazon EMR (Apache Log4j, Hive, and Spark).
Kafka Connect
Kafka Connect is a tool for scalably and reliably streaming data between Apache Kafka and other systems. It makes it simple to quickly define connectors that move large collections of data into and out of Kafka. AWS offers a fully-managed version of Kafka Connect: Amazon MSK Connect. You can use GitHub Copilot to write Kafka Connect Source and Sink Connectors with Kafka Connect and Amazon MSK Connect.

The final Kafka Connect Source Connector, below, was formatted using VS Code’s built-in JSON support. It incorporates the Debezium connector for MySQL, Avro file format, schema registry, and message transformation. Debezium is a popular open source distributed platform for performing change data capture (CDC) with Kafka Connect.

8. Apache Airflow DAGs
Apache Airflow is an open-source platform for developing, scheduling, and monitoring batch-oriented workflows. Airflow’s extensible Python framework enables you to build workflows connecting with virtually any technology. DAG (Directed Acyclic Graph) is the core concept of Airflow, collecting Tasks together, organized with dependencies and relationships to say how they should run.
Amazon Managed Workflows for Apache Airflow (Amazon MWAA) is a managed orchestration service for Apache Airflow. You can use GitHub Copilot to assist in writing DAGs for Apache Airflow, to be used with Amazon MWAA.

The final Python-based Apache Spark job, below, was formatted using the Black Formatter extension. Unfortunately, based on my testing, code optimization with GitHub’s Code Brushes is impossible with Airflow DAGs.

9. Containerization
According to Check Point Software, Containerization is a type of virtualization in which all the components of an application are bundled into a single container image and can be run in isolated user space on the same shared operating system. Containers are lightweight, portable, and highly conducive to automation. AWS describes containerization as a software deployment process that bundles an application’s code with all the files and libraries it needs to run on any infrastructure.
AWS has several container services, including Amazon Elastic Container Service (Amazon ECS), Amazon Elastic Kubernetes Service (Amazon EKS), Amazon Elastic Container Registry (Amazon ECR), and AWS Fargate. Several code-based resources can benefit from a Generative AI coding tool like GitHub Copilot, including Dockerfiles, Kubernetes resources, Helm Charts, Weaveworks Flux, and ArgoCD configuration.
Kubernetes
Kubernetes objects are represented in the Kubernetes API and expressed in YAML format. Below is a Kubernetes Deployment resource file, which creates a ReplicaSet to bring up multiple replicas of nginx
Pods.

The final Kubernetes resource file below contains Deployment and Service resources. In addition to GitHub Copilot, you can use Microsoft’s Kubernetes extension for VS Code to use IntelliSense and flag schema errors in the file.
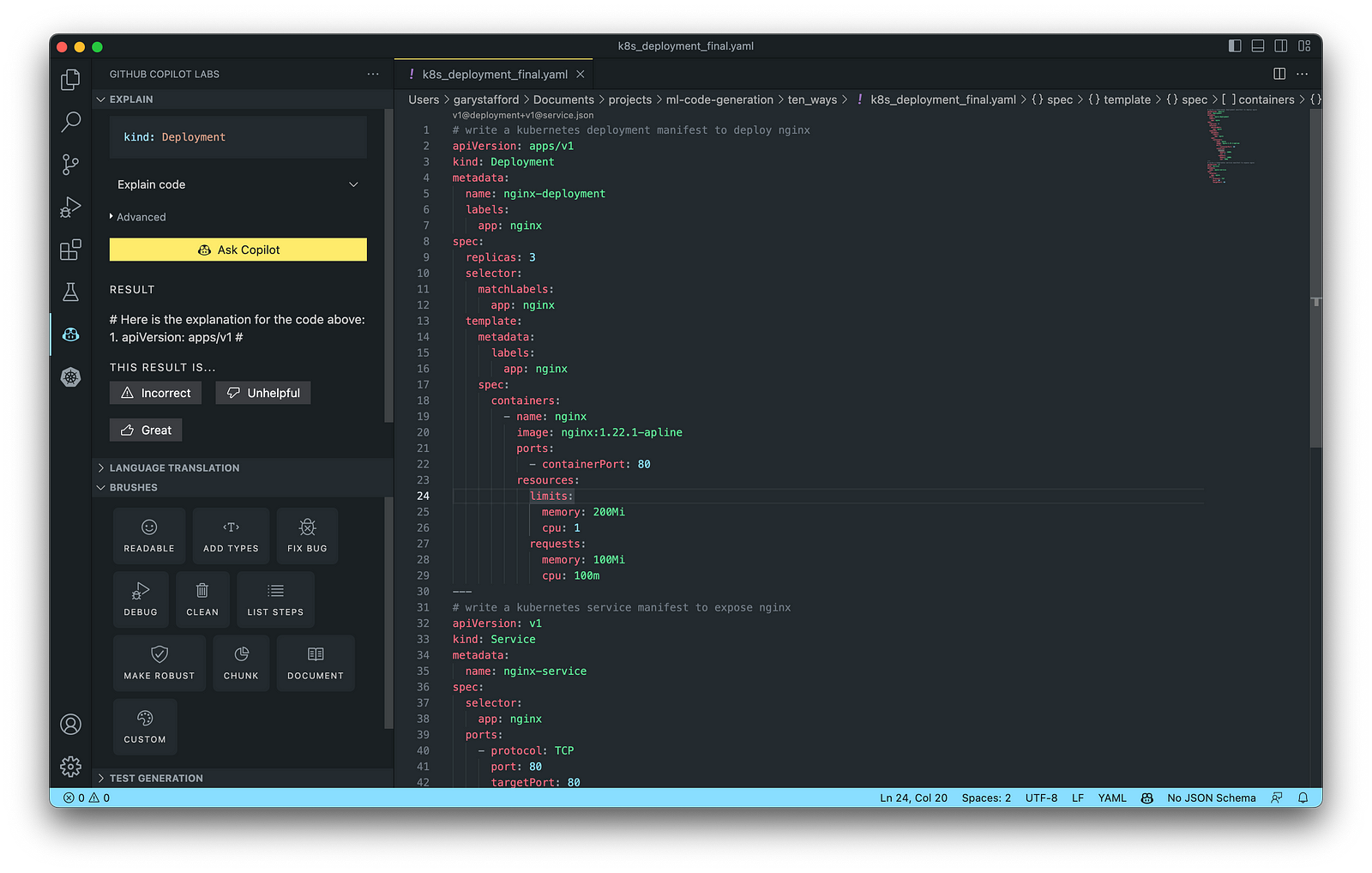
10. Utility Scripts
According to Bing AI — Search, utility scripts are small, simple snippets of code written as independent code files designed to perform a particular task. Utility scripts are commonly written in Bash, Shell, Python, Ruby, PowerShell, and PHP.
AWS utility scripts leverage the AWS Command Line Interface (AWS CLI) for Bash and Shell and AWS SDK for other programming languages. SDKs take the complexity out of coding by providing language-specific APIs for AWS services. For example, Boto3, AWS’s Python SDK, easily integrates your Python application, library, or script with AWS services, including Amazon S3, Amazon EC2, Amazon DynamoDB, and more.

An example of a Python script to calculate the total size of an Amazon S3 bucket, below, was inspired by 100daysofdevops/N-days-of-automation, a fantastic set of open source AWS-oriented automation scripts.

Conclusion
In this post, you learned ten ways to leverage Generative AI coding tools like GitHub Copilot for development on AWS. You saw how combining the latest generation of Generative AI coding tools, a mature and extensible IDE, and your coding experience will accelerate development, increase productivity, and reduce cost.
🔔 To keep up with future content, follow Gary Stafford on LinkedIn.
This blog represents my viewpoints and not those of my employer, Amazon Web Services (AWS). All product names, logos, and brands are the property of their respective owners.
Developing a Multi-Account AWS Environment Strategy
Posted by Gary A. Stafford in AWS, Cloud, DevOps, Enterprise Software Development, Technology Consulting on March 11, 2023
Explore twelve common patterns for developing an effective and efficient multi-account AWS environment strategy

Introduction
Every company is different: its organizational structure, the length of time it has existed, how fast it has grown, the industries it serves, its product and service diversity, public or private sector, and its geographic footprint. This uniqueness is reflected in how it organizes and manages its Cloud resources. Just as no two organizations are exactly alike, the structure of their AWS environments is rarely identical.
Some organizations successfully operate from a single AWS account, while others manage workloads spread across dozens or even hundreds of accounts. The volume and purpose of an organization’s AWS accounts are a result of multiple factors, including length of time spent on AWS, Cloud maturity, organizational structure and complexity, sectors, industries, and geographies served, product and service mix, compliance and regulatory requirements, and merger and acquisition activity.
“By design, all resources provisioned within an AWS account are logically isolated from resources provisioned in other AWS accounts, even within your own AWS Organizations.” (AWS)
Working with industry peers, the AWS community, and a wide variety of customers, one will observe common patterns for how organizations separate environments and workloads using AWS accounts. These patterns form an AWS multi-account strategy for operating securely and reliably in the Cloud at scale. The more planning an organization does in advance to develop a sound multi-account strategy, the less the burden that is required to manage changes as the organization grows over time.
The following post will explore twelve common patterns for effectively and efficiently organizing multiple AWS accounts. These patterns do not represent an either-or choice; they are designed to be purposefully combined to form a multi-account AWS environment strategy for your organization.
Patterns
- Pattern 1: Single “Uber” Account
- Pattern 2: Non-Prod/Prod Environments
- Pattern 3: Upper/Lower Environments
- Pattern 4: SDLC Environments
- Pattern 5: Major Workload Separation
- Pattern 6: Backup
- Pattern 7: Sandboxes
- Pattern 8: Centralized Management and Governance
- Pattern 9: Internal/External Environments
- Pattern 10: PCI DSS Workloads
- Pattern 11: Vendors and Contractors
- Pattern 12: Mergers and Acquisitions
Patterns 1–8 are progressively more mature multi-account strategies, while Patterns 9–12 represent special use cases for supplemental accounts.
Multi-Account Advantages
According to AWS’s whitepaper, Organizing Your AWS Environment Using Multiple Accounts, the benefits of using multiple AWS accounts include the following:
- Group workloads based on business purpose and ownership
- Apply distinct security controls by environment
- Constrain access to sensitive data (including compliance and regulation)
- Promote innovation and agility
- Limit the scope of impact from adverse events
- Support multiple IT operating models
- Manage costs (budgeting and cost attribution)
- Distribute AWS Service Quotas (fka limits) and API request rate limits
As we explore the patterns for organizing your AWS accounts, we will see how and to what degree each of these benefits is demonstrated by that particular pattern.
AWS Control Tower
Discussions about AWS multi-account environment strategies would not be complete without mentioning AWS Control Tower. According to the documentation, “AWS Control Tower offers a straightforward way to set up and govern an AWS multi-account environment, following prescriptive best practices.” AWS Control Tower includes Landing zone, described as “a well-architected, multi-account environment based on security and compliance best practices.”
AWS Control Tower is prescriptive in the Shared accounts it automatically creates within its AWS Organizations’ organizational units (OUs). Shared accounts created by AWS Control Tower include the Management, Log Archive, and Audit accounts. The previous standalone AWS service, AWS Landing Zone, maintained slightly different required accounts, including Shared Services, Log Archive, Security, and optional Network accounts. Although prescriptive, AWS Control Tower is also flexible and relatively unopinionated regarding the structure of Member accounts. Member accounts can be enrolled or unenrolled in AWS Control Tower.
You can decide whether or not to implement AWS Organizations or AWS Control Tower to set up and govern your AWS multi-account environment. Regardless, you will still need to determine how to reflect your organization’s unique structure and requirements in the purpose and quantity of the accounts you create within your AWS environment.
Common Multi-Account Patterns
While working with peers, community members, and a wide variety of customers, I regularly encounter the following twelve patterns for organizing AWS accounts. As noted earlier, these patterns do not represent an either-or choice; they are designed to be purposefully combined to form a multi-account AWS environment strategy for your organization.
Pattern 1: Single “Uber” Account
Organizations that effectively implement Pattern 1: Single “Uber” Account organize and separate environments and workloads at the sub-account level. They often use Amazon Virtual Private Cloud (Amazon VPC), an AWS account-level construct, to organize and separate environments and workloads. They may also use Subnets (VPC-level construct) or AWS Regions and Availability Zones to further organize and separate environments and workloads.
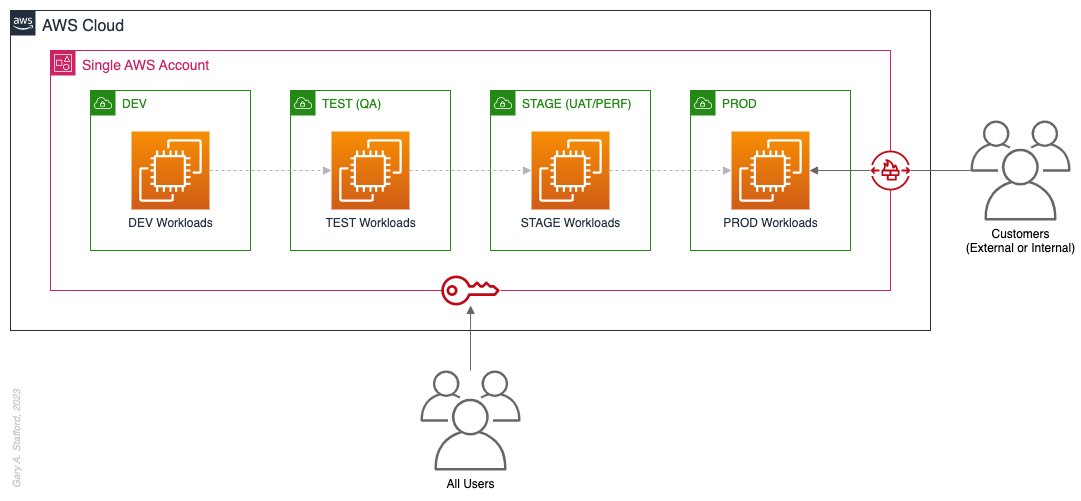
Pros
- Few, if any, significant advantages, especially for customer-facing workloads
Cons
- Decreased ability to limit the scope of impact from adverse events (widest blast radius)
- If the account is compromised, then all the organization’s workloads and data, possibly the entire organization, are potentially compromised (e.g., Ransomware attacks such as Encryptors, Lockers, and Doxware)
- Increased risk that networking or security misconfiguration could lead to unintended access to sensitive workloads and data
- Increased risk that networking, security, or resource management misconfiguration could lead to broad or unintended impairment of all workloads
- Decreased ability to perform team-, environment-, and workload-level budgeting and cost attribution
- Increased risk of resource depletion (soft and hard service quotas)
- Reduced ability to conduct audits and demonstrate compliance
Pattern 2: Non-Prod/Prod Environments
Organizations that effectively implement Pattern 2: Non-Prod/Prod Environments organize and separate non-Production workloads from Production (PROD) workloads using separate AWS accounts. Most often, they use Amazon VPCs within the non-Production account to separate workloads or Software Development Lifecycle (SDLC) environments, most often Development (DEV), Testing (TEST) or Quality Assurance (QA), and Staging (STAGE). Alternatively, these environments might also be designated as “N-1” (previous release), “N” (current release), “N+1” (next release), “N+2” (in development), and so forth, based on the currency of that version of that workload.
In some organizations, the Staging environment is used for User Acceptance Testing (UAT), performance (PERF) testing, and load testing before releasing workloads to Production. While in other organizations, STAGE, UAT, and PERF are each treated as separate environments at the account or VPC level.
Isolating Production workloads into their own account(s) and strictly limiting access to those workloads represents a significant first step in improving the overall maturity of your multi-account AWS environment strategy.

Pros
- Limits the scope of impact on Production as a result of adverse non-Production events (narrower blast radius)
- Logical separation and security of Production workloads and data
- Tightly control and limit access to Production, including the use of Break-the-Glass procedures (aka Break-glass or BTG); draws its name from “breaking the glass to pull a fire alarm”
- Eliminate the risk that non-Production networking or security misconfiguration could lead to unintended access to sensitive Production workloads and data
- Eliminate the chance that non-Production networking, security, or resource management misconfiguration could lead to broad or unintended impairment of Production workloads
- Conduct audits and demonstrate compliance with Production workloads
Cons
- If the Production account is compromised, then all the organization’s customer-facing workloads and data are potentially compromised
- Decreased ability to perform team-, environment-, and workload-level budgeting and cost attribution in the shared non-Production environment
- Increased risk of resource depletion (soft and hard service quotas) in the non-Production environment account
Pattern 3: Upper/Lower Environments
The next pattern, Pattern 3: Upper/Lower Environments, is a finer-grain variation of Pattern 2. With Pattern 3, we split all “Lower” environments into a single account and each “Upper” environment into its own account. In the software development process, initial environments, such as CI/CD for automated testing of code and infrastructure, Development, Test, UAT, and Performance, are called “Lower” environments. Conversely, later environments, such as Staging, Production, and even Disaster Recovery (DR), are called “Upper” environments. Upper environments typically require isolation for stability during testing or security for Production workloads and sensitive data.
Often, courser-grain patterns like Patterns 1–3 are carryovers from more traditional on-premises data centers, where compute, storage, network, and security resources were more constrained. Although these patterns can be successfully reproduced in the Cloud, they may not be optimal compared to more “cloud native” patterns, which provide improved separation of concerns.

Pros
- Limits the scope of impact on individual Upper environments as a result of adverse Lower environment events (narrower blast radius)
- Increased stability of Staging environment for critical UAT, performance, and load testing
- Logical separation and security of Production workloads and data
- Tightly control and limit access to Production, including the use of BTG
- Eliminate the risk that non-Production networking or security misconfiguration could lead to unintended access to sensitive Production workloads and data
- Eliminate the chance that non-Production networking, security, or resource management misconfiguration could lead to broad or unintended impairment of Production workloads
- Conduct audits and demonstrate compliance with Production workloads
Cons
- If the Production account is compromised, then all the organization’s customer-facing workloads and data are potentially compromised (e.g., Ransomware attack)
- Decreased ability to perform team-, environment-, and workload-level budgeting and cost attribution in the shared Lower environment
- Increased risk of resource depletion (soft and hard service quotas) in the Lower environment account
Pattern 4: SDLC Environments
The next pattern, Pattern 4: SDLC Environments, is a finer-grain variation of Pattern 3. With Pattern 4, we gain complete separation of each SDLC environment into its own AWS account. Using AWS services like AWS IAM Identity Center (fka AWS SSO), the Security team can enforce least-privilege permissions at an AWS Account level to individual groups of users, such as Developers, Testers, UAT, and Performance testers.
Based on my experience, Pattern 4 represents the minimal level of workload separation an organization should consider when developing its multi-account AWS environment strategy. Although Pattern 4 has a number of disadvantages, when combined with subsequent patterns and AWS best practices, this pattern begins to provide a scalable foundation for an organization’s growing workload portfolio.

Patterns, such as Pattern 4, not only apply to traditional software applications and services. These patterns can be applied to data analytics, AI/ML, IoT, media services, and similar workloads where separation of environments is required.

Pros
- Limits the scope of impact on one SDLC environment as a result of adverse events in another environment (narrower blast radius)
- Logical separation and security of Production workloads and data
- Increased stability of each SDLC environment
- Tightly control and limit access to Production, including the use of BTG
- Reduced risk that non-Production networking or security misconfiguration could lead to unintended access to sensitive Production workloads and data
- Reduced risk that non-Production networking, security, or resource management misconfiguration could lead to broad or unintended impairment of Production workloads
- Conduct audits and demonstrate compliance with Production workloads
- Increased ability to perform budgeting and cost attribution for each SDLC environment
- Reduced risk of resource depletion (soft and hard service limits) and IP conflicts and exhaustion within any single SDLC environment
Cons
- All workloads for each SDLC environment run within a single account, including Production, increasing the potential scope of impact from adverse events within that environment’s account
- If the Production account is compromised, then all the organization’s customer-facing workloads and data are potentially compromised
- Decreased ability to perform workload-level budgeting and cost attribution
Pattern 5: Major Workload Separation
The next pattern, Pattern 5: Major Workload Separation, is a finer-grain variation of Pattern 4. With Pattern 5, we separate each significant workload into its own separate SDLC environment account. The security team can enforce fine-grain least-privilege permissions at an AWS Account level to individual groups of users, such as Developers, Testers, UAT, and Performance testers, by their designated workload(s).
Pattern 5 has several advantages over the previous patterns. In addition to the increased workload-level security and reliability benefits, Pattern 5 can be particularly useful for organizations that operate significantly different technology stacks and specialized workloads, particularly at scale. Different technology stacks and specialized workloads often each have their own unique development, testing, deployment, and support processes. Isolating these types of workloads will help facilitate the support of multiple IT operating models.

Pros
- Limits the scope of impact on an individual workload as a result of adverse events from another workload or SDLC environment (narrowest blast radius)
- Increased ability to perform team-, environment-, and workload-level budgeting and cost attribution
Cons
- If the Production account is compromised, then all the organization’s customer-facing workloads and data are potentially compromised (e.g., Ransomware attack)
Pattern 6: Backup
In the earlier patterns, we mentioned that if the Production account were compromised, all the organization’s customer-facing workloads and data could be compromised. According to TechTarget, 2022 was a breakout year for Ransomware attacks. According to the US government’s CISA.gov website, “Ransomware is a form of malware designed to encrypt files on a device, rendering any files and the systems that rely on them unusable. Malicious actors then demand ransom in exchange for decryption.”
According to AWS best practices, one of the recommended preparatory actions to protect and recover from Ransomware attacks is backing up data to an alternate account using tools such as AWS Backup and an AWS Backup vault. Solutions such as AWS Backup protect and restore data regardless of how it was made inaccessible.
In Pattern 6: Backup, we create one or more Backup accounts to protect against unintended data loss or account compromise. In the example below, we have two Backup accounts, one for Production data and one for all non-Production data.

Pros
- If the Production account is compromised (e.g., Ransomware attacks such as Encryptors, Lockers, and Doxware), there are secure backups of data stored in a separate account, which can be used to restore or recreate the Production environment
Cons
- Few, if any, significant disadvantages when combined with previous patterns and AWS best-practices
Pattern 7: Sandboxes
The following pattern, Pattern 7: Sandboxes, supplements the previous patterns, designed to address the needs of an organization to allow individual users and teams to learn, build, experiment, and innovate on AWS without impacting the larger organization’s AWS environment. To quote the AWS blog, Best practices for creating and managing sandbox accounts in AWS, “Many organizations need another type of environment, one where users can build and innovate with AWS services that might not be permitted in production or development/test environments because controls have not yet been implemented.” Further, according to TechTarget, “a Sandbox is an isolated testing environment that enables users to run programs or open files without affecting the application, system, or platform on which they run.”
Due to the potential volume of individual user and team accounts, sometimes referred to as Sandbox accounts, mature infrastructure automation practices, cost controls, and self-service provisioning and de-provisioning of Sandbox accounts are critical capabilities for the organization.

Pros
- Allow individual users and teams to learn, build, experiment, and innovate on AWS without impacting the rest of the organization’s AWS environment
Cons
- Without mature automation practices, cost controls, and self-service capabilities, managing multiple individual and team Sandbox accounts can become unwieldy and costly
Pattern 8: Centralized Management and Governance
We discussed AWS Control Tower at the beginning of this post. AWS Control Tower is prescriptive in creating Shared accounts within its AWS Organizations’ organizational units (OUs), including the Management, Log Archive, and Audit accounts. AWS encourages using AWS Control Tower to orchestrate multiple AWS accounts and services on your behalf while maintaining your organization’s security and compliance needs.
As exemplified in Pattern 8: Centralized Management and Governance, many organizations will implement centralized management whether or not they decide to implement AWS Control Tower. In addition to the Management account (payer account, fka master account), organizations often create centralized logging accounts, and centralized tooling (aka Shared services) accounts for functions such as CI/CD, IaC provisioning, and deployment. Another common centralized management account is a Security account. Organizations use this account to centralize the monitoring, analysis, notification, and automated mitigation of potential security issues within their AWS environment. The Security accounts will include services such as Amazon Detective, Amazon Inspector, Amazon GuardDuty, and AWS Security Hub.

Pros
- Increased ability to manage and maintain multiple AWS accounts with fewer resources
- Reduced duplication of management resources across accounts
- Increased ability to use automation and improve the consistency of processes and procedures across multiple accounts
Cons
- Few, if any, significant disadvantages when combined with previous patterns and AWS best-practices
Pattern 9: Internal/External Environments
The next pattern, Pattern 9: Internal/External Environments, focuses on organizations with internal operational systems (aka Enterprise systems) in the Cloud and customer-facing workloads. Pattern 9 separates internal operational systems, platforms, and workloads from external customer-facing workloads. For example, an organization’s divisions and departments, such as Sales and Marketing, Finance, Human Resources, and Manufacturing, are assigned their own AWS account(s). Pattern 9 allows the Security team to ensure that internal departmental or divisional users are isolated from users who are responsible for developing, testing, deploying, and managing customer-facing workloads.
Note that the diagram for Pattern 9 shows remote users who access AWS End User Computing (EUC) services or Virtual Desktop Infrastructure (VDI), such as Amazon WorkSpaces and Amazon AppStream 2.0. In this example, remote workers have secure access to EUC services provisioned in a separate AWS account, and indirectly, internal systems, platforms, and workloads.

Pros
- Separation of internal operational systems, platforms, and workloads from external customer-facing workloads
Cons
- Few, if any, significant disadvantages when combined with previous patterns and AWS best-practices
Pattern 10: PCI DSS Workloads
The next pattern, Pattern 10: PCI DSS Workloads, is a variation of previous patterns, which assumes the existence of Payment Card Industry Data Security Standard (PCI DSS) workloads and data. According to the AWS, “PCI DSS applies to entities that store, process, or transmit cardholder data (CHD) or sensitive authentication data (SAD), including merchants, processors, acquirers, issuers, and service providers. The PCI DSS is mandated by the card brands and administered by the Payment Card Industry Security Standards Council.”
According to AWS’s whitepaper, Architecting for PCI DSS Scoping and Segmentation on AWS, “By design, all resources provisioned within an AWS account are logically isolated from resources provisioned in other AWS accounts, even within your own AWS Organizations. Using an isolated account for PCI workloads is a core best practice when designing your PCI application to run on AWS.” With Pattern 10, we separate non-PCI DSS and PCI DSS Production workloads and data. The assumption is that only Production contains PCI DSS data. Data in lower environments is synthetically generated or sufficiently encrypted, masked, obfuscated, or tokenized.
Note that the diagram for Pattern 10 shows Administrators. Administrators with different spans of responsibility and access are present in every pattern, whether specifically shown or not.

Pros
- Increased ability to meet compliance requirements by separating non-PCI DSS and PCI DSS Production workloads
Cons
- Few, if any, significant disadvantages when combined with previous patterns and AWS best-practices
Pattern 11: Vendors and Contractors
The next pattern, Pattern 11: Vendors and Contractors, is focused on organizations that employ contractors or use third-party vendors who provide products and services that interact with their AWS-based environment. Like Pattern 10, Pattern 11 allows the Security team to ensure that contractor and vendor-based systems’ access to internal systems and customer-facing workloads is tightly controlled and auditable.
Vendor-based products and services are often deployed within an organization’s AWS environment without external means of ingress or egress. Alternatively, a vendor’s product or service may have a secure means of ingress from or egress to external endpoints. Such is the case with some SaaS products, which ship an organization’s data to an external aggregator for analytics or a security vendor’s product that pre-filters incoming data, external to the organization’s AWS environment. Using separate AWS accounts can improve an organization’s security posture and mitigate the risk of adverse events on the organization’s overall AWS environment.

Pros
- Ensure access to internal systems and customer-facing workloads by contractors and vendor-based systems is tightly controlled and auditable
Cons
- Few, if any, significant disadvantages when combined with previous patterns and AWS best-practices
Pattern 12: Mergers and Acquisitions
The next pattern, Pattern 12: Mergers and Acquisitions, is focused on managing the integration of external AWS accounts as a result of a merger or acquisition. This is a common occurrence, but the exact details of how best to handle the integration of two or more integrations depend on several factors. Factors include the required level of integration, for example, maintaining separate AWS Organizations, maintaining different AWS accounts, or merging resources from multiple accounts. Other factors that might impact account structure include changes in ownership or payer of acquired accounts, existing acquired cost-savings agreements (e.g., EDPs, PPAs, RIs, and Savings Plans), and AWS Marketplace vendor agreements. Even existing authentication and authorization methods of the acquiree versus the acquirer (e.g., AWS IAM Identity Center, Microsoft Active Directory (AD), Azure AD, and external identity providers (IdP) like Okta or Auth0).
The diagram for Pattern 12 attempts to show a few different M&A account scenarios, including maintaining separate AWS accounts for the acquirer and acquiree (e.g., acquiree’s Manufacturing Division account). If desired, the accounts can be kept independent but managed within the acquirer’s AWS Organizations’ organization. The diagram also exhibits merging resources from the acquiree’s accounts into the acquirer’s accounts (e.g., Sales and Marketing accounts). Resources will be migrated or decommissioned, and the account will be closed.

Pros
- Maintain separation between an acquirer and an acquiree’s AWS accounts within a single organization’s AWS environment
- Potentially consolidate and maximize cost-saving advantages of volume-related financial agreements and vendor licensing
Cons
- Migrating workloads between different organizations, depending on their complexity, requires careful planning and testing
- Consolidating multiple authentication and authorization methods requires careful planning and testing to avoid improper privileges
- Consolidating and optimizing separate licensing and cost-saving agreements between multiple organizations requires careful planning and an in-depth understanding of those agreements
Multi-Account AWS Environment Example
You can form an efficient and effective multi-account strategy for your organization by purposefully combining multiple patterns. Below is an example of combining the features of several patterns: Major Workload Separation, Backup, Sandboxes, Centralized Management and Governance, Internal/External Environments, and Vendors and Contractors.
According to AWS, “You can use AWS Organizations’ organizational units (OUs) to group accounts together to administer as a single unit. This greatly simplifies the management of your accounts.” If you decide to use AWS Organizations, each set of accounts associated with a pattern could correspond to an OU: Major Workload A, Major Workload B, Sandboxes, Backups, Centralized Management, Internal Environments, Vendors, and Contractors.

Conclusion
This post taught us twelve common patterns for effectively and efficiently organizing your AWS accounts. Instead of an either-or choice, these patterns are designed to be purposefully combined to form a multi-account strategy for your organization. Having a sound multi-account strategy will improve your security posture, maintain compliance, decrease the impact of adverse events on your AWS environment, and improve your organization’s ability to safely and confidently innovate and experiment on AWS.
Recommended References
- Establishing Your Cloud Foundation on AWS (AWS Whitepaper)
- Organizing Your AWS Environment Using Multiple Accounts (AWS Whitepaper)
- Building a Cloud Operating Model (AWS Whitepaper)
- AWS Security Reference Architecture (AWS Prescriptive Guidance)
- AWS Security Maturity Model (AWS Whitepaper)
- AWS re:Invent 2022 — Best practices for organizing and operating on AWS (YouTube Video)
- AWS Break Glass Role (GitHub)
🔔 To keep up with future content, follow Gary Stafford on LinkedIn.
This blog represents my viewpoints and not those of my employer, Amazon Web Services (AWS). All product names, logos, and brands are the property of their respective owners.
Brief Introduction to Observability on AWS
Posted by Gary A. Stafford in AWS, Cloud, DevOps on March 9, 2023
Explore the wide variety of Application Performance Monitoring (APM) and Observability options on AWS

APM and Observability
Observability is “the extent to which the internal states of a system can be inferred from externally available data” (Gartner). The three pillars of observability data are metrics, logs, and traces. Application Performance Monitoring (APM), a term commonly associated with observability, is “software that enables the observation and analysis of application health, performance, and user experience” (Gartner).
Additional features often associated with APM and observability products and services include the following (in alphabetical order):
- Advanced Threat Protection (ATP)
- Endpoint Detection and Response (EDR)
- Incident Detection Response (IDR)
- Infrastructure Performance Monitoring (IPM)
- Network Device Monitoring (NDM)
- Network Performance Monitoring (NPM)
- OpenTelemetry (OTel)
- Operational (or Operations) Intelligence Platform
- Predictive Monitoring (predictive analytics / predictive modeling)
- Real User Monitoring (RUM)
- Security Information and Event Management (SIEM)
- Security Orchestration, Automation, and Response (SOAR)
- Synthetic Monitoring (directed monitoring / synthetic testing)
- Threat Visibility and Risk Scoring
- Unified Security and Observability Platform
- User Behavior Analytics (UBA)
Not all features are offered by all vendors. Most vendors tend to specialize in one or more areas. Determining which features are essential to your organization before choosing a solution is vital.
AI/ML
Given the growing volume and real-time nature of observability telemetry, many vendors have started incorporating AI and ML into their products and services to improve correlation, anomaly detection, and mitigation capabilities. Understand how these features can reduce operational burden, enhance insights, and simplify complexity.
Decision Factors
APM and observability tooling choices often come down to a “Build vs. Buy” decision for organizations. In the Cloud, this usually means integrating several individual purpose-built products and services, self-managed open-source projects, or investing in an end-to-end APM or unified observability platform. Other decision factors include the need for solutions to support:
- Hybrid cloud environments (on-premises/Cloud)
- Multi-cloud environments (Public Cloud, SaaS, Supercloud)
- Specialized workloads (e.g., Mainframes, HPC, VMware, SAP, SAS)
- Compliant workloads (e.g., PCI DSS, PII, GDPR, FedRAMP)
- Edge Computing and IoT/IIoT
- AI, ML, and Data Analytics monitoring (AIOps, MLOps, DataOps)
- SaaS observability (SaaS providers who offer monitoring to their end-users as part of their service offering)
- Custom log formats and protocols
Finally, the 5 V’s of big data: Velocity, Volume, Value, Variety, and Veracity, also influence the choice of APM and observability tooling. The real-time nature of the observability data, the sheer volume of the data, the source and type of data, and the sensitivity of the data, will all guide tooling choices based on features and cost.
Organizations can choose fully-managed native AWS services, AWS Partner products and services, often SaaS, self-managed open-source observability tooling, or a combination of options. Many AWS and Partner products and services are commercial versions of popular open-source software (COSS).
AWS Options
- Amazon CloudWatch: Observe and monitor resources and applications you run on AWS in real time. CloudWatch features include Amazon CloudWatch Container, Lambda, Contributor, and Application Insights.
- Amazon Managed Service for Prometheus (AMP): Prometheus-compatible service that monitors and provides alerts on containerized applications and infrastructure at scale. Prometheus is “an open-source systems monitoring and alerting toolkit originally built at SoundCloud.”
- Amazon Managed Grafana (AMG): Commonly paired with AMP, AMG is a fully managed service for Grafana, an open-source analytics platform that “enables you to query, visualize, alert on, and explore your metrics, logs, and traces wherever they are stored.”
- Amazon OpenSearch Service: Based on open-source OpenSearch, this service provides interactive log analytics and real-time application monitoring and includes OpenSearch Dashboards (comparable to Kibana). Recently announced new security analytics features provide threat monitoring, detection, and alerting capabilities.
- AWS X-Ray: Trace user requests through your application, viewed using the X-Ray service map console or integrated with CloudWatch using the CloudWatch console’s X-Ray Traces Service map.
Data Collection, Processing, and Forwarding
- AWS Distro for OpenTelemetry (ADOT): Open-source APIs, libraries, and agents to collect distributed traces and metrics for application monitoring. ADOT is an open-source distribution of OpenTelemetry, a “high-quality, ubiquitous, and portable telemetry [solution] to enable effective observability.”
- AWS for Fluent Bit: Fluent Bit image with plugins for both CloudWatch Logs and Kinesis Data Firehose. Fluent Bit is an open-source, “super fast, lightweight, and highly scalable logging and metrics processor and forwarder.”
Security-focused Monitoring
- AWS CloudTrail: Helps enable operational and risk auditing, governance, and compliance in your AWS environment. CloudTrail records events, including actions taken in the AWS Management Console, AWS Command Line Interface (CLI), AWS SDKs, and APIs.
Partner Options
According to the 2022 Gartner® Magic Quadrant™ — APM & Observability Report, leading vendors commonly used by AWS customers include the following (in alphabetical order):
- AppDynamics (Cisco)
- Datadog
- Dynatrace
- Elastic
- Honeycomb
- Instanta (IBM)
- logz.io
- New Relic
- Splunk
- Sumo Logic
Open Source Options
There are countless open-source observability projects to choose from, including the following (in alphabetical order):
- Elastic: Elastic Stack: Elasticsearch, Kibana, Beats, and Logstash
- Fluentd: Data collector for unified logging layer
- Grafana: Platform for monitoring and observability
- Jaeger: End-to-end distributed tracing
- Kiali: Configure, visualize, validate, and troubleshoot Istio Service Mesh
- Loki: Like Prometheus, but for logs
- OpenSearch: Scalable, flexible, and extensible software suite for search, analytics, and observability applications
- OpenTelemetry (OTel): Collection of tools, APIs, and SDKs used to instrument, generate, collect, and export telemetry data
- Prometheus: Monitoring system and time series database
- Zabbix: Single pane of glass view of your whole IT infrastructure stack
🔔 To keep up with future content, follow Gary Stafford on LinkedIn.
This blog represents my viewpoints and not those of my employer, Amazon Web Services (AWS). All product names, logos, and brands are the property of their respective owners.
Architecting a Successful SaaS: Interacting with your Customer’s Cloud Accounts
Posted by Gary A. Stafford in AWS, Cloud, Technology Consulting on June 2, 2020
Originally published on the AWS APN Blog.
Introduction
You’re a software vendor selling a SaaS product hosted on the cloud. As part of your solution, the SaaS product needs to interact with your customer’s cloud-based resources—their data, their applications, or perhaps their compute resources. As a vendor, you must architect your SaaS product, and its capability to interact with your customer’s cloud-based resources to be scalable, reliable, performant, cost optimized, and most importantly, secure. In this post, we will explore several common AWS services and architectural patterns used by SaaS vendors to interact with their customer’s AWS accounts. Although multi-cloud and hybrid cloud SaaS interactions are also prevalent in many SaaS solutions, for brevity we will limit ourselves to interactions across accounts within AWS.
Reasons for Interaction
There are many reasons SaaS products need to interact with a customer’s AWS Accounts. Examples of SaaS products that require some level of account interaction often fall into the categories of logging and monitoring, security, compliance, data analytics, DevOps, workflow management, and resource optimization. SaaS products, such as the ones in these categories, regularly interact with resources in the subscribing customer’s AWS Account. Examples of interactions include the following.
- Data Analytics: A product that connects to private data sources within their customer’s cloud accounts;
- Logging and Monitoring: A product that continuously aggregates, analyzes and visualizes logs from their customer’s cloud accounts;
- Security: A product that uses ML and AI to continuously scan their customer’s cloud accounts for suspicious activities;
- Workflow Management: A product that remotely manages resources in their customer’s cloud account to achieve cost optimization;
- Security: A product that intercepts and inspects traffic, in real-time, to and from their customer’s cloud-based applications, detecting fraud and other malicious activities;
- DevOps: A product that creates resources and deploys assets to their customer’s cloud accounts;
- Compliance: A product that continuously audits their customer’s cloud account activities for regulatory compliance and corporate governance violations;
Scaling Interactions
As individuals and businesses, we often interact and share resources between our own AWS Accounts. We can delegate access across our accounts using AWS Single Sign-On (SSO) or IAM Roles, referred to as cross-account access. A typical example is allowing a Developer, an IAM User in the Development account, limited access to the Production account, to troubleshoot a customer issue. We can implement cross-account VPC Sharing within our AWS Organization or VPC Peering to route traffic, privately, between two or more accounts. These methods are used when applications, deployed to different AWS Accounts, such as accounting and shipping, need to communicate with each other.
Below, we see a typical example of an Enterprise AWS account structure, modeled around the Software Development Life Cycle (SDLC) and using good DevOps practices.
Cloud services and architectural patterns that work for an individual customer of the cloud, may not scale for a SaaS vendor. Implementing a scalable and reliable architecture to interact with tens, hundreds, or thousands of a SaaS vendor’s customer’s AWS Accounts, can be vastly more complex and challenging than across a single organization’s accounts, even a large organization. Similarly, losing or exposing data can be devastating to an organization. Losing or exposing hundreds or thousands of SaaS customer’s data and potentially their own customer’s data is likely fatal to a SaaS vendor’s reputation and their business.
Below, we see a typical example of a SaaS vendor’s AWS account structure, modeled around a multi-tier pricing and support strategy. Free Trial and Business customers are pooled together using a multi-tenant architecture, while enterprise customers are isolated using a single-tenant architecture.
Tenancy Model
The tenant isolation strategy of a SaaS vendor can have a direct impact on the methods the vendor implements to integrate with their customer’s accounts. For those unfamiliar with the terms Tenancy Model or Tenant Isolation, think housing. A tenant (a SaaS vendor’s customer) might reside in a single-family house, referred to as a single-tenant architecture. The tenant is isolated or siloed from their neighbors (other SaaS customers). Alternately, a tenant might reside in an apartment building, living adjacent to other tenants, referred to as multi-tenant architecture. Tenants (customers) share the same structure with limited isolation compared to single-tenant architecture. Multiple customer resources are pooled. To learn more about tenancy, I recommend the presentation, AWS re:Invent 2019: SaaS tenant isolation patterns, available on YouTube, by Tod Golding of AWS.
An application’s tenancy model is seldom binary. The degree to which SaaS customer’s resources are isolated or conversely, pooled, varies based on a vendor’s architecture and is influenced by the underlying technologies used by the vendor. Different tiers of the application—front-end, back-end, data—may differ in their degree and means of tenant isolation. For example, on AWS, isolation may be achieved at different levels of the network. A SaaS vendor may assign each customer to their own AWS Account, providing a very high level of isolation. Alternatively, a vendor might choose to use a single account and separate customers within their own VPC. Customers may be housed within the same VPC, but separated by compute instance or other software constructs.
Additionally, many SaaS vendors have more than one tenancy model. Frequently, a SaaS vendor will offer a multi-tier pricing structure. For example, a Business Tier, which is based on a shared, multi-tenant architecture, and an Enterprise Tier, which is based on an isolated, single-tenant architecture. Vendors may also offer a free tier or trial offer, which is almost always multi-tenant.
The cloud services and architectural patterns used by vendors to integrate with customer accounts, each have their own advantages and disadvantages. Some AWS services and architectural patterns may be inherently better at scaling to meet a SaaS vendor’s large growing customer base. They are well-suited for multi-tenant architecture. Other services and architectural patterns may provide a high level of interaction but lack scalability. They may be better suited for a single-tenant architecture.
AWS Services
There are several AWS services capable of enabling integrations between a vendor’s SaaS product and their customer’s resources, within their AWS Accounts. Some AWS services were even designed with SaaS in mind, including AWS PrivateLink and Amazon EventBridge. Services are often combined in common architectural patterns. Here are eight examples of AWS services capable of enabling integrations between a vendor’s SaaS product and their customer’s resources, within their AWS Accounts.
Cross-Account IAM Roles
According to AWS, customers can use an IAM Role to delegate access to resources that are in different AWS accounts, often referred to as a cross-account role. With IAM roles, you can establish trust relationships between your trusting account and other AWS trusted accounts. By setting up cross-account access in this way, you do not need to create individual IAM users in each account. With IAM roles, you can establish trust relationships between your trusting account and other AWS trusted accounts.
External IDs
Using External IDs are a good way to improve the security of cross-account role handling in a SaaS solution, and should be used by vendors who are implementing a SaaS product that uses cross-account roles. Choosing this option restricts access to the role only through the AWS CLI, Tools for Windows PowerShell, or the AWS API.
Using cross-account roles with external IDs, a SaaS customer allows their SaaS vendor programmatic access to their AWS account. The cross-account role’s policies tightly control the permissions of the vendor (trusted account) and limit access within the customer’s account (trusting account). Vendors use cross-account access for many activities, including resource optimization, DevOps functions, patching and upgrades, and managing their agents.
AWS Transfer for SFTP
According to AWS, AWS Transfer for SFTP is a fully managed service that enables the transfer of files directly into and out of Amazon S3 using the Secure File Transfer Protocol (SFTP)—also known as Secure Shell (SSH) File Transfer Protocol. Announced in November 2018, AWS SFTP is fully compatible with the SFTP standard, connects to your Active Directory, LDAP, and other identity systems, and works with Route 53 DNS routing.
Using S3 to transfer objects (files) between a SaaS vendor’s account and their customer’s account is very common. There are multiple AWS services, including AWS Transfer for SFTP, which can be used to interact with a customer’s account using S3. A vendor may choose to pull from or push to the customer’s S3 bucket using one of these services. Alternately, a customer might push to or pull from a vendor’s S3 bucket, as shown below.
To use AWS Transfer for SFTP, you set up users and assign them each an IAM role. This role determines the level of access that they have to your S3 bucket. When you create an SFTP user, you make several decisions about user access. These include which Amazon S3 buckets the user can access, what portions of each S3 bucket are accessible, and what privileges the user has, for example, PUT
or GET
.
Below, we see an example of the SaaS vendor exposing a secure FTP site, backed by S3. The customers use SFTP to exchange data between their account and their SaaS application. File transfer may be managed by the customer or by a vendor agent, deployed into the customer’s account.
In late April 2020, AWS announced AWS Transfer Family, which is the aggregated name of AWS Transfer for SFTP, FTPS, and FTP. In addition to Secure File Transfer Protocol (SFTP), announce the expansion of the service to add support for File Transfer Protocol over SSL (FTPS) and File Transfer Protocol (FTP).
Amazon S3 Access Points
According to AWS, Amazon S3 Access Points, a feature of S3, simplifies managing data access at scale for applications using shared data sets on S3. Announced in December 2019, Access points are unique hostnames that customers create to enforce distinct permissions and network controls for any request made through the access point. Amazon S3 Access Points can easily scale access for hundreds of applications by creating individualized access points with names and permissions customized for each application.
Access Points support AWS Identity and Access Management (IAM) resource policies that allow you to control the use of the access point by resource, user, or other conditions. For an application or user to be able to access objects through an access point, both the access point and the underlying bucket must permit the request. Using an Access Point Policy, you can enable another account to access objects within an S3 bucket. The access point policy gives you fine-grain control over which users and which permissions, such as to GET
and PUT
objects, are allowed.
Below, we see an example of the SaaS vendor exposing an S3 Access Point. The customers use the supplied access points to exchange data between their account and their SaaS application. The interchange of data may be managed by the customer or by a vendor agent, deployed into the customer’s account.
Amazon API Gateway
According to AWS, Amazon API Gateway is a fully managed service that makes it easy for developers to create, publish, maintain, monitor, and secure APIs at any scale. APIs act as the front door for applications to access data, business logic, or functionality from backend services. Using API Gateway, you can create RESTful APIs and WebSocket APIs that enable real-time two-way communication applications. API Gateway supports containerized and serverless workloads, as well as web applications.
Announced in July 2015, Amazon API Gateway and the accompanying APIs serve as a scalable, reliable, performant, and secure connections to backend resources in either the customer or SaaS vendor’s account. API Gateway backend resources include Lambda functions, HTTP endpoints, VPC Links, and other AWS services. Those AWS services include Amazon SQS and Kinesis. These services are often used to exchange data between a SaaS vendor’s account and their customer’s accounts. Eric Johnson of AWS gave a great presentation on API Gateway, available on YouTube, AWS re:Invent 2019: I didn’t know Amazon API Gateway did that.
Several security features can be used to secure communications between the vendor and the customer accounts when using the API Gateway. Security features include encrypting transmitted data using SSL/TLS Certificates (HTTPS), AWS WAF (Web Application Firewall) integration, Client Certificates to ensure HTTP requests to your back-end services are originating from the API Gateway, Authorization using Amazon Cognito User Pools or a Lambda function, API Keys, and VPC Links.
Below, we see an example of the SaaS vendor exposing an API, backed by an SQS queue. The customers use the API to programmatically exchange data between their account and their SaaS application. The interchange of data may be managed by the customer or by a vendor agent, deployed into the customer’s account.
VPC Peering
According to AWS, Amazon Virtual Private Cloud (Amazon VPC) enables customers to launch AWS resources into a virtual network that they have defined. A VPC peering connection is a networking connection between two VPCs that allows you to route traffic between them using private IPv4 addresses or IPv6 addresses. You can create a VPC peering connection between your VPCs, or with a VPC in another AWS account. As announced in November 2018, VPCs can be in different regions (known as inter-region VPC peering).
Below, we see an example of the SaaS vendor and the customer using VPC peering to enable direct interaction between a customer’s application, within their account, and their SaaS application, in the vendor’s account.
One of the limitations of VPC peering, which might impact its ability to scale for SaaS vendors using multi-tenant architectures, is overlapping CIDR blocks. According to AWS, you cannot create a VPC peering connection between VPCs with matching or overlapping IPv4 CIDR blocks. Given tens or hundreds of customers, VPC peering presents challenges in managing individual CIDR blocks when using a multi-tenant SaaS architectural approach.
VPC Endpoints
According to AWS, a VPC endpoint enables you to privately connect your VPC to supported AWS services and VPC endpoint services powered by AWS PrivateLink without requiring an Internet Gateway, NAT device, VPN connection, or AWS Direct Connect connection. Instances in your VPC do not require public IP addresses to communicate with resources in the service. Traffic between your VPC and the other service does not leave the Amazon network.
There are two types of VPC endpoints. An interface endpoint, Powered by AWS PrivateLink, is an elastic network interface (ENI) with a private IP address from the IP address range of your subnet that serves as an entry point for traffic destined to a supported service. Interface endpoints support a large and growing list of AWS services. The second type of VPC endpoint, a gateway endpoint, is a gateway that you specify as a target for a route in your route table for traffic destined to a supported AWS service. Both Amazon S3 and Amazon DynamoDB are currently supported by gateway endpoints.
AWS PrivateLink
According to AWS, AWS PrivateLink simplifies the security of data shared with cloud-based applications by eliminating the exposure of data to the public Internet. AWS PrivateLink provides private connectivity between VPCs, AWS services, and on-premises applications, securely on the AWS network. AWS PrivateLink makes it easy to connect services across different accounts and VPCs to simplify the network architecture significantly. James Devine of AWS delivered a great presentation on PrivateLink, available on YouTube, AWS re:Invent 2018: Best Practices for AWS PrivateLink.
AWS Service Endpoints
AWS Service Endpoints will direct the traffic to AWS services over AWS PrivateLink while keeping the network traffic within the AWS network. AWS PrivateLink enables SaaS providers to offer services that will look and feel like they are hosted directly on a private network. These services are securely accessible both from the cloud and from on-premises via AWS Direct Connect and AWS VPN, in a highly available and scalable manner.
Below, we see an example of routing API calls from the customer’s account to the SaaS vendor’s account, using an interface VPC endpoint (interface endpoint) powered by AWS PrivateLink. The customer’s requests are sent through ENIs in each private subnet to a Network Load Balancer (NLB) within the vendor’s account, using PrivateLink. There is no need for an Internet Gateway to route traffic over the public Internet.
Below, we see an example of near real-time routing messages from an application within the customer’s account to an Amazon Kinesis Data Firehose delivery stream in the SaaS vendor’s account, using an interface endpoint powered by AWS PrivateLink. Again, there is no need for an Internet Gateway to route traffic over the public Internet. The interchange of data may be managed by the customer or by a vendor agent, deployed into the customer’s account.
Amazon EventBridge
According to AWS, Amazon EventBridge is a serverless event bus that makes it easy to connect applications using data from your applications, integrated SaaS applications, and AWS services. EventBridge delivers a stream of real-time data from event sources and routes that data to targets like AWS Lambda. You can set up routing rules to determine where to send your data to build application architectures that react in real-time to all of your data sources. EventBridge makes it easy to build event-driven applications because it takes care of event ingestion and delivery, security, authorization, and error handling for you. Mike Deck of AWS gave an excellent presentation on EventBridge, available on YouTube, AWS re:Invent 2019: Building event-driven architectures w/ Amazon EventBridge.
Amazon EventBridge SaaS Integrations
Amazon EventBridge ingests data from supported SaaS partners and routes it to AWS service targets. With EventBridge, you can use data from your SaaS applications to trigger workflows for customer support, business operations, and more. Currently supported SaaS partners include Auth0, Datadog, Epsagon, MongoDB, New Relic, and PagerDuty, amongst others.
Below, we see an example of routing custom events from the SaaS vendor’s account to multiple targets within the customer’s account using Amazon EventBridge. We can also use interface endpoints powered by AWS PrivateLink within both accounts to eliminate the need to send data over the public Internet. Traffic to targets of EventBridge also remains on the AWS global infrastructure.
JDBC and ODBC Database Connections
Lastly, for data sources on AWS, interactions between vendor and customer AWS Accounts can also be made using JDBC (Java Database Connectivity) and ODBC (Open Database Connectivity) database connections. Services such as Amazon Redshift, Amazon Athena, Amazon RDS, and Amazon Aurora can all be accessed using one or both of these two methods. There multiple ways to enhance the security of the connections, including SSL/TLS connections, data encryption at rest and in flight, AWS IAM service-linked roles, VPCs, and VPC peering.
Below, we see an example of the application in the SaaS vendor’s account communicating over JDBC, with a private Amazon RDS instance in the customer’s account. We can communicate between private subnets, within two different accounts using VPC peering. Like PrivateLink, traffic stays on the global AWS backbone, and never traverses the public Internet.
Conclusion
In this post, we examined several AWS services that help a SaaS vendor interact with their customer’s accounts. In future posts, we will take a more in-depth look at the advantages and disadvantages of each service with respect to their scalability and a vendor’s tenant isolation strategy.
This blog represents my own viewpoints and not of my employer, Amazon Web Services.