Archive for category DevOps
Ten Ways to Leverage Generative AI for Development on AWS
Posted by Gary A. Stafford in AI/ML, AWS, Bash Scripting, Big Data, Build Automation, Client-Side Development, Cloud, DevOps, Enterprise Software Development, Kubernetes, Python, Serverless, Software Development, SQL on April 3, 2023
Explore ten ways you can use Generative AI coding tools to accelerate development and increase your productivity on AWS
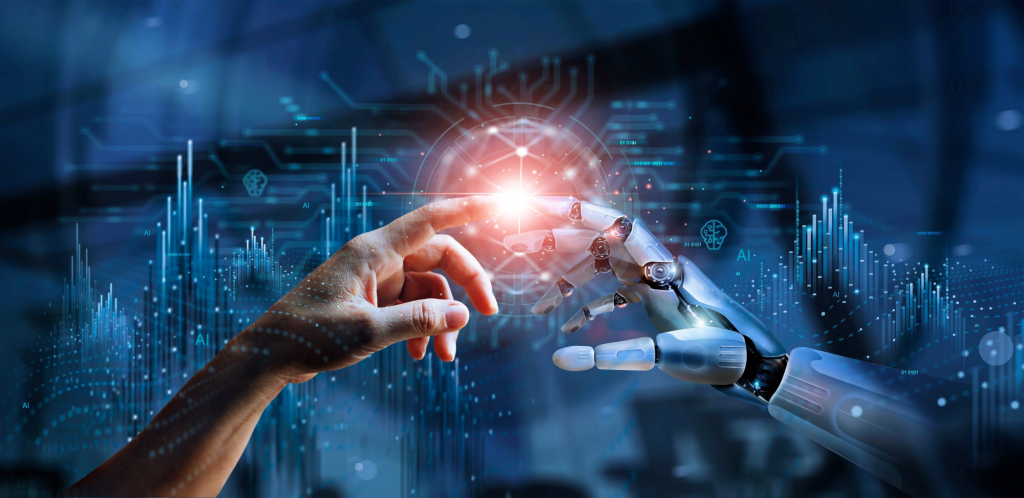
Generative AI coding tools are a new class of software development tools that leverage machine learning algorithms to assist developers in writing code. These tools use AI models trained on vast amounts of code to offer suggestions for completing code snippets, writing functions, and even entire blocks of code.
Quote generated by OpenAI ChatGPT
Introduction
Combining the latest Generative AI coding tools with a feature-rich and extensible IDE and your coding skills will accelerate development and increase your productivity. In this post, we will look at ten examples of how you can use Generative AI coding tools on AWS:
- Application Development: Code, unit tests, and documentation
- Infrastructure as Code (IaC): AWS CloudFormation, AWS CDK, Terraform, and Ansible
- AWS Lambda: Serverless, event-driven functions
- IAM Policies: AWS IAM policies and Amazon S3 bucket policies
- Structured Query Language (SQL): Amazon RDS, Amazon Redshift, Amazon Athena, and Amazon EMR
- Big Data: Apache Spark and Flink on Amazon EMR, AWS Glue, and Kinesis Data Analytics
- Configuration and Properties files: Amazon MSK, Amazon EMR, and Amazon OpenSearch
- Apache Airflow DAGs: Amazon MWAA
- Containerization: Kubernetes resources, Helm Charts, Dockerfiles for Amazon EKS
- Utility Scripts: PowerShell, Bash, Shell, and Python
Choosing a Generative AI Coding Tool
In my recent post, Accelerating Development with Generative AI-Powered Coding Tools, I reviewed six popular tools: ChatGPT, Copilot, CodeWhisperer, Tabnine, Bing, and ChatSonic.
For this post, we will use GitHub Copilot, powered by OpenAI Codex, a new AI system created by OpenAI. Copilot suggests code and entire functions in real-time, right from your IDE. Copilot is trained in all languages that appear in GitHub’s public repositories. GitHub points out that the quality of suggestions you receive may depend on the volume and diversity of training data for that language. Similar tools in this category are limited in the number of languages they support compared to Copilot.

Copilot is currently available as an extension for Visual Studio Code, Visual Studio, Neovim, and JetBrains suite of IDEs. The GitHub Copilot extension for Visual Studio Code (VS Code) already has 4.8 million downloads, and the GitHub Copilot Nightly extension, used for this post, has almost 280,000 downloads. I am also using the GitHub Copilot Labs extension in this post.

Ten Ways to Leverage Generative AI
Take a look at ten examples of how you can use Generative AI coding tools to increase your development productivity on AWS. All the code samples in this post can be found on GitHub.
1. Application Development
According to GitHub, trained on billions of lines of code, GitHub Copilot turns natural language prompts into coding suggestions across dozens of languages. These features make Copilot ideal for developing applications, writing unit tests, and authoring documentation. You can use GitHub Copilot to assist with writing software applications in nearly any popular language, including Go.
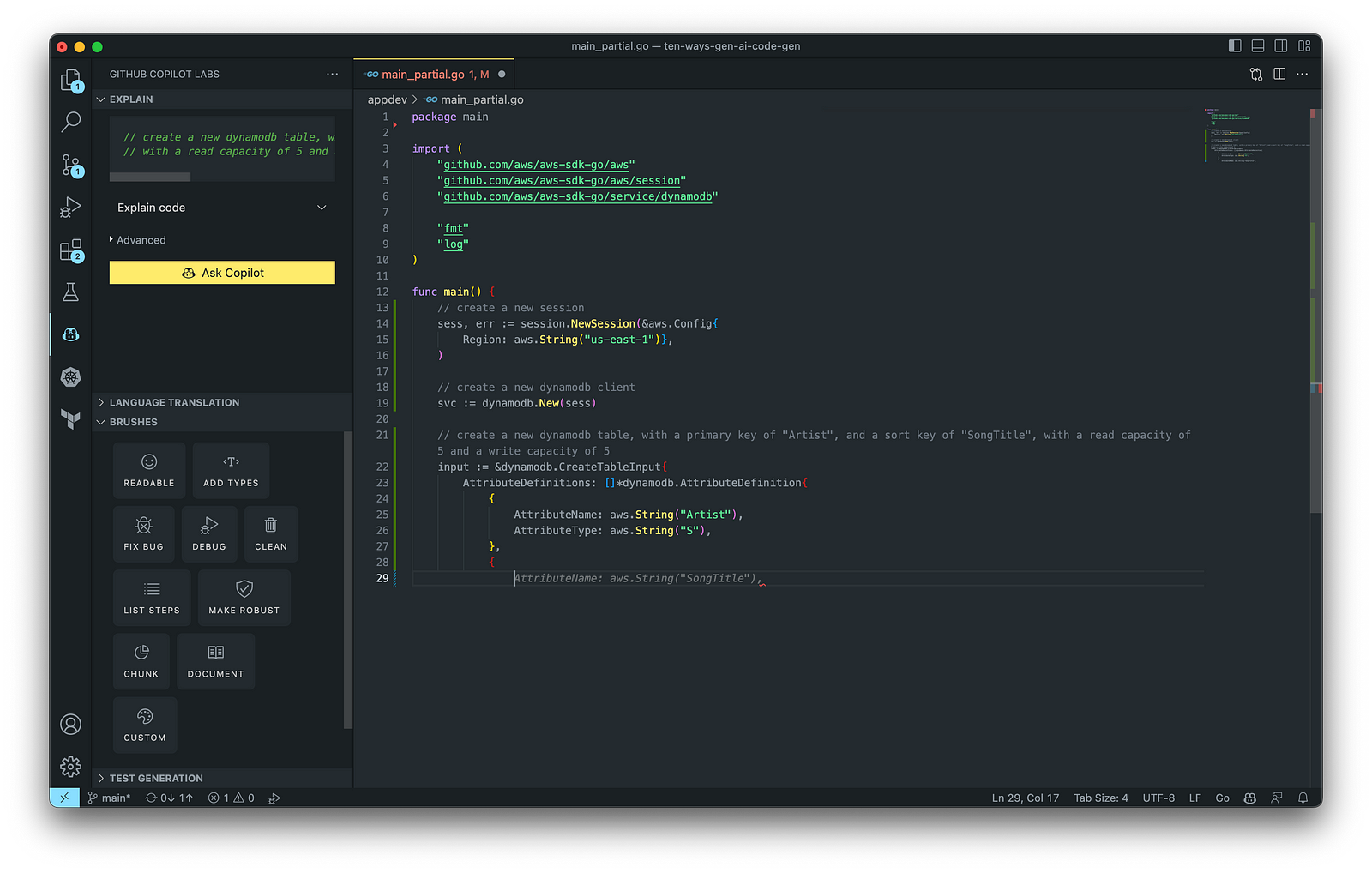
The final application, which uses the AWS SDK for Go to create an Amazon DynamoDB table, shown below, was formatted using the Go extension by Google and optimized using the ‘Readable,’ ‘Make Robust,’ and ‘Fix Bug’ GitHub Code Brushes.

Generating Unit Tests
Using JavaScript and TypeScript, you can take advantage of TestPilot to generate unit tests based on your existing code and documentation. TestPilot, part of GitHub Copilot Labs, uses GitHub Copilot’s AI technology.

2. Infrastructure as Code (IaC)
Widespread Infrastructure as Code (IaC) tools include Pulumi, AWS CloudFormation, Azure ARM Templates, Google Deployment Manager, AWS Cloud Development Kit (AWS CDK), Microsoft Bicep, and Ansible. Many IaC tools, except AWS CDK, use JSON- or YAML-based domain-specific languages (DSLs).
AWS CloudFormation
AWS CloudFormation is an Infrastructure as Code (IaC) service that allows you to easily model, provision, and manage AWS and third-party resources. The CloudFormation template is a JSON or YAML formatted text file. You can use GitHub Copilot to assist with writing IaC, including AWS CloudFormation in either JSON or YAML.

You can use the YAML Language Support by Red Hat extension to write YAML in VS Code.

VS Code has native JSON support with JSON Schema Store, which includes AWS CloudFormation. VS Code uses the CloudFormation schema for IntelliSense and flag schema errors in templates.

HashiCorp Terraform
In addition to AWS CloudFormation, HashiCorp Terraform is an extremely popular IaC tool. According to HashiCorp, Terraform lets you define resources and infrastructure in human-readable, declarative configuration files and manages your infrastructure’s lifecycle. Using Terraform has several advantages over manually managing your infrastructure.
Terraform plugins called providers let Terraform interact with cloud platforms and other services via their application programming interfaces (APIs). You can use the AWS Provider to interact with the many resources supported by AWS.

3. AWS Lambda
Lambda, according to AWS, is a serverless, event-driven compute service that lets you run code for virtually any application or backend service without provisioning or managing servers. You can trigger Lambda from over 200 AWS services and software as a service (SaaS) applications and only pay for what you use. AWS Lambda natively supports Java, Go, PowerShell, Node.js, C#, Python, and Ruby. AWS Lambda also provides a Runtime API allowing you to use additional programming languages to author your functions.
You can use GitHub Copilot to assist with writing AWS Lambda functions in any of the natively supported languages. You can further optimize the resulting Lambda code with GitHub’s Code Brushes.

The final Python-based AWS Lambda, below, was formatted using the Black Formatter and Flake8 extensions and optimized using the ‘Readable,’ ‘Debug,’ ‘Make Robust,’ and ‘Fix Bug’ GitHub Code Brushes.

You can easily convert the Python-based AWS Lambda to Java using GitHub Copilot Lab’s ability to translate code between languages. Install the GitHub Copilot Labs extension for VS Code to try out language translation.

4. IAM Policies
AWS Identity and Access Management (AWS IAM) is a web service that helps you securely control access to AWS resources. According to AWS, you manage access in AWS by creating policies and attaching them to IAM identities (users, groups of users, or roles) or AWS resources. A policy is an object in AWS that defines its permissions when associated with an identity or resource. IAM policies are stored on AWS as JSON documents. You can use GitHub Copilot to assist in writing IAM Policies.

The final AWS IAM Policy, below, was formatted using VS Code’s built-in JSON support.

5. Structured Query Language (SQL)
SQL has many use cases on AWS, including Amazon Relational Database Service (RDS) for MySQL, PostgreSQL, MariaDB, Oracle, and SQL Server databases. SQL is also used with Amazon Aurora, Amazon Redshift, Amazon Athena, Apache Presto, Trino (PrestoSQL), and Apache Hive on Amazon EMR.
You can use IDEs like VS Code with its SQL dialect-specific language support and formatted extensions. You can further optimize the resulting SQL statements with GitHub’s Code Brushes.

The final PostgreSQL script, below, was formatted using the Sql Formatter extension and optimized using the ‘Readable’ and ‘Fix Bug’ GitHub Code Brushes.

6. Big Data
Big Data, according to AWS, can be described in terms of data management challenges that — due to increasing volume, velocity, and variety of data — cannot be solved with traditional databases. AWS offers managed versions of Apache Spark, Apache Flink, Apache Zepplin, and Jupyter Notebooks on Amazon EMR, AWS Glue, and Amazon Kinesis Data Analytics (KDA).
Apache Spark
According to their website, Apache Spark is a multi-language engine for executing data engineering, data science, and machine learning on single-node machines or clusters. Spark jobs can be written in various languages, including Python (PySpark), SQL, Scala, Java, and R. Apache Spark is available on a growing number of AWS services, including Amazon EMR and AWS Glue.

The final Python-based Apache Spark job, below, was formatted using the Black Formatter extension and optimized using the ‘Readable,’ ‘Document,’ ‘Make Robust,’ and ‘Fix Bug’ GitHub Code Brushes.

7. Configuration and Properties Files
According to TechTarget, a configuration file (aka config) defines the parameters, options, settings, and preferences applied to operating systems, infrastructure devices, and applications. There are many examples of configuration and properties files on AWS, including Amazon MSK Connect (Kafka Connect Source/Sink Connectors), Amazon OpenSearch (Filebeat, Logstash), and Amazon EMR (Apache Log4j, Hive, and Spark).
Kafka Connect
Kafka Connect is a tool for scalably and reliably streaming data between Apache Kafka and other systems. It makes it simple to quickly define connectors that move large collections of data into and out of Kafka. AWS offers a fully-managed version of Kafka Connect: Amazon MSK Connect. You can use GitHub Copilot to write Kafka Connect Source and Sink Connectors with Kafka Connect and Amazon MSK Connect.

The final Kafka Connect Source Connector, below, was formatted using VS Code’s built-in JSON support. It incorporates the Debezium connector for MySQL, Avro file format, schema registry, and message transformation. Debezium is a popular open source distributed platform for performing change data capture (CDC) with Kafka Connect.

8. Apache Airflow DAGs
Apache Airflow is an open-source platform for developing, scheduling, and monitoring batch-oriented workflows. Airflow’s extensible Python framework enables you to build workflows connecting with virtually any technology. DAG (Directed Acyclic Graph) is the core concept of Airflow, collecting Tasks together, organized with dependencies and relationships to say how they should run.
Amazon Managed Workflows for Apache Airflow (Amazon MWAA) is a managed orchestration service for Apache Airflow. You can use GitHub Copilot to assist in writing DAGs for Apache Airflow, to be used with Amazon MWAA.

The final Python-based Apache Spark job, below, was formatted using the Black Formatter extension. Unfortunately, based on my testing, code optimization with GitHub’s Code Brushes is impossible with Airflow DAGs.

9. Containerization
According to Check Point Software, Containerization is a type of virtualization in which all the components of an application are bundled into a single container image and can be run in isolated user space on the same shared operating system. Containers are lightweight, portable, and highly conducive to automation. AWS describes containerization as a software deployment process that bundles an application’s code with all the files and libraries it needs to run on any infrastructure.
AWS has several container services, including Amazon Elastic Container Service (Amazon ECS), Amazon Elastic Kubernetes Service (Amazon EKS), Amazon Elastic Container Registry (Amazon ECR), and AWS Fargate. Several code-based resources can benefit from a Generative AI coding tool like GitHub Copilot, including Dockerfiles, Kubernetes resources, Helm Charts, Weaveworks Flux, and ArgoCD configuration.
Kubernetes
Kubernetes objects are represented in the Kubernetes API and expressed in YAML format. Below is a Kubernetes Deployment resource file, which creates a ReplicaSet to bring up multiple replicas of nginx
Pods.

The final Kubernetes resource file below contains Deployment and Service resources. In addition to GitHub Copilot, you can use Microsoft’s Kubernetes extension for VS Code to use IntelliSense and flag schema errors in the file.
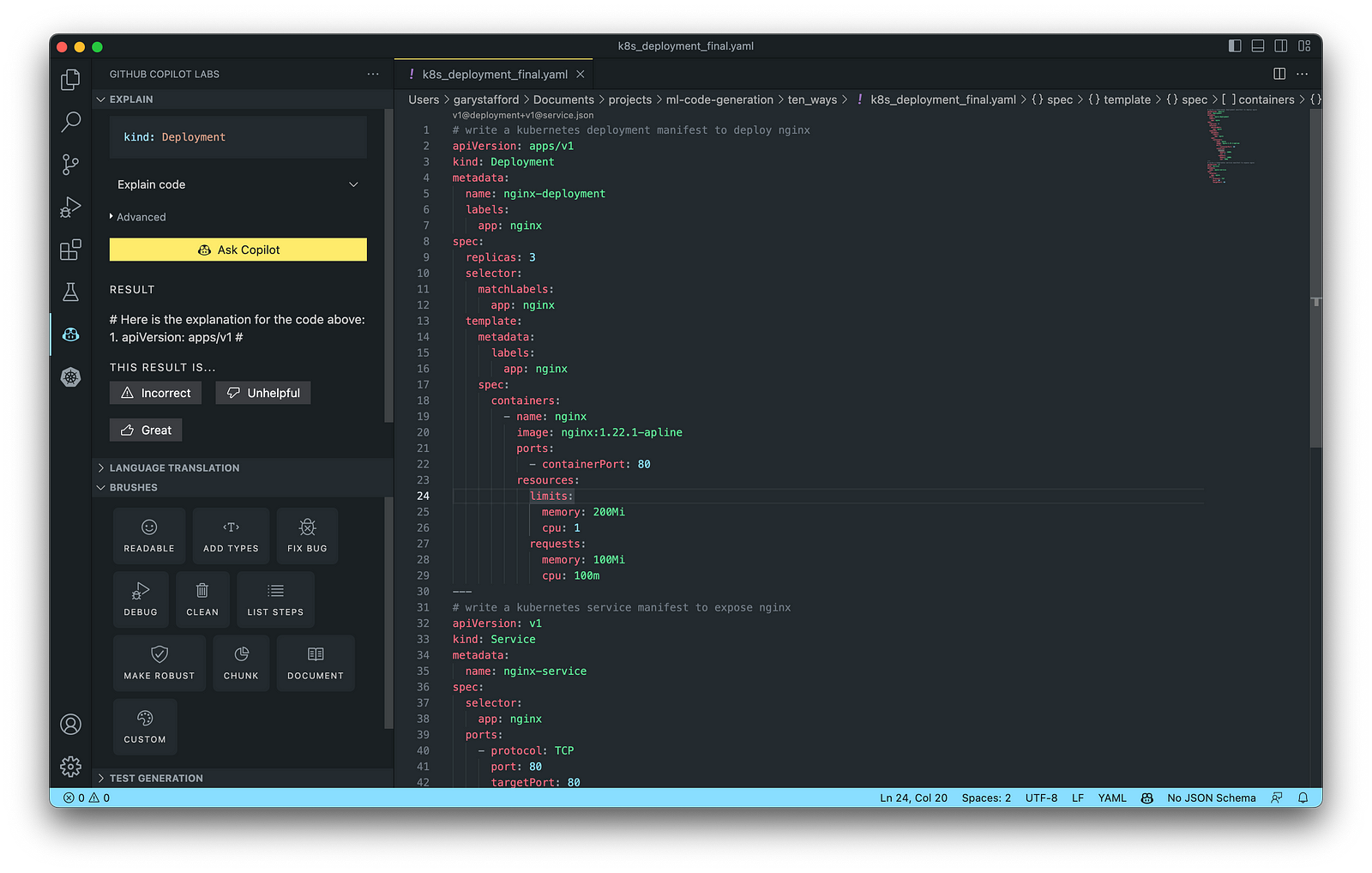
10. Utility Scripts
According to Bing AI — Search, utility scripts are small, simple snippets of code written as independent code files designed to perform a particular task. Utility scripts are commonly written in Bash, Shell, Python, Ruby, PowerShell, and PHP.
AWS utility scripts leverage the AWS Command Line Interface (AWS CLI) for Bash and Shell and AWS SDK for other programming languages. SDKs take the complexity out of coding by providing language-specific APIs for AWS services. For example, Boto3, AWS’s Python SDK, easily integrates your Python application, library, or script with AWS services, including Amazon S3, Amazon EC2, Amazon DynamoDB, and more.

An example of a Python script to calculate the total size of an Amazon S3 bucket, below, was inspired by 100daysofdevops/N-days-of-automation, a fantastic set of open source AWS-oriented automation scripts.

Conclusion
In this post, you learned ten ways to leverage Generative AI coding tools like GitHub Copilot for development on AWS. You saw how combining the latest generation of Generative AI coding tools, a mature and extensible IDE, and your coding experience will accelerate development, increase productivity, and reduce cost.
🔔 To keep up with future content, follow Gary Stafford on LinkedIn.
This blog represents my viewpoints and not those of my employer, Amazon Web Services (AWS). All product names, logos, and brands are the property of their respective owners.
Developing a Multi-Account AWS Environment Strategy
Posted by Gary A. Stafford in AWS, Cloud, DevOps, Enterprise Software Development, Technology Consulting on March 11, 2023
Explore twelve common patterns for developing an effective and efficient multi-account AWS environment strategy

Introduction
Every company is different: its organizational structure, the length of time it has existed, how fast it has grown, the industries it serves, its product and service diversity, public or private sector, and its geographic footprint. This uniqueness is reflected in how it organizes and manages its Cloud resources. Just as no two organizations are exactly alike, the structure of their AWS environments is rarely identical.
Some organizations successfully operate from a single AWS account, while others manage workloads spread across dozens or even hundreds of accounts. The volume and purpose of an organization’s AWS accounts are a result of multiple factors, including length of time spent on AWS, Cloud maturity, organizational structure and complexity, sectors, industries, and geographies served, product and service mix, compliance and regulatory requirements, and merger and acquisition activity.
“By design, all resources provisioned within an AWS account are logically isolated from resources provisioned in other AWS accounts, even within your own AWS Organizations.” (AWS)
Working with industry peers, the AWS community, and a wide variety of customers, one will observe common patterns for how organizations separate environments and workloads using AWS accounts. These patterns form an AWS multi-account strategy for operating securely and reliably in the Cloud at scale. The more planning an organization does in advance to develop a sound multi-account strategy, the less the burden that is required to manage changes as the organization grows over time.
The following post will explore twelve common patterns for effectively and efficiently organizing multiple AWS accounts. These patterns do not represent an either-or choice; they are designed to be purposefully combined to form a multi-account AWS environment strategy for your organization.
Patterns
- Pattern 1: Single “Uber” Account
- Pattern 2: Non-Prod/Prod Environments
- Pattern 3: Upper/Lower Environments
- Pattern 4: SDLC Environments
- Pattern 5: Major Workload Separation
- Pattern 6: Backup
- Pattern 7: Sandboxes
- Pattern 8: Centralized Management and Governance
- Pattern 9: Internal/External Environments
- Pattern 10: PCI DSS Workloads
- Pattern 11: Vendors and Contractors
- Pattern 12: Mergers and Acquisitions
Patterns 1–8 are progressively more mature multi-account strategies, while Patterns 9–12 represent special use cases for supplemental accounts.
Multi-Account Advantages
According to AWS’s whitepaper, Organizing Your AWS Environment Using Multiple Accounts, the benefits of using multiple AWS accounts include the following:
- Group workloads based on business purpose and ownership
- Apply distinct security controls by environment
- Constrain access to sensitive data (including compliance and regulation)
- Promote innovation and agility
- Limit the scope of impact from adverse events
- Support multiple IT operating models
- Manage costs (budgeting and cost attribution)
- Distribute AWS Service Quotas (fka limits) and API request rate limits
As we explore the patterns for organizing your AWS accounts, we will see how and to what degree each of these benefits is demonstrated by that particular pattern.
AWS Control Tower
Discussions about AWS multi-account environment strategies would not be complete without mentioning AWS Control Tower. According to the documentation, “AWS Control Tower offers a straightforward way to set up and govern an AWS multi-account environment, following prescriptive best practices.” AWS Control Tower includes Landing zone, described as “a well-architected, multi-account environment based on security and compliance best practices.”
AWS Control Tower is prescriptive in the Shared accounts it automatically creates within its AWS Organizations’ organizational units (OUs). Shared accounts created by AWS Control Tower include the Management, Log Archive, and Audit accounts. The previous standalone AWS service, AWS Landing Zone, maintained slightly different required accounts, including Shared Services, Log Archive, Security, and optional Network accounts. Although prescriptive, AWS Control Tower is also flexible and relatively unopinionated regarding the structure of Member accounts. Member accounts can be enrolled or unenrolled in AWS Control Tower.
You can decide whether or not to implement AWS Organizations or AWS Control Tower to set up and govern your AWS multi-account environment. Regardless, you will still need to determine how to reflect your organization’s unique structure and requirements in the purpose and quantity of the accounts you create within your AWS environment.
Common Multi-Account Patterns
While working with peers, community members, and a wide variety of customers, I regularly encounter the following twelve patterns for organizing AWS accounts. As noted earlier, these patterns do not represent an either-or choice; they are designed to be purposefully combined to form a multi-account AWS environment strategy for your organization.
Pattern 1: Single “Uber” Account
Organizations that effectively implement Pattern 1: Single “Uber” Account organize and separate environments and workloads at the sub-account level. They often use Amazon Virtual Private Cloud (Amazon VPC), an AWS account-level construct, to organize and separate environments and workloads. They may also use Subnets (VPC-level construct) or AWS Regions and Availability Zones to further organize and separate environments and workloads.
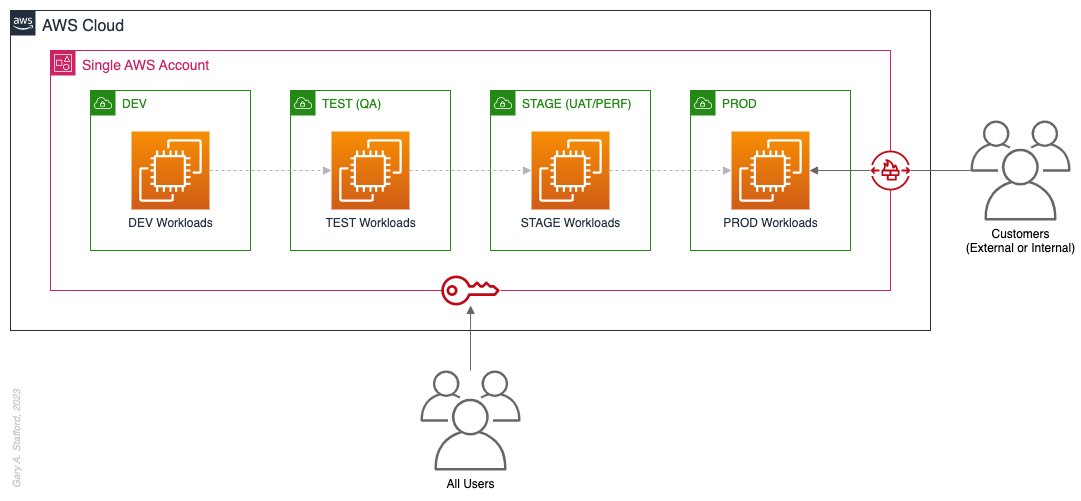
Pros
- Few, if any, significant advantages, especially for customer-facing workloads
Cons
- Decreased ability to limit the scope of impact from adverse events (widest blast radius)
- If the account is compromised, then all the organization’s workloads and data, possibly the entire organization, are potentially compromised (e.g., Ransomware attacks such as Encryptors, Lockers, and Doxware)
- Increased risk that networking or security misconfiguration could lead to unintended access to sensitive workloads and data
- Increased risk that networking, security, or resource management misconfiguration could lead to broad or unintended impairment of all workloads
- Decreased ability to perform team-, environment-, and workload-level budgeting and cost attribution
- Increased risk of resource depletion (soft and hard service quotas)
- Reduced ability to conduct audits and demonstrate compliance
Pattern 2: Non-Prod/Prod Environments
Organizations that effectively implement Pattern 2: Non-Prod/Prod Environments organize and separate non-Production workloads from Production (PROD) workloads using separate AWS accounts. Most often, they use Amazon VPCs within the non-Production account to separate workloads or Software Development Lifecycle (SDLC) environments, most often Development (DEV), Testing (TEST) or Quality Assurance (QA), and Staging (STAGE). Alternatively, these environments might also be designated as “N-1” (previous release), “N” (current release), “N+1” (next release), “N+2” (in development), and so forth, based on the currency of that version of that workload.
In some organizations, the Staging environment is used for User Acceptance Testing (UAT), performance (PERF) testing, and load testing before releasing workloads to Production. While in other organizations, STAGE, UAT, and PERF are each treated as separate environments at the account or VPC level.
Isolating Production workloads into their own account(s) and strictly limiting access to those workloads represents a significant first step in improving the overall maturity of your multi-account AWS environment strategy.

Pros
- Limits the scope of impact on Production as a result of adverse non-Production events (narrower blast radius)
- Logical separation and security of Production workloads and data
- Tightly control and limit access to Production, including the use of Break-the-Glass procedures (aka Break-glass or BTG); draws its name from “breaking the glass to pull a fire alarm”
- Eliminate the risk that non-Production networking or security misconfiguration could lead to unintended access to sensitive Production workloads and data
- Eliminate the chance that non-Production networking, security, or resource management misconfiguration could lead to broad or unintended impairment of Production workloads
- Conduct audits and demonstrate compliance with Production workloads
Cons
- If the Production account is compromised, then all the organization’s customer-facing workloads and data are potentially compromised
- Decreased ability to perform team-, environment-, and workload-level budgeting and cost attribution in the shared non-Production environment
- Increased risk of resource depletion (soft and hard service quotas) in the non-Production environment account
Pattern 3: Upper/Lower Environments
The next pattern, Pattern 3: Upper/Lower Environments, is a finer-grain variation of Pattern 2. With Pattern 3, we split all “Lower” environments into a single account and each “Upper” environment into its own account. In the software development process, initial environments, such as CI/CD for automated testing of code and infrastructure, Development, Test, UAT, and Performance, are called “Lower” environments. Conversely, later environments, such as Staging, Production, and even Disaster Recovery (DR), are called “Upper” environments. Upper environments typically require isolation for stability during testing or security for Production workloads and sensitive data.
Often, courser-grain patterns like Patterns 1–3 are carryovers from more traditional on-premises data centers, where compute, storage, network, and security resources were more constrained. Although these patterns can be successfully reproduced in the Cloud, they may not be optimal compared to more “cloud native” patterns, which provide improved separation of concerns.

Pros
- Limits the scope of impact on individual Upper environments as a result of adverse Lower environment events (narrower blast radius)
- Increased stability of Staging environment for critical UAT, performance, and load testing
- Logical separation and security of Production workloads and data
- Tightly control and limit access to Production, including the use of BTG
- Eliminate the risk that non-Production networking or security misconfiguration could lead to unintended access to sensitive Production workloads and data
- Eliminate the chance that non-Production networking, security, or resource management misconfiguration could lead to broad or unintended impairment of Production workloads
- Conduct audits and demonstrate compliance with Production workloads
Cons
- If the Production account is compromised, then all the organization’s customer-facing workloads and data are potentially compromised (e.g., Ransomware attack)
- Decreased ability to perform team-, environment-, and workload-level budgeting and cost attribution in the shared Lower environment
- Increased risk of resource depletion (soft and hard service quotas) in the Lower environment account
Pattern 4: SDLC Environments
The next pattern, Pattern 4: SDLC Environments, is a finer-grain variation of Pattern 3. With Pattern 4, we gain complete separation of each SDLC environment into its own AWS account. Using AWS services like AWS IAM Identity Center (fka AWS SSO), the Security team can enforce least-privilege permissions at an AWS Account level to individual groups of users, such as Developers, Testers, UAT, and Performance testers.
Based on my experience, Pattern 4 represents the minimal level of workload separation an organization should consider when developing its multi-account AWS environment strategy. Although Pattern 4 has a number of disadvantages, when combined with subsequent patterns and AWS best practices, this pattern begins to provide a scalable foundation for an organization’s growing workload portfolio.

Patterns, such as Pattern 4, not only apply to traditional software applications and services. These patterns can be applied to data analytics, AI/ML, IoT, media services, and similar workloads where separation of environments is required.

Pros
- Limits the scope of impact on one SDLC environment as a result of adverse events in another environment (narrower blast radius)
- Logical separation and security of Production workloads and data
- Increased stability of each SDLC environment
- Tightly control and limit access to Production, including the use of BTG
- Reduced risk that non-Production networking or security misconfiguration could lead to unintended access to sensitive Production workloads and data
- Reduced risk that non-Production networking, security, or resource management misconfiguration could lead to broad or unintended impairment of Production workloads
- Conduct audits and demonstrate compliance with Production workloads
- Increased ability to perform budgeting and cost attribution for each SDLC environment
- Reduced risk of resource depletion (soft and hard service limits) and IP conflicts and exhaustion within any single SDLC environment
Cons
- All workloads for each SDLC environment run within a single account, including Production, increasing the potential scope of impact from adverse events within that environment’s account
- If the Production account is compromised, then all the organization’s customer-facing workloads and data are potentially compromised
- Decreased ability to perform workload-level budgeting and cost attribution
Pattern 5: Major Workload Separation
The next pattern, Pattern 5: Major Workload Separation, is a finer-grain variation of Pattern 4. With Pattern 5, we separate each significant workload into its own separate SDLC environment account. The security team can enforce fine-grain least-privilege permissions at an AWS Account level to individual groups of users, such as Developers, Testers, UAT, and Performance testers, by their designated workload(s).
Pattern 5 has several advantages over the previous patterns. In addition to the increased workload-level security and reliability benefits, Pattern 5 can be particularly useful for organizations that operate significantly different technology stacks and specialized workloads, particularly at scale. Different technology stacks and specialized workloads often each have their own unique development, testing, deployment, and support processes. Isolating these types of workloads will help facilitate the support of multiple IT operating models.

Pros
- Limits the scope of impact on an individual workload as a result of adverse events from another workload or SDLC environment (narrowest blast radius)
- Increased ability to perform team-, environment-, and workload-level budgeting and cost attribution
Cons
- If the Production account is compromised, then all the organization’s customer-facing workloads and data are potentially compromised (e.g., Ransomware attack)
Pattern 6: Backup
In the earlier patterns, we mentioned that if the Production account were compromised, all the organization’s customer-facing workloads and data could be compromised. According to TechTarget, 2022 was a breakout year for Ransomware attacks. According to the US government’s CISA.gov website, “Ransomware is a form of malware designed to encrypt files on a device, rendering any files and the systems that rely on them unusable. Malicious actors then demand ransom in exchange for decryption.”
According to AWS best practices, one of the recommended preparatory actions to protect and recover from Ransomware attacks is backing up data to an alternate account using tools such as AWS Backup and an AWS Backup vault. Solutions such as AWS Backup protect and restore data regardless of how it was made inaccessible.
In Pattern 6: Backup, we create one or more Backup accounts to protect against unintended data loss or account compromise. In the example below, we have two Backup accounts, one for Production data and one for all non-Production data.

Pros
- If the Production account is compromised (e.g., Ransomware attacks such as Encryptors, Lockers, and Doxware), there are secure backups of data stored in a separate account, which can be used to restore or recreate the Production environment
Cons
- Few, if any, significant disadvantages when combined with previous patterns and AWS best-practices
Pattern 7: Sandboxes
The following pattern, Pattern 7: Sandboxes, supplements the previous patterns, designed to address the needs of an organization to allow individual users and teams to learn, build, experiment, and innovate on AWS without impacting the larger organization’s AWS environment. To quote the AWS blog, Best practices for creating and managing sandbox accounts in AWS, “Many organizations need another type of environment, one where users can build and innovate with AWS services that might not be permitted in production or development/test environments because controls have not yet been implemented.” Further, according to TechTarget, “a Sandbox is an isolated testing environment that enables users to run programs or open files without affecting the application, system, or platform on which they run.”
Due to the potential volume of individual user and team accounts, sometimes referred to as Sandbox accounts, mature infrastructure automation practices, cost controls, and self-service provisioning and de-provisioning of Sandbox accounts are critical capabilities for the organization.

Pros
- Allow individual users and teams to learn, build, experiment, and innovate on AWS without impacting the rest of the organization’s AWS environment
Cons
- Without mature automation practices, cost controls, and self-service capabilities, managing multiple individual and team Sandbox accounts can become unwieldy and costly
Pattern 8: Centralized Management and Governance
We discussed AWS Control Tower at the beginning of this post. AWS Control Tower is prescriptive in creating Shared accounts within its AWS Organizations’ organizational units (OUs), including the Management, Log Archive, and Audit accounts. AWS encourages using AWS Control Tower to orchestrate multiple AWS accounts and services on your behalf while maintaining your organization’s security and compliance needs.
As exemplified in Pattern 8: Centralized Management and Governance, many organizations will implement centralized management whether or not they decide to implement AWS Control Tower. In addition to the Management account (payer account, fka master account), organizations often create centralized logging accounts, and centralized tooling (aka Shared services) accounts for functions such as CI/CD, IaC provisioning, and deployment. Another common centralized management account is a Security account. Organizations use this account to centralize the monitoring, analysis, notification, and automated mitigation of potential security issues within their AWS environment. The Security accounts will include services such as Amazon Detective, Amazon Inspector, Amazon GuardDuty, and AWS Security Hub.

Pros
- Increased ability to manage and maintain multiple AWS accounts with fewer resources
- Reduced duplication of management resources across accounts
- Increased ability to use automation and improve the consistency of processes and procedures across multiple accounts
Cons
- Few, if any, significant disadvantages when combined with previous patterns and AWS best-practices
Pattern 9: Internal/External Environments
The next pattern, Pattern 9: Internal/External Environments, focuses on organizations with internal operational systems (aka Enterprise systems) in the Cloud and customer-facing workloads. Pattern 9 separates internal operational systems, platforms, and workloads from external customer-facing workloads. For example, an organization’s divisions and departments, such as Sales and Marketing, Finance, Human Resources, and Manufacturing, are assigned their own AWS account(s). Pattern 9 allows the Security team to ensure that internal departmental or divisional users are isolated from users who are responsible for developing, testing, deploying, and managing customer-facing workloads.
Note that the diagram for Pattern 9 shows remote users who access AWS End User Computing (EUC) services or Virtual Desktop Infrastructure (VDI), such as Amazon WorkSpaces and Amazon AppStream 2.0. In this example, remote workers have secure access to EUC services provisioned in a separate AWS account, and indirectly, internal systems, platforms, and workloads.

Pros
- Separation of internal operational systems, platforms, and workloads from external customer-facing workloads
Cons
- Few, if any, significant disadvantages when combined with previous patterns and AWS best-practices
Pattern 10: PCI DSS Workloads
The next pattern, Pattern 10: PCI DSS Workloads, is a variation of previous patterns, which assumes the existence of Payment Card Industry Data Security Standard (PCI DSS) workloads and data. According to the AWS, “PCI DSS applies to entities that store, process, or transmit cardholder data (CHD) or sensitive authentication data (SAD), including merchants, processors, acquirers, issuers, and service providers. The PCI DSS is mandated by the card brands and administered by the Payment Card Industry Security Standards Council.”
According to AWS’s whitepaper, Architecting for PCI DSS Scoping and Segmentation on AWS, “By design, all resources provisioned within an AWS account are logically isolated from resources provisioned in other AWS accounts, even within your own AWS Organizations. Using an isolated account for PCI workloads is a core best practice when designing your PCI application to run on AWS.” With Pattern 10, we separate non-PCI DSS and PCI DSS Production workloads and data. The assumption is that only Production contains PCI DSS data. Data in lower environments is synthetically generated or sufficiently encrypted, masked, obfuscated, or tokenized.
Note that the diagram for Pattern 10 shows Administrators. Administrators with different spans of responsibility and access are present in every pattern, whether specifically shown or not.

Pros
- Increased ability to meet compliance requirements by separating non-PCI DSS and PCI DSS Production workloads
Cons
- Few, if any, significant disadvantages when combined with previous patterns and AWS best-practices
Pattern 11: Vendors and Contractors
The next pattern, Pattern 11: Vendors and Contractors, is focused on organizations that employ contractors or use third-party vendors who provide products and services that interact with their AWS-based environment. Like Pattern 10, Pattern 11 allows the Security team to ensure that contractor and vendor-based systems’ access to internal systems and customer-facing workloads is tightly controlled and auditable.
Vendor-based products and services are often deployed within an organization’s AWS environment without external means of ingress or egress. Alternatively, a vendor’s product or service may have a secure means of ingress from or egress to external endpoints. Such is the case with some SaaS products, which ship an organization’s data to an external aggregator for analytics or a security vendor’s product that pre-filters incoming data, external to the organization’s AWS environment. Using separate AWS accounts can improve an organization’s security posture and mitigate the risk of adverse events on the organization’s overall AWS environment.

Pros
- Ensure access to internal systems and customer-facing workloads by contractors and vendor-based systems is tightly controlled and auditable
Cons
- Few, if any, significant disadvantages when combined with previous patterns and AWS best-practices
Pattern 12: Mergers and Acquisitions
The next pattern, Pattern 12: Mergers and Acquisitions, is focused on managing the integration of external AWS accounts as a result of a merger or acquisition. This is a common occurrence, but the exact details of how best to handle the integration of two or more integrations depend on several factors. Factors include the required level of integration, for example, maintaining separate AWS Organizations, maintaining different AWS accounts, or merging resources from multiple accounts. Other factors that might impact account structure include changes in ownership or payer of acquired accounts, existing acquired cost-savings agreements (e.g., EDPs, PPAs, RIs, and Savings Plans), and AWS Marketplace vendor agreements. Even existing authentication and authorization methods of the acquiree versus the acquirer (e.g., AWS IAM Identity Center, Microsoft Active Directory (AD), Azure AD, and external identity providers (IdP) like Okta or Auth0).
The diagram for Pattern 12 attempts to show a few different M&A account scenarios, including maintaining separate AWS accounts for the acquirer and acquiree (e.g., acquiree’s Manufacturing Division account). If desired, the accounts can be kept independent but managed within the acquirer’s AWS Organizations’ organization. The diagram also exhibits merging resources from the acquiree’s accounts into the acquirer’s accounts (e.g., Sales and Marketing accounts). Resources will be migrated or decommissioned, and the account will be closed.

Pros
- Maintain separation between an acquirer and an acquiree’s AWS accounts within a single organization’s AWS environment
- Potentially consolidate and maximize cost-saving advantages of volume-related financial agreements and vendor licensing
Cons
- Migrating workloads between different organizations, depending on their complexity, requires careful planning and testing
- Consolidating multiple authentication and authorization methods requires careful planning and testing to avoid improper privileges
- Consolidating and optimizing separate licensing and cost-saving agreements between multiple organizations requires careful planning and an in-depth understanding of those agreements
Multi-Account AWS Environment Example
You can form an efficient and effective multi-account strategy for your organization by purposefully combining multiple patterns. Below is an example of combining the features of several patterns: Major Workload Separation, Backup, Sandboxes, Centralized Management and Governance, Internal/External Environments, and Vendors and Contractors.
According to AWS, “You can use AWS Organizations’ organizational units (OUs) to group accounts together to administer as a single unit. This greatly simplifies the management of your accounts.” If you decide to use AWS Organizations, each set of accounts associated with a pattern could correspond to an OU: Major Workload A, Major Workload B, Sandboxes, Backups, Centralized Management, Internal Environments, Vendors, and Contractors.

Conclusion
This post taught us twelve common patterns for effectively and efficiently organizing your AWS accounts. Instead of an either-or choice, these patterns are designed to be purposefully combined to form a multi-account strategy for your organization. Having a sound multi-account strategy will improve your security posture, maintain compliance, decrease the impact of adverse events on your AWS environment, and improve your organization’s ability to safely and confidently innovate and experiment on AWS.
Recommended References
- Establishing Your Cloud Foundation on AWS (AWS Whitepaper)
- Organizing Your AWS Environment Using Multiple Accounts (AWS Whitepaper)
- Building a Cloud Operating Model (AWS Whitepaper)
- AWS Security Reference Architecture (AWS Prescriptive Guidance)
- AWS Security Maturity Model (AWS Whitepaper)
- AWS re:Invent 2022 — Best practices for organizing and operating on AWS (YouTube Video)
- AWS Break Glass Role (GitHub)
🔔 To keep up with future content, follow Gary Stafford on LinkedIn.
This blog represents my viewpoints and not those of my employer, Amazon Web Services (AWS). All product names, logos, and brands are the property of their respective owners.
Brief Introduction to Observability on AWS
Posted by Gary A. Stafford in AWS, Cloud, DevOps on March 9, 2023
Explore the wide variety of Application Performance Monitoring (APM) and Observability options on AWS

APM and Observability
Observability is “the extent to which the internal states of a system can be inferred from externally available data” (Gartner). The three pillars of observability data are metrics, logs, and traces. Application Performance Monitoring (APM), a term commonly associated with observability, is “software that enables the observation and analysis of application health, performance, and user experience” (Gartner).
Additional features often associated with APM and observability products and services include the following (in alphabetical order):
- Advanced Threat Protection (ATP)
- Endpoint Detection and Response (EDR)
- Incident Detection Response (IDR)
- Infrastructure Performance Monitoring (IPM)
- Network Device Monitoring (NDM)
- Network Performance Monitoring (NPM)
- OpenTelemetry (OTel)
- Operational (or Operations) Intelligence Platform
- Predictive Monitoring (predictive analytics / predictive modeling)
- Real User Monitoring (RUM)
- Security Information and Event Management (SIEM)
- Security Orchestration, Automation, and Response (SOAR)
- Synthetic Monitoring (directed monitoring / synthetic testing)
- Threat Visibility and Risk Scoring
- Unified Security and Observability Platform
- User Behavior Analytics (UBA)
Not all features are offered by all vendors. Most vendors tend to specialize in one or more areas. Determining which features are essential to your organization before choosing a solution is vital.
AI/ML
Given the growing volume and real-time nature of observability telemetry, many vendors have started incorporating AI and ML into their products and services to improve correlation, anomaly detection, and mitigation capabilities. Understand how these features can reduce operational burden, enhance insights, and simplify complexity.
Decision Factors
APM and observability tooling choices often come down to a “Build vs. Buy” decision for organizations. In the Cloud, this usually means integrating several individual purpose-built products and services, self-managed open-source projects, or investing in an end-to-end APM or unified observability platform. Other decision factors include the need for solutions to support:
- Hybrid cloud environments (on-premises/Cloud)
- Multi-cloud environments (Public Cloud, SaaS, Supercloud)
- Specialized workloads (e.g., Mainframes, HPC, VMware, SAP, SAS)
- Compliant workloads (e.g., PCI DSS, PII, GDPR, FedRAMP)
- Edge Computing and IoT/IIoT
- AI, ML, and Data Analytics monitoring (AIOps, MLOps, DataOps)
- SaaS observability (SaaS providers who offer monitoring to their end-users as part of their service offering)
- Custom log formats and protocols
Finally, the 5 V’s of big data: Velocity, Volume, Value, Variety, and Veracity, also influence the choice of APM and observability tooling. The real-time nature of the observability data, the sheer volume of the data, the source and type of data, and the sensitivity of the data, will all guide tooling choices based on features and cost.
Organizations can choose fully-managed native AWS services, AWS Partner products and services, often SaaS, self-managed open-source observability tooling, or a combination of options. Many AWS and Partner products and services are commercial versions of popular open-source software (COSS).
AWS Options
- Amazon CloudWatch: Observe and monitor resources and applications you run on AWS in real time. CloudWatch features include Amazon CloudWatch Container, Lambda, Contributor, and Application Insights.
- Amazon Managed Service for Prometheus (AMP): Prometheus-compatible service that monitors and provides alerts on containerized applications and infrastructure at scale. Prometheus is “an open-source systems monitoring and alerting toolkit originally built at SoundCloud.”
- Amazon Managed Grafana (AMG): Commonly paired with AMP, AMG is a fully managed service for Grafana, an open-source analytics platform that “enables you to query, visualize, alert on, and explore your metrics, logs, and traces wherever they are stored.”
- Amazon OpenSearch Service: Based on open-source OpenSearch, this service provides interactive log analytics and real-time application monitoring and includes OpenSearch Dashboards (comparable to Kibana). Recently announced new security analytics features provide threat monitoring, detection, and alerting capabilities.
- AWS X-Ray: Trace user requests through your application, viewed using the X-Ray service map console or integrated with CloudWatch using the CloudWatch console’s X-Ray Traces Service map.
Data Collection, Processing, and Forwarding
- AWS Distro for OpenTelemetry (ADOT): Open-source APIs, libraries, and agents to collect distributed traces and metrics for application monitoring. ADOT is an open-source distribution of OpenTelemetry, a “high-quality, ubiquitous, and portable telemetry [solution] to enable effective observability.”
- AWS for Fluent Bit: Fluent Bit image with plugins for both CloudWatch Logs and Kinesis Data Firehose. Fluent Bit is an open-source, “super fast, lightweight, and highly scalable logging and metrics processor and forwarder.”
Security-focused Monitoring
- AWS CloudTrail: Helps enable operational and risk auditing, governance, and compliance in your AWS environment. CloudTrail records events, including actions taken in the AWS Management Console, AWS Command Line Interface (CLI), AWS SDKs, and APIs.
Partner Options
According to the 2022 Gartner® Magic Quadrant™ — APM & Observability Report, leading vendors commonly used by AWS customers include the following (in alphabetical order):
- AppDynamics (Cisco)
- Datadog
- Dynatrace
- Elastic
- Honeycomb
- Instanta (IBM)
- logz.io
- New Relic
- Splunk
- Sumo Logic
Open Source Options
There are countless open-source observability projects to choose from, including the following (in alphabetical order):
- Elastic: Elastic Stack: Elasticsearch, Kibana, Beats, and Logstash
- Fluentd: Data collector for unified logging layer
- Grafana: Platform for monitoring and observability
- Jaeger: End-to-end distributed tracing
- Kiali: Configure, visualize, validate, and troubleshoot Istio Service Mesh
- Loki: Like Prometheus, but for logs
- OpenSearch: Scalable, flexible, and extensible software suite for search, analytics, and observability applications
- OpenTelemetry (OTel): Collection of tools, APIs, and SDKs used to instrument, generate, collect, and export telemetry data
- Prometheus: Monitoring system and time series database
- Zabbix: Single pane of glass view of your whole IT infrastructure stack
🔔 To keep up with future content, follow Gary Stafford on LinkedIn.
This blog represents my viewpoints and not those of my employer, Amazon Web Services (AWS). All product names, logos, and brands are the property of their respective owners.
Building and Deploying Cloud-Native Quarkus-based Java Applications to Kubernetes
Posted by Gary A. Stafford in AWS, Cloud, DevOps, Java Development, Kubernetes, Software Development on June 1, 2022
Developing, testing, building, and deploying Native Quarkus-based Java microservices to Kubernetes on AWS, using GitOps
Introduction
Although it may no longer be the undisputed programming language leader, according to many developer surveys, Java still ranks right up there with Go, Python, C/C++, and JavaScript. Given Java’s continued popularity, especially amongst enterprises, and the simultaneous rise of cloud-native software development, vendors have focused on creating purpose-built, modern JVM-based frameworks, tooling, and standards for developing applications — specifically, microservices.
Leading JVM-based microservice application frameworks typically provide features such as native support for a Reactive programming model, MicroProfile, GraalVM Native Image, OpenAPI and Swagger definition generation, GraphQL, CORS (Cross-Origin Resource Sharing), gRPC (gRPC Remote Procedure Calls), CDI (Contexts and Dependency Injection), service discovery, and distributed tracing.
Leading JVM-based Microservices Frameworks
Review lists of the most popular cloud-native microservices framework for Java, and you are sure to find Spring Boot with Spring Cloud, Micronaut, Helidon, and Quarkus at or near the top.
Spring Boot with Spring Cloud
According to their website, Spring makes programming Java quicker, easier, and safer for everybody. Spring’s focus on speed, simplicity, and productivity has made it the world’s most popular Java framework. Spring Boot makes it easy to create stand-alone, production-grade Spring based Applications that you can just run. Spring Boot’s many purpose-built features make it easy to build and run your microservices in production at scale. However, the distributed nature of microservices brings challenges. Spring Cloud can help with service discovery, load-balancing, circuit-breaking, distributed tracing, and monitoring with several ready-to-run cloud patterns. It can even act as an API gateway.
Helidon
Oracle’s Helidon is a cloud-native, open‑source set of Java libraries for writing microservices that run on a fast web core powered by Netty. Helidon supports MicroProfile, a reactive programming model, and, similar to Micronaut, Spring, and Quarkus, it supports GraalVM Native Image.
Micronaut
According to their website, the Micronaut framework is a modern, open-source, JVM-based, full-stack toolkit for building modular, easily testable microservice and serverless applications. Micronaut supports a polyglot programming model, discovery services, distributed tracing, and aspect-oriented programming (AOP). In addition, Micronaut offers quick startup time, blazing-fast throughput, and a minimal memory footprint.
Quarkus
Quarkus, developed and sponsored by RedHat, is self-described as the ‘Supersonic Subatomic Java.’ Quarkus is a cloud-native, Kubernetes-native, [Linux] container first, microservices first framework for writing Java applications. Quarkus is a Kubernetes Native Java stack tailored for OpenJDK HotSpot and GraalVM, crafted from over fifty best-of-breed Java libraries and standards.
Developing Native Quarkus Microservices
In the following post, we will develop, build, test, deploy, and monitor a native Quarkus microservice application to Kubernetes. The RESTful service will expose a rich Application Programming Interface (API) and interacts with a PostgreSQL database on the backend.
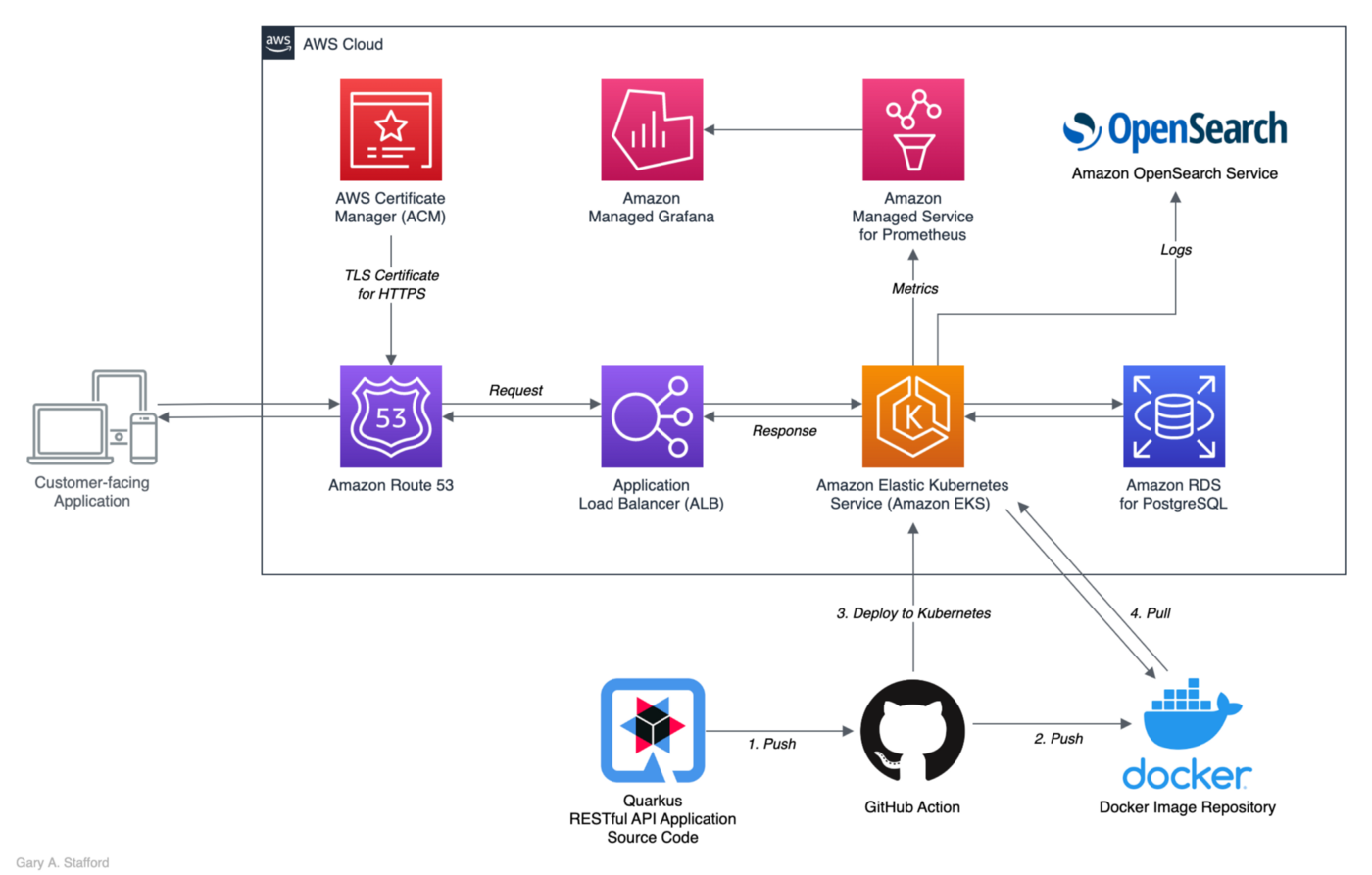
Some of the features of the Quarkus application in this post include:
- Hibernate Object Relational Mapper (ORM), the de facto Jakarta Persistence API (formerly Java Persistence API) implementation
- Hibernate Reactive with Panache, a Quarkus-specific library that simplifies the development of Hibernate Reactive entities
- RESTEasy Reactive, a new JAX-RS implementation, works with the common Vert.x layer and is thus fully reactive
- Advanced RESTEasy Reactive Jackson support for JSON serialization
- Reactive PostgreSQL client
- Built on Mandrel, a downstream distribution of the GraalVM community edition
- Built with Gradle, the modern, open-source build automation tool focused on flexibility and performance
TL;DR
Do you want to explore the source code for this post’s Quarkus microservice application or deploy it to Kubernetes before reading the full article? All the source code and Kubernetes resources are open-source and available on GitHub:
git clone --depth 1 -b main \
https://github.com/garystafford/tickit-srv.git
The latest Docker Image is available on docker.io:
docker pull garystafford/tickit-srv:<latest-tag>
Quarkus Projects with IntelliJ IDE
Although not a requirement, I used JetBrains IntelliJ IDEA 2022 (Ultimate Edition) to develop and test the post’s Quarkus application. Bootstrapping Quarkus projects with IntelliJ is easy. Using the Quarkus plugin bundled with the Ultimate edition, developers can quickly create a Quarkus project.

The Quarkus plugin’s project creation wizard is based on code.quarkus.io. If you have bootstrapped a Spring Initializr project, code.quarkus.io works very similar to start.spring.io.

Visual Studio Code
RedHat also provides a Quarkus extension for the popular Visual Studio Code IDE.

Gradle
This post uses Gradle instead of Maven to develop, test, build, package, and deploy the Quarkus application to Kubernetes. Based on the packages selected in the new project setup shown above, the Quarkus plugin’s project creation wizard creates the following build.gradle
file (Lombak added separately).
The wizard also created the following gradle.properties
file, which has been updated to the latest release of Quarkus available at the time of this post, 2.9.2.
Gradle and Quarkus
You can use the Quarkus CLI or the Quarkus Maven plugin to scaffold a Gradle project. Taking a dependency on the Quarkus plugin adds several additional Quarkus tasks to Gradle. We will use Gradle to develop, test, build, containerize, and deploy the Quarkus microservice application to Kubernetes. The quarkusDev
, quarkusTest
, and quarkusBuild
tasks will be particularly useful in this post.

Java Compilation
The Quarkus application in this post is compiled as a native image with the most recent Java 17 version of Mandrel, a downstream distribution of the GraalVM community edition.
GraalVM and Native Image
According to the documentation, GraalVM is a high-performance JDK distribution. It is designed to accelerate the execution of applications written in Java and other JVM languages while also providing runtimes for JavaScript, Ruby, Python, and other popular languages.
Further, according to GraalVM, Native Image is a technology to ahead-of-time compile Java code to a stand-alone executable, called a native image. This executable includes the application classes, classes from its dependencies, runtime library classes, and statically linked native code from the JDK. The Native Image builder (native-image
) is a utility that processes all classes of an application and their dependencies, including those from the JDK. It statically analyzes data to determine which classes and methods are reachable during the application execution.
Mandrel
Mandrel is a downstream distribution of the GraalVM community edition. Mandrel’s main goal is to provide a native-image
release specifically to support Quarkus. The aim is to align the native-image
capabilities from GraalVM with OpenJDK and Red Hat Enterprise Linux libraries to improve maintainability for native Quarkus applications. Mandrel can best be described as a distribution of a regular OpenJDK with a specially packaged GraalVM Native Image builder (native-image
).
Docker Image
Once complied, the native Quarkus executable will run within the quarkus-micro-image:1.0
base runtime image deployed to Kubernetes. Quarkus provides this base image to ease the containerization of native executables. It has a minimal footprint (10.9 compressed/29.5 MB uncompressed) compared to other images. For example, the latest UBI (Universal Base Image) Quarkus Mandrel image (ubi-quarkus-mandrel:22.1.0.0-Final-java17
) is 714 MB uncompressed, while the OpenJDK 17 image (openjdk:17-jdk
) is 471 MB uncompressed. Even RedHat’s Universal Base Image Minimal image (ubi-minimal:8.6
) is 93.4 MB uncompressed.

An even smaller option from Quarkus is a distroless base image (quarkus-distroless-image:1.0)
is only 9.2 MB compressed / 22.7 MB uncompressed. Quarkus is careful to note that distroless image support is experimental and should not be used in production without rigorous testing.
PostgreSQL Database
For the backend data persistence tier of the Quarkus application, we will use PostgreSQL. All DDL (Data Definition Language) and DML (Data Manipulation Language) statements used in the post were tested with the most current version of PostgreSQL 14.
There are many PostgreSQL-compatible sample databases available that could be used for this post. I am using the TICKIT sample database provided by AWS and designed for Amazon Redshift, AWS’s cloud data warehousing service. The database consists of seven tables — two fact tables and five dimensions tables — in a traditional data warehouse star schema.
For this post, I have remodeled the TICKIT database’s star schema into a normalized relational data model optimized for the Quarkus application. The most significant change to the database is splitting the original Users
dimension table into two separate tables — buyer
and seller
. This change will allow for better separation of concerns (SoC), scalability, and increased protection of Personal Identifiable Information (PII).

Source Code
Each of the six tables in the PostgreSQL TICKIT database is represented by an Entity, Repository, and Resource Java class.

Entity Class
Java Persistence is the API for managing persistence and object/relational mapping. The Java Persistence API (JPA) provides Java developers with an object/relational mapping facility for managing relational data in Java applications. Each table in the PostgreSQL TICKIT database is represented by a Java Persistence Entity, as indicated by the Entity
annotation on the class declaration. The annotation specifies that the class is an entity.

Each entity class extends the PanacheEntityBase
class, part of the io.quarkus.hibernate.orm.panache
package. According to the Quarkus documentation, You can specify your own custom ID strategy, which is done in this post’s example, by extending PanacheEntityBase
instead of PanacheEntity
.
If you do not want to bother defining getters/setters for your entities, which we did not in the post’s example, extending PanacheEntityBase
, Quarkus will generate them for you. Alternately, extend PanacheEntity
and take advantage of the default ID it provides if you are not using a custom ID strategy.
The example SaleEntity
class shown below is typical of the Quarkus application’s entities. The entity class contains several additional JPA annotations in addition to Entity
, including Table
, NamedQueries
, Id
, SequenceGenerator
, GeneratedValue
, and Column
. The entity class also leverages Project Lombok annotations. Lombok generates two boilerplate constructors, one that takes no arguments (NoArgsConstructor
) and one that takes one argument for every field (AllArgsConstructor
).
The SaleEntity
class also defines two many-to-one relationships, with the ListingEntity
and BuyerEntity
entity classes. This relationship mirrors the database’s data model, as reflected in the schema diagram above. The relationships are defined using the ManyToOne
and JoinColumn
JPA annotations.
Given the relationships between the entities, a saleEntity
object, represented as a nested JSON object, would look as follows:
Repository Class
Each table in the PostgreSQL TICKIT database also has a corresponding repository class, often referred to as the ‘repository pattern.’ The repository class implements the PanacheRepositoryBase
interface, part of the io.quarkus.hibernate.orm.panache
package. The PanacheRepositoryBase
Java interface represents a Repository for a specific type of Entity. According to the documentation, if you are using repositories and have a custom ID strategy, then you will want to extend PanacheRepositoryBase
instead of PanacheRepository
and specify your ID type as an extra type parameter. Implementing the PanacheRepositoryBase
will give you the same methods on the PanacheEntityBase
.

The repository class allows us to leverage the methods already available through PanacheEntityBase
and add additional custom methods. For example, the repository class contains a custom method listWithPaging
. This method retrieves (GET
) a list of SaleEntity
objects with the added benefit of being able to indicate the page number, page size, sort by field, and sort direction.
Since there is a many-to-one relationship between the SaleEntity
class and the ListingEntity
and BuyerEntity
entity classes, we also have two custom methods that retrieve all SaleEntity
objects by either the BuyerEntity
ID or the EventEntity
ID. These two methods call the SQL queries in the SaleEntity, annotated with the JPA NamedQueries
/NamedQuery
annotations on the class declaration.
SmallRye Mutiny
Each method defined in the repository class returns a SmallRye Mutiny Uni<T>
. According to the website, Mutiny is an intuitive, event-driven Reactive programming library for Java. Mutiny provides a simple but powerful asynchronous development model that lets you build reactive applications. Mutiny can be used in any Java application exhibiting asynchrony, including reactive microservices, data streaming, event processing, API gateways, and network utilities.
Uni
Again, according to Mutiny’s documentation, a Uni
represents a stream that can only emit either an item or a failure event. A Uni<T>
is a specialized stream that emits only an item or a failure. Typically, Uni<T>
are great for representing asynchronous actions such as a remote procedure call, an HTTP request, or an operation producing a single result. A Uni
represents a lazy asynchronous action. It follows the subscription pattern, meaning that the action is only triggered once a UniSubscriber
subscribes to the Uni
.
Resource Class
Lastly, each table in the PostgreSQL TICKIT database has a corresponding resource class. According to the Quarkus documentation, all the operations defined within PanacheEntityBase
are available on your repository, so using it is exactly the same as using the active record pattern, except you need to inject it. We inject the corresponding repository class into the resource class, exposing all the available methods of the repository and PanacheRepositoryBase
. For example, note the custom listWithPaging
method below, which was declared in the SaleRepository
class.

Similar to the repository class, each method defined in the resource class also returns a SmallRye Mutiny (io.smallrye.mutiny
) Uni<T>
.
The repository defines HTTP methods (POST
, GET
, PUT
, and DELETE
) corresponding to CRUD operations on the database (Create, Read, Update, and Delete). The methods are annotated with the corresponding javax.ws.rs
annotation, indicating the type of HTTP request they respond to. The javax.ws.rs
package contains high-level interfaces and annotations used to create RESTful service resources, such as our Quarkus application.
The POST
, PUT
, and DELETE
annotated methods all have the io.quarkus.hibernate.reactive.panache.common.runtime
package’s ReactiveTransactional
annotation associated with them. We use this annotation on methods to run them in a reactive Mutiny.Session.Transation
. If the annotated method returns a Uni
, which they do, this has precisely the same behavior as if the method was enclosed in a call to Mutiny.Session.withTransaction(java.util.function.Function)
. If the method call fails, the complete transaction is rolled back.
Developer Experience
Quarkus has several features to enhance the developer experience. Features include Dev Services, Dev UI, live reload of code without requiring a rebuild and restart of the application, continuous testing where tests run immediately after code changes have been saved, configuration profiles, Hibernate ORM, JUnit, and REST Assured integrations. Using these Quarkus features, it’s easy to develop and test Quarkus applications.
Configuration Profiles
Similar to Spring, Quarkus works with configuration profiles. According to RedHat, you can use different configuration profiles depending on your environment. Configuration profiles enable you to have multiple configurations in the same application.properties
file and select between them using a profile name. Quarkus recognizes three default profiles:
- dev: Activated in development mode
- test: Activated when running tests
- prod: The default profile when not running in development or test mode
In the application.properties
file, the profile is prefixed using %environment.
format. For example, when defining Quarkus’ log level as INFO
, you add the common quarkus.log.level=INFO
property. However, to change only the test environment’s log level to DEBUG
, corresponding to the test
profile, you would add a property with the %test.
prefix, such as %test.quarkus.log.level=DEBUG
.
Dev Services
Quarkus supports the automatic provisioning of unconfigured services in development and test mode, referred to as Dev Services. If you include an extension and do not configure it, then Quarkus will automatically start the relevant service using Test containers behind the scenes and wire up your application to use this service.
When developing your Quarkus application, you could create your own local PostgreSQL database, for example, with Docker:
And the corresponding application configuration properties:
Zero-Config Database
Alternately, we can rely on Dev Services, using a feature referred to as zero config setup. Quarkus provides you with a zero-config database out of the box; no database configuration is required. Quarkus takes care of provisioning the database, running your DDL and DML statements to create database objects and populate the database with test data, and finally, de-provisioning the database container when the development or test session is completed. The database Dev Services will be enabled when a reactive or JDBC datasource extension is present in the application and the database URL has not been configured.
Using the quarkusDev
Gradle task, we can start the application running, as shown in the video below. Note the two new Docker containers that are created. Also, note the project’s import.sql
SQL script is run automatically, executing all DDL and DML statements to prepare and populate the database.
Bootstrapping the TICKIT Database
When using Hibernate ORM with Quarkus, we have several options regarding how the database is handled when the Quarkus application starts. These are defined in the application.properties file. The quarkus.hibernate-orm.database.generation
property determines whether the database schema is generated or not. drop-and-create
is ideal in development mode, as shown above. This property defaults to none
, however, if Dev Services is in use and no other extensions that manage the schema are present, this will default to drop-and-create
. Accepted values: none
, create
, drop-and-create
, drop
, update
, validate
. For development and testing modes, we are using Dev Services with the default value of drop-and-create
. For this post, we assume the database and schema already exist in production.
A second property, quarkus.hibernate-orm.sql-load-script
, provides the path to a file containing the SQL statements to execute when Hibernate ORM starts. In dev and test modes, it defaults to import.sql
. Simply add an import.sql
file in the root of your resources directory, Hibernate will be picked up without having to set this property. The project contains an import.sql
script to create all database objects and a small amount of test data. You can also explicitly set different files for different profiles and prefix the property with the profile (e.g., %dev.
or %test.
).
%dev.quarkus.hibernate-orm.database.generation=drop-and-create
%dev.quarkus.hibernate-orm.sql-load-script=import.sql
Another option is Flyway, the popular database migration tool commonly used in JVM environments. Quarkus provides first-class support for using Flyway.
Dev UI
According to the documentation, Quarkus now ships with a new experimental Dev UI, which is available in dev mode (when you start Quarkus with Gradle’s quarkusDev task) at /q/dev
by default. It allows you to quickly visualize all the extensions currently loaded, see their status and go directly to their documentation. In addition to access to loaded extensions, you can review logs and run tests in the Dev UI.

Configuration
From the Dev UI, you can access and modify the Quarkus application’s application configuration.

You also can view the configuration of Dev Services, including the running containers and no-config database config.

Quarkus REST Score Console
With RESTEasy Reactive extension loaded, you can access the Quarkus REST Score Console from the Dev UI. The REST Score Console shows endpoint performance through scores and color-coding: green, yellow, or red. RedHat published a recent blog that talks about the scoring process and how to optimize the performance endpoints. Three measurements show whether a REST reactive application can be optimized further.

Application Testing
Quarkus enables robust JVM-based and Native continuous testing by providing integrations with common test frameworks, such as including JUnit, Mockito, and REST Assured. Many of Quarkus’ testing features are enabled through annotations, such as QuarkusTestResource
, QuarkusTest
, QuarkusIntegrationTest
, and TransactionalQuarkusTest
.
Quarkus supports the use of mock objects using two different approaches. You can either use CDI alternatives to mock out a bean for all test classes or use QuarkusMock
to mock out beans on a per-test basis. This includes integration with Mockito.
The REST Assured integration is particularly useful for testing the Quarkus microservice API application. According to their website, REST Assured is a Java DSL for simplifying testing of REST-based services. It supports the most common HTTP request methods and can be used to validate and verify the response of these requests. REST Assured uses the given()
, when()
, then()
methods of testing made popular as part of Behavior-Driven Development (BDD).
The tests can be run using the the quarkusTest
Gradle task. The application contains a small number of integration tests to demonstrate this feature.

Swagger and OpenAPI
Quarkus provides the Smallrye OpenAPI extension compliant with the MicroProfile OpenAPI specification, which allows you to generate an API OpenAPI v3 specification and expose the Swagger UI. The /q/swagger-ui
resource exposes the Swagger UI, allowing you to visualize and interact with the Quarkus API’s resources without having any implementation logic in place.

Resources can be tested using the Swagger UI without writing any code.

OpenAPI Specification (formerly Swagger Specification) is an API description format for REST APIs. The /q/openapi
resource allows you to generate an OpenAPI v3 specification file. An OpenAPI file allows you to describe your entire API.

The OpenAPI v3 specification can be saved as a file and imported into applications like Postman, the API platform for building and using APIs.


GitOps with GitHub Actions
For this post, GitOps is used to continuously test, build, package, and deploy the Quarkus microservice application to Kubernetes. Specifically, the post uses GitHub Actions. GitHub Actions is a continuous integration and continuous delivery (CI/CD) platform that allows you to automate your build, test, and deployment pipelines. Workflows are defined in the .github/workflows
directory in a repository, and a repository can have multiple workflows, each of which can perform a different set of tasks.

Two GitHub Actions are associated with this post’s GitHub repository. The first action, build-test.yml
, natively builds and tests the source code in a native Mandrel container on each push to GitHub. The second action (shown below), docker-build-push.yml
, builds and containerizes the natively-built executable, pushes it to Docker’s Container Registry (docker.io), and finally deploys the application to Kubernetes. This action is triggered by pushing a new Git Tag to GitHub.

There are several Quarkus configuration properties included in the action’s build step. Alternately, these properties could be defined in the application.properties
file. However, I have decided to include them as part of the Gradle build task since they are specific to the type of build and container registry and Kubernetes platform I am pushing to artifacts.
Kubernetes Resources
The Kubernetes resources YAML file, created by the Quarkus build, is also uploaded and saved as an artifact in GitHub by the final step in the GitHub Action.

Quarkus automatically generates ServiceAccount
, Role
, RoleBinding
, Service
, Deployment
resources.
Choosing a Kubernetes Platform
The only cloud provider-specific code is in the second GitHub action.
In this case, the application is being deployed to an existing Amazon Elastic Kubernetes Service (Amazon EKS), a fully managed, certified Kubernetes conformant service from AWS. These steps can be easily replaced with steps to deploy to other Cloud platforms, such as Microsoft’s Azure Kubernetes Service (AKS) or Google Cloud’s Google Kubernetes Engine (GKE).
GitHub Secrets
Some of the properties use GitHub environment variables, and others use secure GitHub repository encrypted secrets. Secrets are used to secure Docker credentials used to push the Quarkus application image to Docker’s image repository, AWS IAM credentials, and the base64 encoded contents of the kubeconfig
file required to deploy to Kubernetes on AWS when using the kodermax/kubectl-aws-eks@master
GitHub action.

Docker
Reviewing the configuration properties included in the action’s build step, note the Mandrel container used to build the native Quarkus application, quay.io/quarkus/ubi-quarkus-mandrel:22.1.0.0-Final-java17
. Also, note the project’s Docker file is used to build the final Docker image, pushed to the image repository, and then used to provision containers on Kubernetes, src/main/docker/Dockerfile.native-micro
. This Dockerfile uses the quay.io/quarkus/quarkus-micro-image:1.0
base image to containerize the native Quarkus application.
The properties also define the image’s repository name and tag (e.g., garystafford/tickit-srv:1.1.0
).


Kubernetes
In addition to creating the ticket
Namespace in advance, a Kubernetes secret is pre-deployed to the ticket
Namespace. The GitHub Action also requires a Role and RoleBinding to deploy the workload to the Kubernetes cluster. Lastly, a HorizontalPodAutoscaler (HPA) is used to automatically scale the workload.
export NAMESPACE=tickit# Namespace
kubectl create namespace ${NAMESPACE}# Role and RoleBinding for GitHub Actions to deploy to Amazon EKS
kubectl apply -f kubernetes/github_actions_role.yml -n ${NAMESPACE}# Secret
kubectl apply -f kubernetes/secret.yml -n ${NAMESPACE}# HorizontalPodAutoscaler (HPA)
kubectl apply -f kubernetes/tickit-srv-hpa.yml -n ${NAMESPACE}
As part of the configuration properties included in the action’s build step, note the use of Kubernetes secrets.
-Dquarkus.kubernetes-config.secrets=tickit
-Dquarkus.kubernetes-config.secrets.enabled=true
This secret contains base64 encoded sensitive credentials and connection values to connect to the Production PostgreSQL database. For this post, I have pre-built an Amazon RDS for PostgreSQL database instance, created the ticket database and required database objects, and lastly, imported the sample data included in the GitHub repository, garystafford/tickit-srv-data
.
The five keys seen in the Secret are used in the application.properties
file to provide access to the Production PostgreSQL database from the Quakus application.
An even better alternative to using Kubernetes secrets on Amazon EKS is AWS Secrets and Configuration Provider (ASCP) for the Kubernetes Secrets Store CSI Driver. AWS Secrets Manager stores secrets as files mounted in Amazon EKS pods.
AWS Architecture
The GitHub Action pushes the application’s image to Docker’s Container Registry (docker.io), then deploys the application to Kubernetes. Alternately, you could use AWS’s Amazon Elastic Container Registry (Amazon ECR). Amazon EKS pulls the image from Docker as it creates the Kubernetes Pod containers.
There are many ways to route traffic from a requestor to the Quarkus application running on Kubernetes. For this post, the Quarkus application is exposed as a Kubernetes Service on a NodePort. For this post, I have registered a domain, example-api.com
, with Amazon Route 53 and a corresponding TLS certificate with AWS Certificate Manager. Inbound requests to the Quarkus application are directed to a subdomain, ticket.example-api.com
using HTTPS or port 443. Amazon Route 53 routes those requests to a Layer 7 application load balancer (ALB). The ALB then routes those requests to the Amazon EKS Kubernetes cluster on the NodePort using simple round-robin load balancing. Requests will be routed automatically by Kubernetes to the appropriate worker node and Kubernetes pod. The response then traverses a similar path back to the requestor.
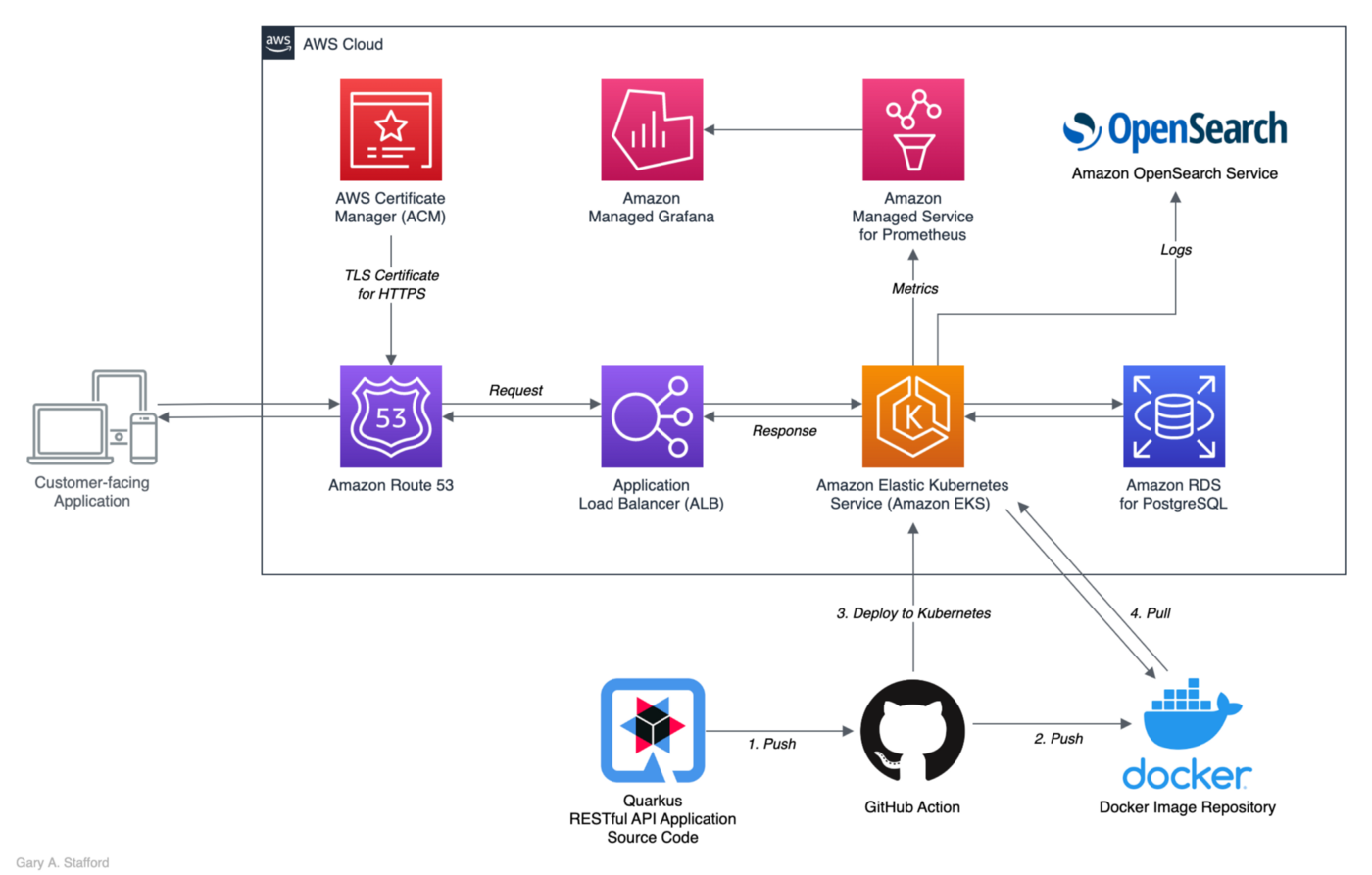
Results
If the GitHub action is successful, any push of code changes to GitHub results in the deployment of the application to Kubernetes.

We can also view the deployed Quarkus application resources using the Kubernetes Dashboard.

Metrics
The post’s Quarkus application implements the micrometer-registry-prometheus
extension. The Micrometer metrics library exposes runtime and application metrics. Micrometer defines a core library, providing a registration mechanism for metrics and core metric types.

Using the Micrometer extension, a metrics resource is exposed at /q/metrics
, which can be scraped and visualized by tools such as Prometheus. AWS offers its fully-managed Amazon Managed Service for Prometheus (AMP), which easily integrates with Amazon EKS.

Using Prometheus as a datasource, we can build dashboards in Grafana to observe the Quarkus Application metrics. Similar to AMP, AWS offers its fully managed Amazon Managed Grafana (AMG).

Centralized Log Management
According to Quarkus documentation, internally, Quarkus uses JBoss Log Manager and the JBoss Logging facade. You can use the JBoss Logging facade inside your code or any of the supported Logging APIs, including JDK java.util.logging
(aka JUL), JBoss Logging, SLF4J, and Apache Commons Logging. Quarkus will send them to JBoss Log Manager.
There are many ways to centralize logs. For example, you can send these logs to open-source centralized log management systems like Graylog, Elastic Stack, fka ELK (Elasticsearch, Logstash, Kibana), EFK (Elasticsearch, Fluentd, Kibana), and OpenSearch with Fluent Bit.
If you are using Kubernetes, the simplest way is to send logs to the console and integrate a central log manager inside your cluster. Since the Quarkus application in this post is running on Amazon EKS, I have chosen Amazon OpenSearch Service with Fluent Bit, an open-source and multi-platform Log Processor and Forwarder. Fluent Bit is fully compatible with Docker and Kubernetes environments. Amazon provides an excellent workshop on installing and configuring Amazon OpenSearch Service with Fluent Bit.


Conclusion
As we learned in this post, Quarkus, the ‘Supersonic Subatomic Java’ framework, is a cloud-native, Kubernetes-native, container first, microservices first framework for writing Java applications. We observed how to build, test, and deploy a RESTful Quarkus Native application to Kubernetes.
Quarkus has capabilities and features well beyond this post’s scope. In a future post, we will explore other abilities of Quarkus, including observability, GraphQL integration, caching, database proxying, tracing and debugging, message queues, data pipelines, and streaming analytics.
This blog represents my own viewpoints and not of my employer, Amazon Web Services (AWS). All product names, logos, and brands are the property of their respective owners. All diagrams and illustrations are property of the author.
End-to-End Data Discovery, Observability, and Governance on AWS with LinkedIn’s Open-source DataHub
Posted by Gary A. Stafford in Analytics, AWS, Azure, Bash Scripting, Build Automation, Cloud, DevOps, GCP, Kubernetes, Python, Software Development, SQL, Technology Consulting on March 26, 2022
Use DataHub’s data catalog capabilities to collect, organize, enrich, and search for metadata across multiple platforms
Introduction
According to Shirshanka Das, Founder of LinkedIn DataHub, Apache Gobblin, and Acryl Data, one of the simplest definitions for a data catalog can be found on the Oracle website: “Simply put, a data catalog is an organized inventory of data assets in the organization. It uses metadata to help organizations manage their data. It also helps data professionals collect, organize, access, and enrich metadata to support data discovery and governance.”
Another succinct description of a data catalog’s purpose comes from Alation: “a collection of metadata, combined with data management and search tools, that helps analysts and other data users to find the data that they need, serves as an inventory of available data, and provides information to evaluate the fitness of data for intended uses.”
Working with many organizations in the area of Analytics, one of the more common requests I receive regards choosing and implementing a data catalog. Organizations have datasources hosted in corporate data centers, on AWS, by SaaS providers, and with other Cloud Service Providers. Several of these organizations have recently gravitated to DataHub, the open-source metadata platform for the modern data stack, originally developed by LinkedIn.

In this post, we will explore the capabilities of DataHub to build a centralized data catalog on AWS for datasources hosted in multiple AWS accounts, SaaS providers, cloud service providers, and corporate data centers. I will demonstrate how to build a DataHub data catalog using out-of-the-box data source plugins for automated metadata ingestion.
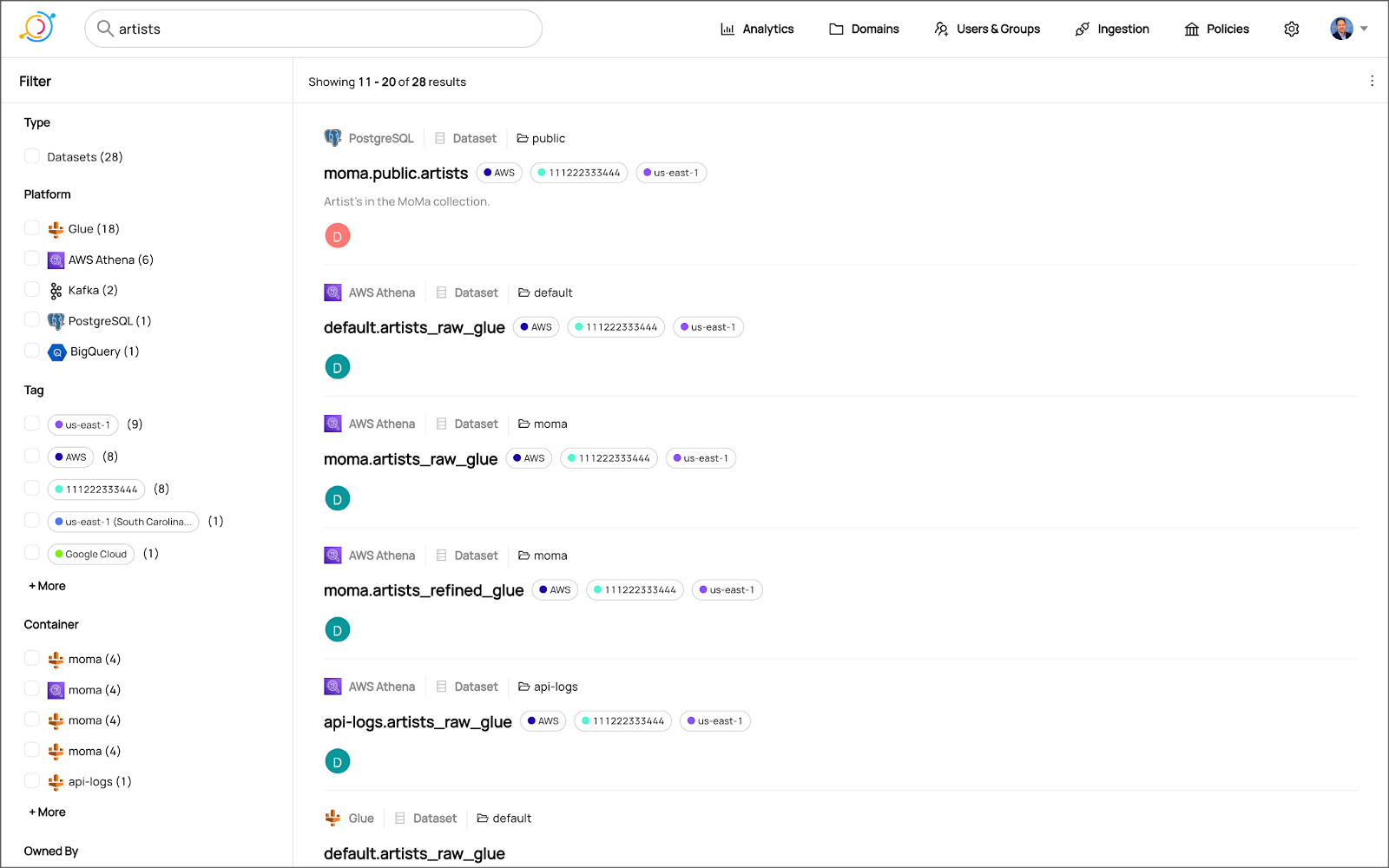
Data Catalog Competitors
Data catalogs are not new; technologies such as data dictionaries have been around as far back as the 1980’s. Gartner publishes their Metadata Management (EMM) Solutions Reviews and Ratings and Metadata Management Magic Quadrant. These reports contain a comprehensive list of traditional commercial enterprise players, modern cloud-native SaaS vendors, and Cloud Service Provider (CSP) offerings. DBMS Tools also hosts a comprehensive list of 30 data catalogs. A sampling of current data catalogs includes:
Open Source Software
Commercial
- Acryl Data (based on LinkedIn’s DataHub)
- Atlan
- Stemma (based on Lyft’s Amundsen)
- Talend
- Alation
- Collibra
- data.world
Cloud Service Providers
Data Catalog Features
DataHub describes itself as “a modern data catalog built to enable end-to-end data discovery, data observability, and data governance.” Sorting through vendor’s marketing jargon and hype, standard features of leading data catalogs include:
- Metadata ingestion
- Data discovery
- Data governance
- Data observability
- Data lineage
- Data dictionary
- Data classification
- Usage/popularity statistics
- Sensitive data handling
- Data fitness (aka data quality or data profiling)
- Manage both technical and business metadata
- Business glossary
- Tagging
- Natively supported datasource integrations
- Advanced metadata search
- Fine-grain authentication and authorization
- UI- and API-based interaction
Datasources
When considering a data catalog solution, in my experience, the most common datasources that customers want to discover, inventory, and search include:
- Relational databases and other OLTP datasources such as PostgreSQL, MySQL, Microsoft SQL Server, and Oracle
- Cloud Data Warehouses and other OLAP datasources such as Amazon Redshift, Snowflake, and Google BigQuery
- NoSQL datasources such as MongoDB, MongoDB Atlas, and Azure Cosmos DB
- Persistent event-streaming platforms such as Apache Kafka (Amazon MSK and Confluent)
- Distributed storage datasets (e.g., Data Lakes) such as Amazon S3, Apache Hive, and AWS Glue Data Catalogs
- Business Intelligence (BI), dashboards, and data visualization sources such as Looker, Tableau, and Microsoft Power BI
- ETL sources, such as Apache Spark, Apache Airflow, Apache NiFi, and dbt
DataHub on AWS
DataHub’s convenient AWS setup guide covers options to deploy DataHub to AWS. For this post, I have hosted DataHub on Kubernetes, using Amazon Elastic Kubernetes Service (Amazon EKS). Alternately, you could choose Google Kubernetes Engine (GKE) on Google Cloud or Azure Kubernetes Service (AKS) on Microsoft Azure.
Conveniently, DataHub offers a Helm chart, making deployment to Kubernetes straightforward. Furthermore, Helm charts are easily integrated with popular CI/CD tools. For this post, I’ve used ArgoCD, the declarative GitOps continuous delivery tool for Kubernetes, to deploy the DataHub Helm charts to Amazon EKS.

According to the documentation, DataHub consists of four main components: GMS, MAE Consumer (optional), MCE Consumer (optional), and Frontend. Kubernetes deployment for each of the components is defined as sub-charts under the main DataHub Helm chart.
External Storage Layer Dependencies
Four external storage layer dependencies power the main DataHub components: Kafka, Local DB (MySQL, Postgres, or MariaDB), Search Index (Elasticsearch), and Graph Index (Neo4j or Elasticsearch). DataHub has provided a separate DataHub Prerequisites Helm chart for the dependencies. The dependencies must be deployed before deploying DataHub.
Alternately, you can substitute AWS managed services for the external storage layer dependencies, which is also detailed in the Deploying to AWS documentation. AWS managed service dependency substitutions include Amazon RDS for MySQL, Amazon OpenSearch (fka Amazon Elasticsearch), and Amazon Managed Streaming for Apache Kafka (Amazon MSK). According to DataHub, support for using AWS Neptune as the Graph Index is coming soon.
DataHub CLI and Plug-ins
DataHub comes with the datahub
CLI, allowing you to perform many common operations on the command line. You can install and use the DataHub CLI within your development environment or integrate it with your CI/CD tooling.

DataHub uses a plugin architecture. Plugins allow you to install only the datasource dependencies you need. For example, if you want to ingest metadata from Amazon Athena, just install the Athena plugin: pip install 'acryl-datahub[athena]'
. DataHub Source, Sink, and Transformer plugins can be displayed using the datahub check plugins
CLI command.


Secure Metadata Ingestion
Often, datasources are not externally accessible for security reasons. Further, many datasources may not be accessible to individual users, especially in higher environments like UAT, Staging, and Production. They are only accessible to applications or CI/CD tooling. To overcome these limitations when extracting metadata with DataHub, I prefer to perform my DataHub-related development and testing locally but execute all DataHub ingestion securely on AWS.
In my local development environment, I use JetBrains PyCharm to author the Python and YAML-based DataHub configuration files and ingestion pipeline recipes, then commit those files to git and push them to a private GitHub repository. Finally, I use GitHub Actions to test DataHub files.
To run DataHub ingestion jobs and push the results to DataHub running in Kubernetes on Amazon EKS, I have built a custom Python-based Docker container. The container runs the DataHub CLI, required DataHub plugins, and any additional Python dependencies. The container’s pod has the appropriate AWS IAM permissions, using IAM Roles for Service Accounts (IRSA), to securely access datasources to ingest and the DataHub application.
Schedule and Monitor Pipelines
Scheduling and managing multiple metadata ingestion jobs on AWS is best handled with Apache Airflow with Amazon Managed Workflows for Apache Airflow (Amazon MWAA). Ingestion jobs run as Airflow DAG tasks, which call the EKS-based DataHub CLI container. With MWAA, datasource connections, credentials, and other sensitive configurations can be kept secure and not be exposed externally or in plain text.
When running the ingestion pipelines on AWS with DataHub, all communications between AWS-based datasources, ingestion jobs running in Airflow, and DataHub, should use secure private IP addressing and DNS resolution instead of transferring metadata over the Internet. Make sure to create all the necessary VPC peering connections, network route table configurations, and VPC endpoints to connect all relevant services.
SaaS services such as Snowflake or MongoDB Atlas, services provided by other Cloud Service Providers such as Google Cloud and Microsoft Azure, and datasources in corporate datasources require alternate networking and security strategies to access metadata securely.

Markup or Code?
According to the documentation, a DataHub recipe is a configuration file that tells ingestion scripts where to pull data from (source) and where to put it (sink). Recipes normally contain a source
, sink
, and transformers
configuration section. Mark-up language-based job automation written in YAML, JSON, or Domain Specific Languages (DSLs) is often an alternative to writing code. DataHub recipes can be written in YAML. The example recipe shown below is used to ingest metadata from an Amazon RDS for PostgreSQL database, running on AWS.
YAML-based recipes can also use automatic environment variable expansion for convenience, automation, and security. It is considered best practice to secure sensitive configuration values, such as database credentials, in a secure location and reference them as environment variables. For example, note the server: ${DATAHUB_REST_ENDPOINT}
entry in the sink
section below. The DATAHUB_REST_ENDPOINT
environment variable is set ahead of time and re-used for all ingestion jobs. Sensitive database connection information has also been variablized and stored separately.
Using Python
You can configure and run a pipeline entirely from within a custom Python script using DataHub’s Python API as an alternative to YAML. Below, we see two nearly identical ingestion recipes to the YAML above, written in Python. Writing ingestion pipeline logic programmatically gives you increased flexibility for automation, error checking, unit-testing, and notification. Below is a basic pipeline written in Python. The code is functional, but not very Pythonic, secure, scalable, or Production ready.
The second version of the same pipeline is more Production ready. The code is more Pythonic in nature and makes use of error checking, logging, and the AWS Systems Manager (SSM) Parameter Store. Like recipes written in YAML, environment variables can be used for convenience and security. In this example, commonly reused and sensitive connection configuration items have been extracted and placed in the SSM Parameter Store. Additional configuration is pulled from the environment, such as AWS Account ID and AWS Region. The script loads these values at runtime.
Sinking to DataHub
When syncing metadata to DataHub, you have two choices, the GMS REST API or Kafka. According to DataHub, the advantage of the REST-based interface is that any errors can immediately be reported. On the other hand, the advantage of the Kafka-based interface is that it is asynchronous and can handle higher throughput. For this post, I am DataHub’s REST API.


Column-level Metadata
In addition to column names and data types, it is possible to extract column descriptions and key types from certain datasources. Column descriptions, tags, and glossary terms can also be input through the DataHub UI. Below, we see an example of an Amazon Redshift fact table, whose table and column descriptions were ingested as part of the metadata.

Business Glossary
DataHub can assign business glossary terms to entities. The DataHub Business Glossary plugin pulls business glossary metadata from a YAML-based configuration file.
Business glossary terms can be reviewed in the Glossary Terms tab of the DataHub’s UI. Below, we see the three terms associated with the Classification
glossary node: Confidential
, HighlyConfidential
, and Sensitive
.

We can search for entities inventoried in DataHub using their assigned business glossary terms.

Finally, we see an example of an AWS Athena data catalog table with business glossary terms applied to columns within the table’s schema.

SQL-based Profiler
DataHub also can extract statistics about entities in DataHub using the SQL-based Profiler. According to the DataHub documentation, the Profiler can extract the following:
- Row and column counts for each table
- Column null counts and proportions
- Column distinct counts and proportions
- Column min, max, mean, median, standard deviation, quantile values
- Column histograms or frequencies of unique values
In addition, we can also track the historical stats for each profiled entity each time metadata is ingested.


Data Lineage
DataHub’s data lineage features allow us to view upstream and downstream relationships between different types of entities. DataHub can trace lineage across multiple platforms, datasets, pipelines, charts, and dashboards.
Below, we see a simple example of dataset entity-to-entity lineage in Amazon Redshift and then Apache Spark on Amazon EMR. The fact table has a downstream relationship to four database views. The views are based on SQL queries that include the upstream table as a datasource.


DataHub Analytics
DataHub provides basic metadata quality and usage analytics in the DataHub UI: user activity, counts of datasource types, business glossary terms, environments, and actions.


Conclusion
In this post, we explored the features of a data catalog and learned about some of the leading commercial and open-source data catalogs. Next, we learned how DataHub could collect, organize, enrich, and search metadata across multiple datasources. Lastly, we discovered how easy it is to catalog metadata from datasources spread across multiple CSP, SaaS providers, and corporate data centers, and centralize those results in DataHub.
In addition to the basic features reviewed in this post, DataHub offers a growing number of additional capabilities, including GraphQL and Timeline APIs, robust authentication and authorization, application monitoring observability, and Great Expectations integration. All these qualities make DataHub an excellent choice for a data catalog.
This blog represents my own viewpoints and not of my employer, Amazon Web Services (AWS). All product names, logos, and brands are the property of their respective owners.
Data Preparation on AWS: Comparing Available ELT Options to Cleanse and Normalize Data
Posted by Gary A. Stafford in Analytics, AWS, Build Automation, Cloud, Python, SQL, Technology Consulting on March 1, 2022
Comparing the features and performance of different AWS analytics services for Extract, Load, Transform (ELT)
Introduction
According to Wikipedia, “Extract, load, transform (ELT) is an alternative to extract, transform, load (ETL) used with data lake implementations. In contrast to ETL, in ELT models the data is not transformed on entry to the data lake but stored in its original raw format. This enables faster loading times. However, ELT requires sufficient processing power within the data processing engine to carry out the transformation on demand, to return the results in a timely manner.”
As capital investments and customer demand continue to drive the growth of the cloud-based analytics market, the choice of tools seems endless, and that can be a problem. Customers face a constant barrage of commercial and open-source tools for their batch, streaming, and interactive exploratory data analytics needs. The major Cloud Service Providers (CSPs) have even grown to a point where they now offer multiple services to accomplish similar analytics tasks.
This post will examine the choice of analytics services available on AWS capable of performing ELT. Specifically, this post will compare the features and performance of AWS Glue Studio, Amazon Glue DataBrew, Amazon Athena, and Amazon EMR using multiple ELT use cases and service configurations.
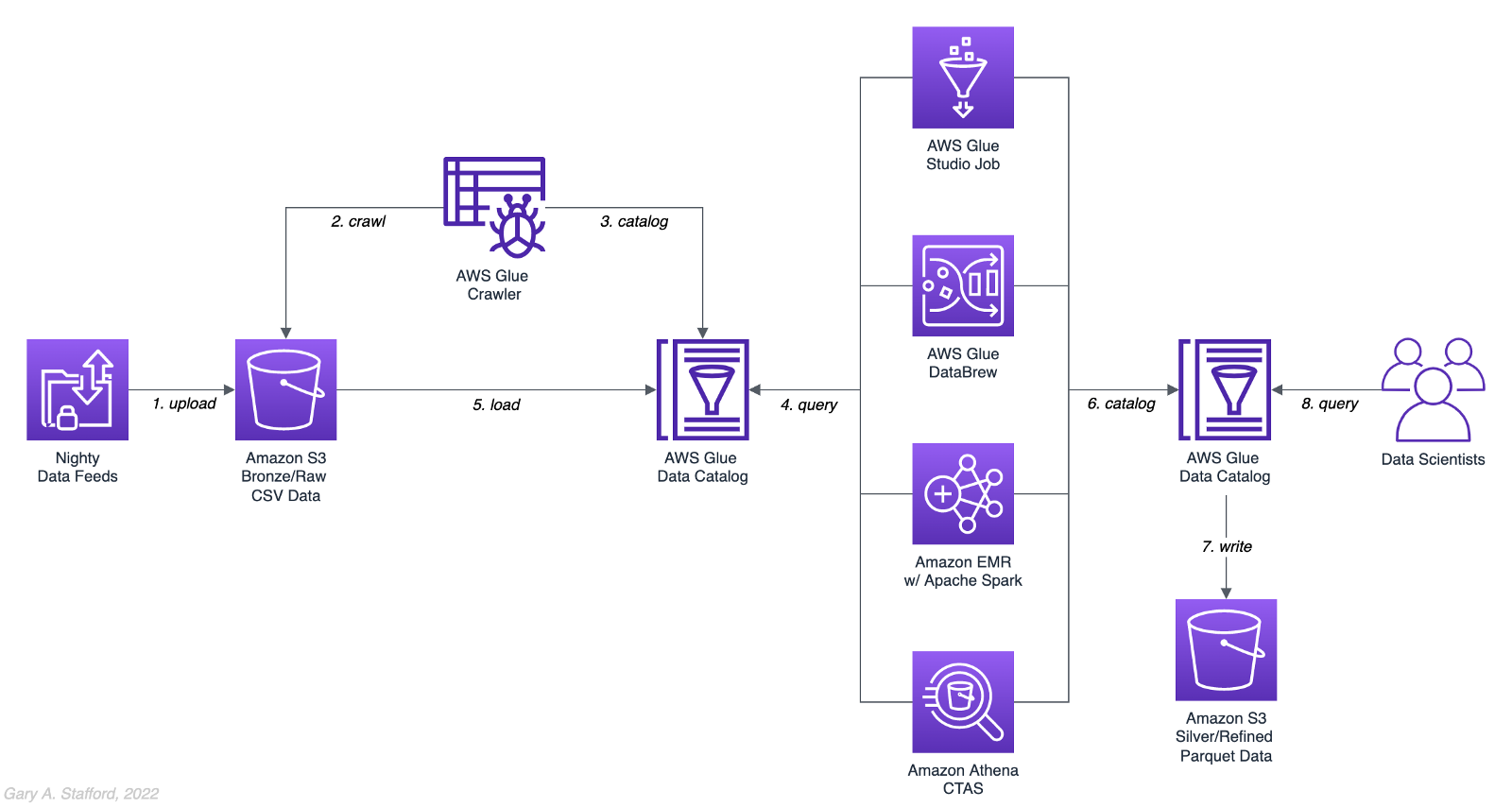
Analytics Use Case
We will address a simple yet common analytics challenge for this comparison — preparing a nightly data feed for analysis the next day. Each night a batch of approximately 1.2 GB of raw CSV-format healthcare data will be exported from a Patient Administration System (PAS) and uploaded to Amazon S3. The data must be cleansed, deduplicated, refined, normalized, and made available to the Data Science team the following morning. The team of Data Scientists will perform complex data analytics on the data and build machine learning models designed for early disease detection and prevention.
Sample Dataset
The dataset used for this comparison is generated by Synthea, an open-source patient population simulation. The high-quality, synthetic, realistic patient data and associated health records cover every aspect of healthcare. The dataset contains the patient-related healthcare history for allergies, care plans, conditions, devices, encounters, imaging studies, immunizations, medications, observations, organizations, patients, payers, procedures, providers, and supplies.
The Synthea dataset was first introduced in my March 2021 post examining the handling of sensitive PII data using Amazon Macie: Data Lakes: Discovery, Security, and Privacy of Sensitive Data.
The Synthea synthetic patient data is available in different record volumes and various data formats, including HL7 FHIR, C-CDA, and CSV. We will use CSV-format data files for this post. Since this post seeks to measure the performance of different AWS ELT-capable services, we will use a larger version of the Synthea dataset containing hundreds of thousands to millions of records.
AWS Glue Data Catalog
The dataset comprises nine uncompressed CSV files uploaded to Amazon S3 and cataloged to an AWS Glue Data Catalog, a persistent metadata store, using an AWS Glue Crawler.

Test Cases
We will use three data preparation test cases based on the Synthea dataset to examine the different AWS ELT-capable services.

Test Case 1: Encounters for Symptom
An encounter is a health care contact between the patient and the provider responsible for diagnosing and treating the patient. In our first test case, we will process 1.26M encounters records for an ongoing study of patient symptoms by our Data Science team.
Data preparation includes the following steps:
- Load 1.26M encounter records using the existing AWS Glue Data Catalog table.
- Remove any duplicate records.
- Select only the records where the
description
column contains “Encounter for symptom.” - Remove any rows with an empty
reasoncodes
column. - Extract a new
year
,month
, andday
column from thedate
column. - Remove the
date
column. - Write resulting dataset back to Amazon S3 as Snappy-compressed Apache Parquet files, partitioned by
year
,month
, andday
. - Given the small resultset, bucket the data such that only one file is written per
day
partition to minimize the impact of too many small files on future query performance. - Catalog resulting dataset to a new table in the existing AWS Glue Data Catalog, including partitions.
Test Case 2: Observations
Clinical observations ensure that treatment plans are up-to-date and correctly administered and allow healthcare staff to carry out timely and regular bedside assessments. We will process 5.38M encounters records for our Data Science team in our second test case.
Data preparation includes the following steps:
- Load 5.38M observation records using the existing AWS Glue Data Catalog table.
- Remove any duplicate records.
- Extract a new
year
,month
, andday
column from the date column. - Remove the
date
column. - Write resulting dataset back to Amazon S3 as Snappy-compressed Apache Parquet files, partitioned by
year
,month
, andday
. - Given the small resultset, bucket the data such that only one file is written per
day
partition to minimize the impact of too many small files on future query performance. - Catalog resulting dataset to a new table in the existing AWS Glue Data Catalog, including partitions.
Test Case 3: Sinusitis Study
A medical condition is a broad term that includes all diseases, lesions, and disorders. In our second test case, we will join the conditions records with the patient records and filter for any condition containing the term ‘sinusitis’ in preparation for our Data Science team.
Data preparation includes the following steps:
- Load 483k condition records using the existing AWS Glue Data Catalog table.
- Inner join the condition records with the 132k patient records based on patient ID.
- Remove any duplicate records.
- Drop approximately 15 unneeded columns.
- Select only the records where the
description
column contains the term “sinusitis.” - Remove any rows with empty
ethnicity
,race
,gender
, ormarital
columns. - Create a new column,
condition_age
, based on a calculation of the age in days at which the patient’s condition was diagnosed. - Write the resulting dataset back to Amazon S3 as Snappy-compressed Apache Parquet-format files. No partitions are necessary.
- Given the small resultset, bucket the data such that only one file is written to minimize the impact of too many small files on future query performance.
- Catalog resulting dataset to a new table in the existing AWS Glue Data Catalog.
AWS ELT Options
There are numerous options on AWS to handle the batch transformation use case described above; a non-exhaustive list includes:
- AWS Glue Studio (UI-driven with AWS Glue PySpark Extensions)
- Amazon Glue DataBrew
- Amazon Athena
- Amazon EMR with Apache Spark
- AWS Glue Studio (Apache Spark script)
- AWS Glue Jobs (Legacy jobs)
- Amazon EMR with Presto
- Amazon EMR with Trino
- Amazon EMR with Hive
- AWS Step Functions and AWS Lambda
- Amazon Redshift Spectrum
- Partner solutions on AWS, such as Databricks, Snowflake, Upsolver, StreamSets, Stitch, and Fivetran
- Self-managed custom solutions using a combination of OSS, such as dbt, Airbyte, Dagster, Meltano, Apache NiFi, Apache Drill, Apache Beam, Pandas, Apache Airflow, and Kubernetes
For this comparison, we will choose the first five options listed above to develop our ELT data preparation pipelines: AWS Glue Studio (UI-driven job creation with AWS Glue PySpark Extensions), Amazon Glue DataBrew, Amazon Athena, Amazon EMR with Apache Spark, and AWS Glue Studio (Apache Spark script).
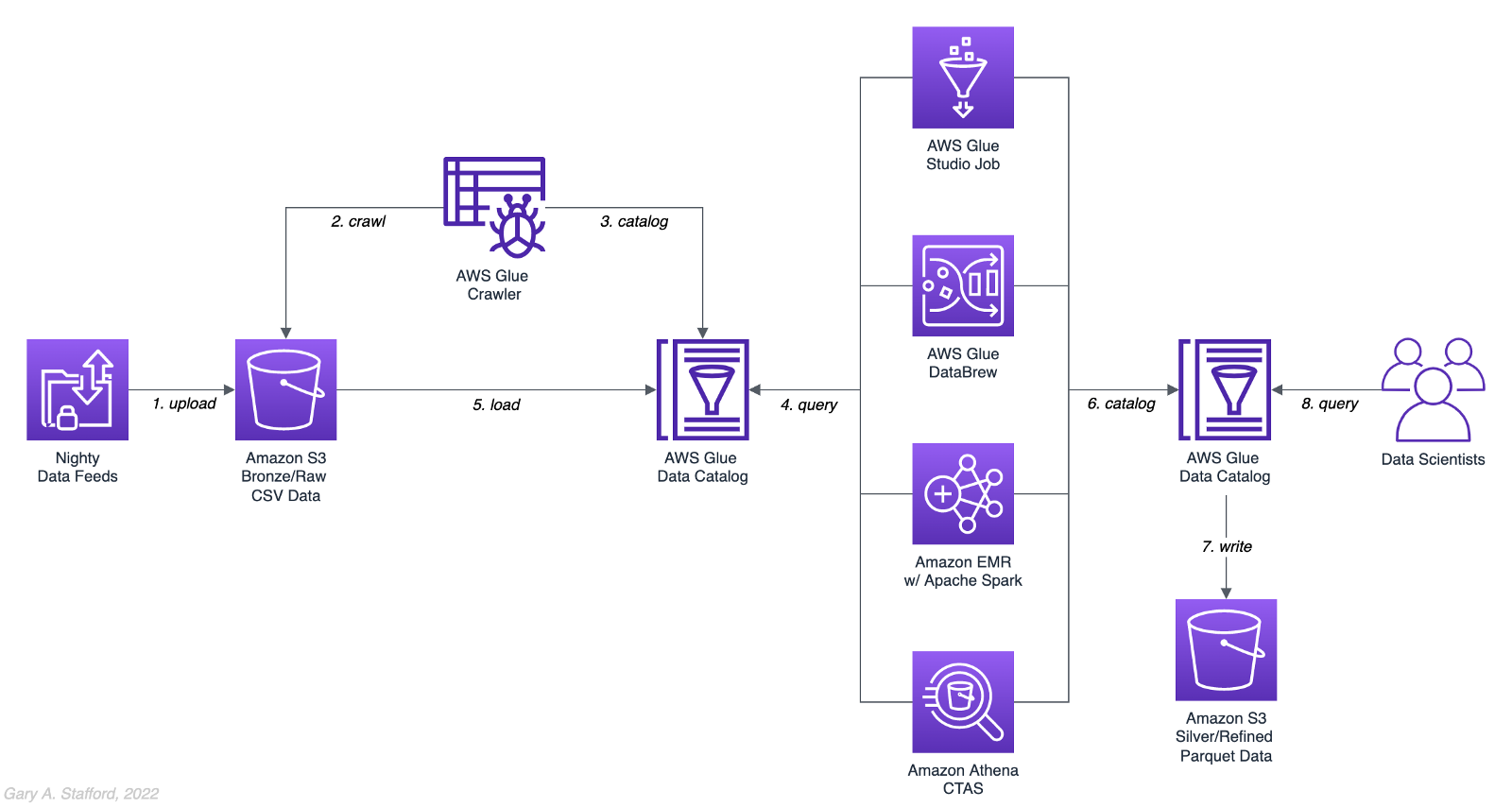
AWS Glue Studio
According to the documentation, “AWS Glue Studio is a new graphical interface that makes it easy to create, run, and monitor extract, transform, and load (ETL) jobs in AWS Glue. You can visually compose data transformation workflows and seamlessly run them on AWS Glue’s Apache Spark-based serverless ETL engine. You can inspect the schema and data results in each step of the job.”
AWS Glue Studio’s visual job creation capability uses the AWS Glue PySpark Extensions, an extension of the PySpark Python dialect for scripting ETL jobs. The extensions provide easier integration with AWS Glue Data Catalog and other AWS-managed data services. As opposed to using the graphical interface for creating jobs with AWS Glue PySpark Extensions, you can also run your Spark scripts with AWS Glue Studio. In fact, we can use the exact same scripts run on Amazon EMR.
For the tests, we are using the G.2X worker type, Glue version 3.0 (Spark 3.1.1 and Python 3.7), and Python as the language choice for this comparison. We will test three worker configurations using both UI-driven job creation with AWS Glue PySpark Extensions and Apache Spark script options:
- 10 workers with a maximum of 20 DPUs
- 20 workers with a maximum of 40 DPUs
- 40 workers with a maximum of 80 DPUs



AWS Glue DataBrew
According to the documentation, “AWS Glue DataBrew is a visual data preparation tool that enables users to clean and normalize data without writing any code. Using DataBrew helps reduce the time it takes to prepare data for analytics and machine learning (ML) by up to 80 percent, compared to custom-developed data preparation. You can choose from over 250 ready-made transformations to automate data preparation tasks, such as filtering anomalies, converting data to standard formats, and correcting invalid values.”
DataBrew allows you to set the maximum number of DataBrew nodes that can be allocated when a job runs. For this comparison, we will test three different node configurations:
- 3 maximum nodes
- 10 maximum nodes
- 20 maximum nodes



Amazon Athena
According to the documentation, “Athena helps you analyze unstructured, semi-structured, and structured data stored in Amazon S3. Examples include CSV, JSON, or columnar data formats such as Apache Parquet and Apache ORC. You can use Athena to run ad-hoc queries using ANSI SQL, without the need to aggregate or load the data into Athena.”
Although Athena is classified as an ad-hoc query engine, using a CREATE TABLE AS SELECT
(CTAS) query, we can create a new table in the AWS Glue Data Catalog and write to Amazon S3 from the results of a SELECT
statement from another query. That other query statement performs a transformation on the data using SQL.
Amazon Athena is a fully managed AWS service and has no performance settings to adjust or monitor.


CTAS and Partitions
A notable limitation of Amazon Athena for the batch use case is the 100 partition limit with CTAS queries. Athena [only] supports writing to 100 unique partition and bucket combinations with CTAS. Partitioned by year
, month
, and day
, the observations test case requires 2,558 partitions, and the observations test case requires 10,433 partitions. There is a recommended workaround using an INSERT INTO
statement. However, the workaround requires additional SQL logic, computation, and most important cost. It is not practical, in my opinion, compared to other methods when a higher number of partitions are needed. To avoid the partition limit with CTAS, we will only partition by year
and bucket by month
when using Athena. Take this limitation into account when comparing the final results.
Amazon EMR with Apache Spark
According to the documentation, “Amazon EMR is a cloud big data platform for running large-scale distributed data processing jobs, interactive SQL queries, and machine learning (ML) applications using open-source analytics frameworks such as Apache Spark, Apache Hive, and Presto. You can quickly and easily create managed Spark clusters from the AWS Management Console, AWS CLI, or the Amazon EMR API.”
For this comparison, we are using two different Spark 3.1.2 EMR clusters:
- (1) r5.xlarge Master node and (2) r5.2xlarge Core nodes
- (1) r5.2xlarge Master node and (4) r5.2xlarge Core nodes
All Spark jobs are written in both Python (PySpark) and Scala. We are using the AWS Glue Data Catalog as the metastore for Spark SQL instead of Apache Hive.



Results
Data pipelines were developed and tested for each of the three test cases using the five chosen AWS ELT services and configuration variations. Each pipeline was then run 3–5 times, for a total of approximately 150 runs. The resulting AWS Glue Data Catalog table and data in Amazon S3 were deleted between each pipeline run. Each new run created a new data catalog table and wrote new results to Amazon S3. The median execution times from these tests are shown below.


Although we can make some general observations about the execution times of the chosen AWS services, the results are not meant to be a definitive guide to performance. An accurate comparison would require a deeper understanding of how each of these managed services works under the hood, in order to both optimize and balance their compute profiles correctly.
Amazon Athena
The Resultset
column contains the final number of records written to Amazon S3 by Athena. The results contain the data pipeline’s median execution time and any additional data points.

AWS Glue Studio (AWS Glue PySpark Extensions)
Tests were run with three different configurations for AWS Glue Studio using the graphical interface for creating jobs with AWS Glue PySpark Extensions. Times for each configuration were nearly identical.

AWS Glue Studio (Apache PySpark script)
As opposed to using the graphical interface for creating jobs with AWS Glue PySpark Extensions, you can also run your Apache Spark scripts with AWS Glue Studio. The tests were run with the same three configurations as above. The execution times compared to the Amazon EMR tests, below, are almost identical.

Amazon EMR with Apache Spark
Tests were run with three different configurations for Amazon EMR with Apache Spark using PySpark. The first set of results is for the 2-node EMR cluster. The second set of results is for the 4-node cluster. The third set of results is for the same 4-node cluster in which the data was not bucketed into a single file within each partition. Compare the execution times and the number of objects against the previous set of results. Too many small files can negatively impact query performance.

It is commonly stated that “Scala is almost ten times faster than Python.” However, with Amazon EMR, jobs written in Python (PySpark) and Scala had similar execution times for all three test cases.

Amazon Glue DataBrew
Tests were run with three different configurations Amazon Glue DataBrew, including 3, 10, and 20 maximum nodes. Times for each configuration were nearly identical.

Observations
- All tested AWS services can read and write to an AWS Glue Data Catalog and the underlying datastore, Amazon S3. In addition, they all work with the most common analytics data file formats.
- All tested AWS services have rich APIs providing access through the AWS CLI and SDKs, which support multiple programming languages.
- Overall, AWS Glue Studio, using the AWS Glue PySpark Extensions, appears to be the most capable ELT tool of the five services tested and with the best performance.
- Both AWS Glue DataBrew and AWS Glue Studio are no-code or low-code services, democratizing access to data for non-programmers. Conversely, Amazon Athena requires knowledge of ANSI SQL, and Amazon EMR with Apache Spark requires knowledge of Scala or Python. Be cognizant of the potential trade-offs from using no-code or low-code services on observability, configuration control, and automation.
- Both AWS Glue DataBrew and AWS Glue Studio can write a custom Parquet writer type optimized for Dynamic Frames, GlueParquet. One potential advantage, a pre-computed schema is not required before writing.
- There is a slight ‘cold-start’ with Glue Studio. Studio startup times ranged from 7 seconds to 2 minutes and 4 seconds in the tests. However, the lower execution time of AWS Glue Studio compared to Amazon EMR with Spark and AWS Glue DataBrew in the tests offsets any initial cold-start time, in my opinion.
- Changing the maximum number of units from 3 to 10 to 20 for AWS Glue DataBrew made negligible differences in job execution times. Given the nearly identical execution times, it is unclear exactly how many units are being used by the job. More importantly, how many DataBrew node hours we are being billed for. These are some of the trade-offs with a fully-managed service — visibility and fine-tuning configuration.
- Similarly, with AWS Glue Studio, using either 10 workers w/ max. 20 DPUs, 20 workers w/ max. 40 DPUs, or 40 workers w/ max. 80 DPUs resulted in nearly identical executions times.
- Amazon Athena had the fastest execution times but is limited by the 100 partition limit for large CTAS resultsets. Athena is not practical, in my opinion, compared to other ELT methods, when a higher number of partitions are needed.
- It is commonly stated that “Scala is almost ten times faster than Python.” However, with Amazon EMR, jobs written in Python (PySpark) and Scala had almost identical execution times for all three test cases.
- Using Amazon EMR with EC2 instances takes about 9 minutes to provision a new cluster for this comparison fully. Given nearly identical execution times to AWS Glue Studio with Apache Spark scripts, Glue has the clear advantage of nearly instantaneous startup times.
- AWS recently announced Amazon EMR Serverless. Although this service is still in Preview, this new version of EMR could potentially reduce or eliminate the lengthy startup time for ephemeral clusters requirements.
- Although not discussed, scheduling the data pipelines to run each night was a requirement for our use case. AWS Glue Studio jobs and AWS Glue DataBrew jobs are schedulable from those services. For Amazon EMR and Amazon Athena, we could use Amazon Managed Workflows for Apache Airflow (MWAA), AWS Data Pipeline, or AWS Step Functions combined with Amazon CloudWatch Events Rules to schedule the data pipelines.
Conclusion
Customers have many options for ELT — the cleansing, deduplication, refinement, and normalization of raw data. We examined chosen services on AWS, each capable of handling the analytics use case presented. The best choice of tools depends on your specific ELT use case and performance requirements.
This blog represents my own viewpoints and not of my employer, Amazon Web Services (AWS). All product names, logos, and brands are the property of their respective owners.
DevOps for DataOps: Building a CI/CD Pipeline for Apache Airflow DAGs
Posted by Gary A. Stafford in Analytics, AWS, Big Data, Build Automation, Cloud, Continuous Delivery, DevOps, Python, Software Development, Technology Consulting on December 14, 2021
Build an effective CI/CD pipeline to test and deploy your Apache Airflow DAGs to Amazon MWAA using GitHub Actions
Introduction
In this post, we will learn how to use GitHub Actions to build an effective CI/CD workflow for our Apache Airflow DAGs. We will use the DevOps concepts of Continuous Integration and Continuous Delivery to automate the testing and deployment of Airflow DAGs to Amazon Managed Workflows for Apache Airflow (Amazon MWAA) on AWS.
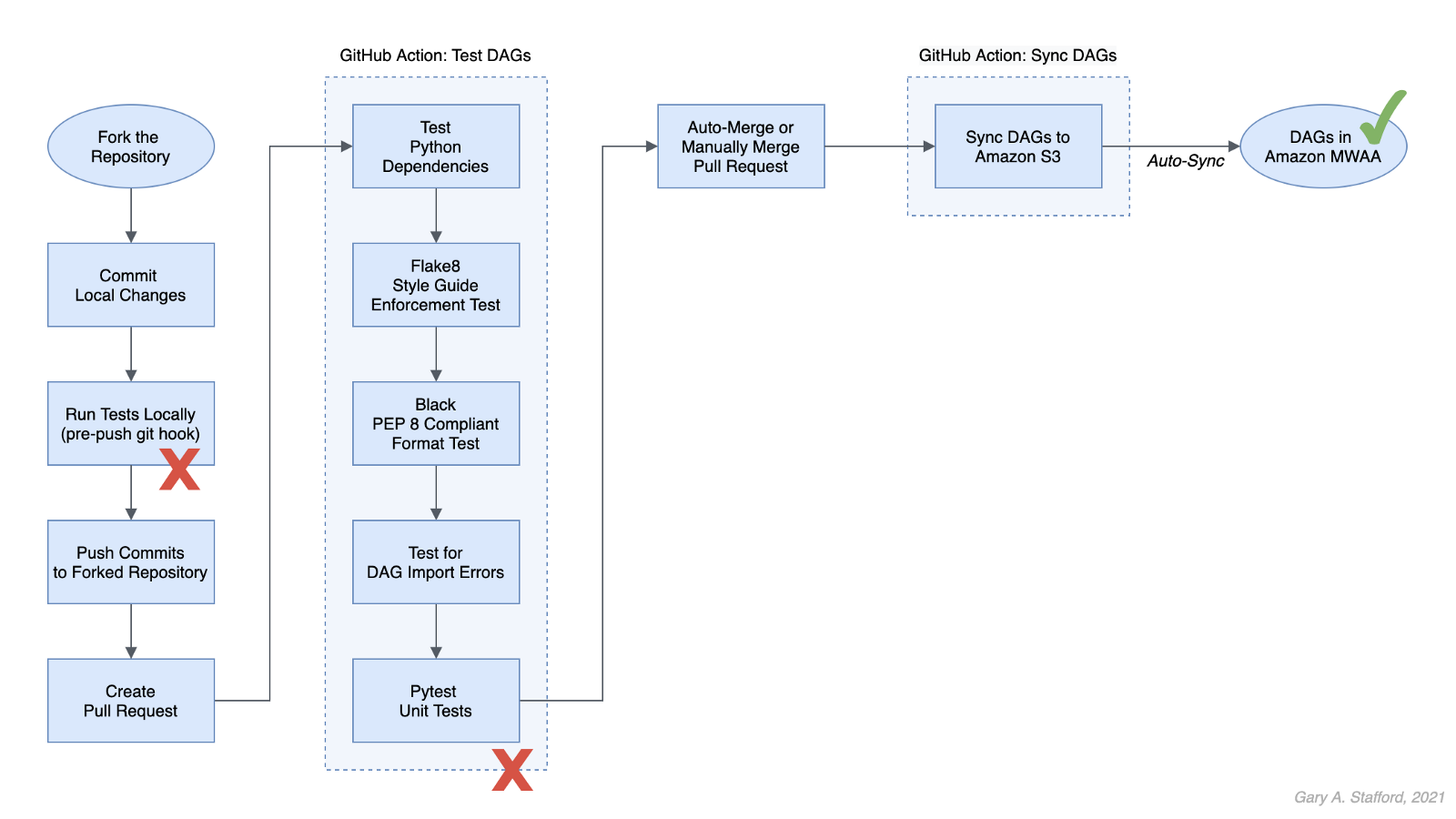
Technologies
Apache Airflow
According to the documentation, Apache Airflow is an open-source platform to author, schedule, and monitor workflows programmatically. With Airflow, you author workflows as Directed Acyclic Graphs (DAGs) of tasks written in Python.
Amazon Managed Workflows for Apache Airflow
According to AWS, Amazon Managed Workflows for Apache Airflow (Amazon MWAA) is a highly available, secure, and fully-managed workflow orchestration for Apache Airflow. MWAA automatically scales its workflow execution capacity to meet your needs and is integrated with AWS security services to help provide fast and secure access to data.
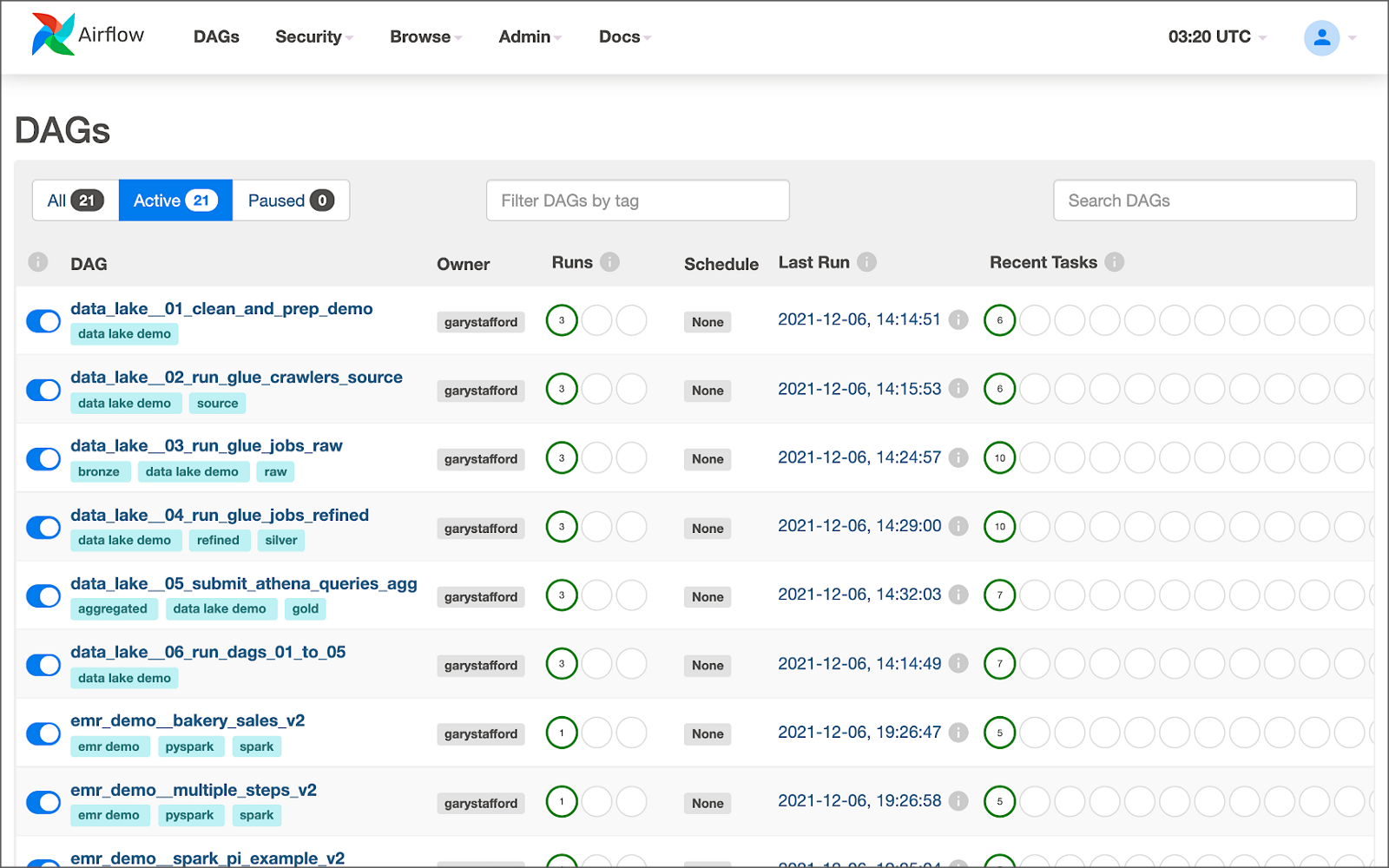
GitHub Actions
According to GitHub, GitHub Actions makes it easy to automate software workflows with CI/CD. GitHub Actions allow you to build, test, and deploy code right from GitHub. GitHub Actions are workflows triggered by GitHub events like push, issue creation, or a new release. You can leverage GitHub Actions prebuilt and maintained by the community.

If you are new to GitHub Actions, I recommend my previous post, Continuous Integration and Deployment of Docker Images using GitHub Actions.
Terminology
DataOps
According to Wikipedia, DataOps is an automated, process-oriented methodology used by analytic and data teams to improve the quality and reduce the cycle time of data analytics. While DataOps began as a set of best practices, it has now matured to become a new approach to data analytics.
DataOps applies to the entire data lifecycle from data preparation to reporting and recognizes the interconnected nature of the data analytics team and IT operations. DataOps incorporates the Agile methodology to shorten the software development life cycle (SDLC) of analytics development.
DevOps
According to Wikipedia, DevOps is a set of practices that combines software development (Dev) and IT operations (Ops). It aims to shorten the systems development life cycle and provide continuous delivery with high software quality.
DevOps is a set of practices intended to reduce the time between committing a change to a system and the change being placed into normal production, while ensuring high quality. -Wikipedia
Fail Fast
According to Wikipedia, a fail-fast system is one that immediately reports any condition that is likely to indicate a failure. Using the DevOps concept of fail fast, we build steps into our workflows to uncover errors sooner in the SDLC. We shift testing as far to the left as possible (referring to the pipeline of steps moving from left to right) and test at multiple points along the way.
Source Code
All source code for this demonstration, including the GitHub Actions, Pytest unit tests, and Git Hooks, is open-sourced and located on GitHub.
Architecture
The diagram below represents the architecture for a recent blog post and video demonstration, Lakehouse Automation on AWS with Apache Airflow. The post and video show how to programmatically load and upload data from Amazon Redshift to an Amazon S3-based data lake using Apache Airflow.
In this post, we will review how the DAGs from the previous were developed, tested, and deployed to MWAA using a variety of progressively more effective CI/CD workflows. The workflows demonstrated could also be easily applied to other Airflow resources in addition to DAGs, such as SQL scripts, configuration and data files, Python requirement files, and plugins.
Workflows
No DevOps
Below we see a minimally viable workflow for loading DAGs into Amazon MWAA, which does not use the principles of CI/CD. Changes are made in the local Airflow developer’s environment. The modified DAGs are copied directly to the Amazon S3 bucket, which are then automatically synced with Amazon MWAA, barring any errors. Those changes are also (hopefully) pushed back to the centralized version control or source code management (SCM) system, which is GitHub in this post.

There are at least two significant issues with this error-prone workflow. First, the DAGs are always out of sync between the Amazon S3 bucket and GitHub. These are two independent steps — copying or syncing the DAGs to S3 and pushing the DAGs to GitHub. A developer might continue making changes and pushing DAGs to S3 without pushing to GitHub or vice versa.
Secondly, the DevOps concept of fail-fast is missing. The first time you know your DAG contains errors is likely when it is synced to MWAA and throws an Import Error. By then, the DAG has already been copied to S3, synced to MWAA, and possibly pushed to GitHub, which other developers could then pull.

GitHub Actions
A significant step up from the previous workflow is using GitHub Actions to test and deploy your code after pushing it to GitHub. Although in this workflow, code is still ‘pushed straight to Trunk’ (the main branch in GitHub) and risks other developers in a collaborative environment pulling potentially erroneous code, you have far less chance of DAG errors making it to MWAA.

Using GitHub Actions, you also eliminate human error that could result in the changes to DAGs not being synced to Amazon S3. Lastly, using this workflow improves security by eliminating the need to provide direct access to the Airflow Amazon S3 bucket to Airflow Developers.
Types of Tests
The first GitHub Action, test_dags.yml
, is triggered on a push to the dags
directory in the main
branch of the repository. It is also triggered whenever a pull request is made for the main
branch. The first GitHub Action runs a battery of tests, including checking Python dependencies, code style, code quality, DAG import errors, and unit tests. The tests catch issues with DAGs before being synced to S3 by a second GitHub Action.
name: Test DAGs on: push: paths: - 'dags/**' pull_request: branches: - main jobs: test: runs-on: ubuntu-latest steps: - uses: actions/checkout@v2 - name: Set up Python uses: actions/setup-python@v2 with: python-version: '3.7' - name: Install dependencies run: | python -m pip install --upgrade pip pip install -r requirements/requirements.txt pip check - name: Lint with Flake8 run: | pip install flake8 flake8 --ignore E501 dags --benchmark -v - name: Confirm Black code compliance (psf/black) run: | pip install pytest-black pytest dags --black -v - name: Test with Pytest run: | pip install pytest cd tests || exit pytest tests.py -v

Python Dependencies
The first test installs the modules listed in the requirements.txt
file used locally to develop the application. This test is designed to uncover any missing or conflicting modules.
- name: Install dependencies
run: |
python -m pip install --upgrade pip
pip install -r requirements/requirements.txt
pip check
It is essential to develop your DAGs against the same version of Python and with the same version of the Python modules used in your Airflow environment. You can use the BashOperator to run shell commands to obtain the versions of Python and module installed in your Airflow environment:
python3 --version; python3 -m pip list
A snippet of log output from DAG showing Python version and Python modules available in MWAA 2.0.2:

The latest stable release of Airflow is currently version 2.2.2, released 2021-11-15. However, as of December 2021, Amazon’s latest version of MWAA 2.x is version 2.0.2, released 2021-04-19. MWAA 2.0.2 currently runs Python3 version 3.7.10.

Flake8
Known as ‘your tool for style guide enforcement,’ Flake8 is described as the modular source code checker. It is a command-line utility for enforcing style consistency across Python projects. Flake8 is a wrapper around PyFlakes, pycodestyle, and Ned Batchelder’s McCabe script. The module, pycodestyle
, is a tool to check your Python code against some of the style conventions in PEP 8.
Flake8 is highly configurable, with options to ignore specific rules if not required by your development team. For example, in this demonstration, I intentionally ignored rule E501, which states that ‘line length should be limited to 72 characters.’
- name: Lint with Flake8
run: |
pip install flake8
flake8 --ignore E501 dags --benchmark -v
Black
Known as ‘the uncompromising code formatter,’ Python code formatted using Black (referred to as Blackened code) looks the same regardless of the project you’re reading. Formatting becomes transparent, allowing teams to focus on the content instead. Black makes code review faster by producing the smallest diffs possible, assuming all developers are using black
to format their code.
The Airflow DAGs in this GitHub repository are automatically formatted with black
using a pre-commit
Git Hooks before being committed and pushed to GitHub. The test confirms black
code compliance.
- name: Confirm Black code compliance (psf/black)
run: |
pip install pytest-black
pytest dags --black -v
Pytest
The pytest framework describes itself as a mature, fully-featured Python testing tool that helps you write better programs. The Pytest framework makes it easy to write small tests yet scales to support complex functional testing for applications and libraries.
The GitHub Action in the GitHub project, test_dags.yml
, calls the tests.py
file, also contained in the project.
- name: Test with Pytest
run: |
pip install pytest
cd tests || exit
pytest tests.py -v
The tests.py
file contains several pytest
unit tests. The tests are based on my project requirements; your tests will vary. These tests confirm that all DAGs:
- Do not contain DAG Import Errors (test catches 75% of my errors);
- Follow specific file naming conventions;
- Include a description and an owner other than ‘airflow’;
- Contain required project tags;
- Do not send emails (my projects use SNS or Slack for notifications);
- Do not retry more than three times;
import os
import sys
import pytest
from airflow.models import DagBag
sys.path.append(os.path.join(os.path.dirname(__file__), "../dags"))
sys.path.append(os.path.join(os.path.dirname(__file__), "../dags/utilities"))
# Airflow variables called from DAGs under test are stubbed out
os.environ["AIRFLOW_VAR_DATA_LAKE_BUCKET"] = "test_bucket"
os.environ["AIRFLOW_VAR_ATHENA_QUERY_RESULTS"] = "SELECT 1;"
os.environ["AIRFLOW_VAR_SNS_TOPIC"] = "test_topic"
os.environ["AIRFLOW_VAR_REDSHIFT_UNLOAD_IAM_ROLE"] = "test_role_1"
os.environ["AIRFLOW_VAR_GLUE_CRAWLER_IAM_ROLE"] = "test_role_2"
@pytest.fixture(params=["../dags/"])
def dag_bag(request):
return DagBag(dag_folder=request.param, include_examples=False)
def test_no_import_errors(dag_bag):
assert not dag_bag.import_errors
def test_requires_tags(dag_bag):
for dag_id, dag in dag_bag.dags.items():
assert dag.tags
def test_requires_specific_tag(dag_bag):
for dag_id, dag in dag_bag.dags.items():
try:
assert dag.tags.index("data lake demo") >= 0
except ValueError:
assert dag.tags.index("redshift demo") >= 0
def test_desc_len_greater_than_fifteen(dag_bag):
for dag_id, dag in dag_bag.dags.items():
assert len(dag.description) > 15
def test_owner_len_greater_than_five(dag_bag):
for dag_id, dag in dag_bag.dags.items():
assert len(dag.owner) > 5
def test_owner_not_airflow(dag_bag):
for dag_id, dag in dag_bag.dags.items():
assert str.lower(dag.owner) != "airflow"
def test_no_emails_on_retry(dag_bag):
for dag_id, dag in dag_bag.dags.items():
assert not dag.default_args["email_on_retry"]
def test_no_emails_on_failure(dag_bag):
for dag_id, dag in dag_bag.dags.items():
assert not dag.default_args["email_on_failure"]
def test_three_or_less_retries(dag_bag):
for dag_id, dag in dag_bag.dags.items():
assert dag.default_args["retries"] <= 3
def test_dag_id_contains_prefix(dag_bag):
for dag_id, dag in dag_bag.dags.items():
assert str.lower(dag_id).find("__") != -1
def test_dag_id_requires_specific_prefix(dag_bag):
for dag_id, dag in dag_bag.dags.items():
assert str.lower(dag_id).startswith("data_lake__") \
or str.lower(dag_id).startswith("redshift_demo__")
If you are building custom Airflow Operators, additional unit, functional, and integration tests are recommended.
Fork and Pull
We can improve on the practice of pushing directly to Trunk by implementing one of two collaborative development models, recommended by GitHub:
- The Shared repository model: uses ‘topic’ branches, which are reviewed, approved, and merged into the main branch.
- Fork and pull model: a repo is forked, changes are made, a pull request is created, the request is reviewed, and if approved, merged into the main branch.
In the fork and pull model, we create a fork of the DAG repository where we make our changes. We then commit and push those changes back to the forked repository. When ready, we create a pull request. If the pull request is approved and passes all the tests, it is manually or automatically merged into the main branch. DAGs are then synced to S3 and, eventually, to MWAA. I usually prefer to trigger merges manually once all tests have passed.
The fork and pull model greatly reduces the chance that bad code is merged to the main branch before passing all tests.

Syncing DAGs to S3
The second GitHub Action in the GitHub project, sync_dags.yml
, is triggered when the previous Action, test_dags.yml
, completes successfully, or in the case of the folk and pull method, the merge to the main
branch is successful.
name: Sync DAGs
on:
workflow_run:
workflows:
- 'Test DAGs'
types:
- completed
pull_request:
types:
- closed
jobs:
deploy:
runs-on: ubuntu-latest
if: ${{ github.event.workflow_run.conclusion == 'success' }}
steps:
- uses: actions/checkout@master
- uses: jakejarvis/s3-sync-action@master
env:
AWS_S3_BUCKET: ${{ secrets.AWS_S3_BUCKET }}
AWS_ACCESS_KEY_ID: ${{ secrets.AWS_ACCESS_KEY_ID }}
AWS_SECRET_ACCESS_KEY: ${{ secrets.AWS_SECRET_ACCESS_KEY }}
AWS_REGION: 'us-east-1'
SOURCE_DIR: 'dags'
DEST_DIR: 'dags'
The GitHub Action, sync_dags.yml
, requires three GitHub encrypted secrets, created in advance and associated with the GitHub repository. According to GitHub, secrets are encrypted environment variables you create in an organization, repository, or repository environment. Encrypted secrets allow you to store sensitive information, such as access tokens, in your repository. The secrets that you create are available to use in GitHub Actions workflows.

The DAGs are synced to Amazon S3 and, eventually, automatically synced to MWAA.

Local Testing and Git Hooks
To further improve your CI/CD workflows, you should consider using Git Hooks. Using Git Hooks, we can ensure code is tested locally before committing and pushing changes to GitHub. Testing locally allows us to fail-faster, catching errors during development instead of once code is pushed to GitHub.

According to the documentation, Git has a way to fire off custom scripts when certain important actions occur. There are two types of hooks: client-side and server-side. Client-side hooks are triggered by operations such as committing and merging, while server-side hooks run on network operations such as receiving pushed commits.
You can use these hooks for all sorts of reasons. I often use a client-side pre-commit
hook to format DAGs using black
. Using a client-side pre-push
Git Hook, we will ensure that tests are run before pushing the DAGs to GitHub. According to Git, The pre-push
hook runs when the git push
command is executed after the remote refs have been updated but before any objects have been transferred. You can use it to validate a set of ref updates before a push occurs. A non-zero exit code will abort the push. The test could instead be run as part of the pre-commit
hook if they are not too time-consuming.
To use the pre-push
hook, create the following file within the local repository, .git/hooks/pre-push
:
#!/bin/sh
# do nothing if there are no commits to push
if [ -z "$(git log @{u}..)" ]; then
exit 0
fi
sh ./run_tests_locally.sh
Then, run the following chmod
command to make the hook executable:
chmod 755 .git/hooks/pre-push
The the pre-push
hook runs the shell script, run_tests_locally.sh
. The script executes nearly identical tests, locally, as the GitHub Action, test_dags.yml
, does remotely on GitHub:
#!/bin/sh
echo "Starting Flake8 test..."
flake8 --ignore E501 dags --benchmark || exit 1
echo "Starting Black test..."
python3 -m pytest --cache-clear
python3 -m pytest dags/ --black -v || exit 1
echo "Starting Pytest tests..."
cd tests || exit
python3 -m pytest tests.py -v || exit 1
echo "All tests completed successfully! 🥳"
References
Here are some additional references for testing and deploying Airflow DAGs and the use of GitHub Actions:
- Astronomer: Testing Airflow DAGs (documentation)
- Astronomer: Testing Airflow to Bullet Proof Your Code (YouTube video)
- GitHub: Building and testing Python (documentation)
- Manning: Chapter 9 of Data Pipelines with Apache Airflow
This blog represents my own viewpoints and not of my employer, Amazon Web Services (AWS). All product names, logos, and brands are the property of their respective owners.
Video Demonstration: Lakehouse Automation on AWS with Apache Airflow
Posted by Gary A. Stafford in Analytics, AWS, Build Automation, Cloud, DevOps, Python, SQL, Technology Consulting on December 2, 2021
Programmatically load and upload data from Amazon Redshift to an Amazon S3-based Data Lake using Apache Airflow
Introduction
In the following video demonstration, we will learn how to programmatically load and upload data from Amazon Redshift to an Amazon S3-based Data Lake using Apache Airflow. Since we are on AWS, we will be using the fully-managed Amazon Managed Workflows for Apache Airflow (Amazon MWAA). Using Airflow, we will COPY
raw data into staging tables, then merge that staging data into a series of tables. We will then load incremental data into Redshift on a regular schedule. Next, we will join and aggregate data from several tables and UNLOAD
the resulting dataset to an Amazon S3-based data lake. Lastly, we will catalog the data in S3 using AWS Glue and query with Amazon Athena.

Demonstration
Source Code
The source code for this demonstration, including the Airflow DAGs, SQL statements, and data files, is open-sourced and located on GitHub.
DAGs
The DAGs included in the GitHub project are:
- redshift_demo__01_create_tables.py
- redshift_demo__02_initial_load.py
- redshift_demo__03_incremental_load.py
- redshift_demo__04_unload_data.py
- redshift_demo__05_catalog_and_query.py
- redshift_demo__06_run_dags_01_to_05.py
- redshift_demo__06B_run_dags_01_to_05.py (alt. ver. w/external notifications module)

This blog represents my own viewpoints and not of my employer, Amazon Web Services (AWS). All product names, logos, and brands are the property of their respective owners.
Video Demonstration: Building a Data Lake with Apache Airflow
Posted by Gary A. Stafford in Analytics, AWS, Big Data, Build Automation, Cloud, Python on November 12, 2021
Build a simple Data Lake on AWS using a combination of services, including Amazon Managed Workflows for Apache Airflow (Amazon MWAA), AWS Glue, AWS Glue Studio, Amazon Athena, and Amazon S3
Introduction
In the following video demonstration, we will build a simple data lake on AWS using a combination of services, including Amazon Managed Workflows for Apache Airflow (Amazon MWAA), AWS Glue Data Catalog, AWS Glue Crawlers, AWS Glue Jobs, AWS Glue Studio, Amazon Athena, Amazon Relational Database Service (Amazon RDS), and Amazon S3.
Using a series of Airflow DAGs (Directed Acyclic Graphs), we will catalog and move data from three separate data sources into our Amazon S3-based data lake. Once in the data lake, we will perform ETL (or more accurately ELT) on the raw data — cleansing, augmenting, and preparing it for data analytics. Finally, we will perform aggregations on the refined data and write those final datasets back to our data lake. The data lake will be organized around the data lake pattern of bronze (aka raw), silver (aka refined), and gold (aka aggregated) data, popularized by Databricks.

Demonstration
Source Code
The source code for this demonstration, including the Airflow DAGs, SQL files, and data files, is open-sourced and located on GitHub.
DAGs
The DAGs shown in the video demonstration have been renamed for easier project management within the Airflow UI. The DAGs included in the GitHub project are as follows:
- data_lake__01_clean_and_prep_demo.py
- data_lake__02_run_glue_crawlers_source.py
- data_lake__03_run_glue_jobs_raw.py
- data_lake__04_run_glue_jobs_refined.py
- data_lake__05_submit_athena_queries_agg.py
- data_lake__06_run_dags_01_to_05.py
This blog represents my own viewpoints and not of my employer, Amazon Web Services (AWS). All product names, logos, and brands are the property of their respective owners.
Getting Started with Spark Structured Streaming and Kafka on AWS using Amazon MSK and Amazon EMR
Posted by Gary A. Stafford in Analytics, AWS, Big Data, Build Automation, Cloud, Software Development on September 9, 2021
Exploring Apache Spark with Apache Kafka using both batch queries and Spark Structured Streaming
Introduction
Structured Streaming is a scalable and fault-tolerant stream processing engine built on the Spark SQL engine. Using Structured Streaming, you can express your streaming computation the same way you would express a batch computation on static data. In this post, we will learn how to use Apache Spark and Spark Structured Streaming with Apache Kafka. Specifically, we will utilize Structured Streaming on Amazon EMR (fka Amazon Elastic MapReduce) with Amazon Managed Streaming for Apache Kafka (Amazon MSK). We will consume from and publish to Kafka using both batch and streaming queries. Spark jobs will be written in Python with PySpark for this post.

Apache Spark
According to the documentation, Apache Spark is a unified analytics engine for large-scale data processing. It provides high-level APIs in Java, Scala, Python (PySpark), and R, and an optimized engine that supports general execution graphs. In addition, Spark supports a rich set of higher-level tools, including Spark SQL for SQL and structured data processing, MLlib for machine learning, GraphX for graph processing, and Structured Streaming for incremental computation and stream processing.

Spark Structured Streaming
According to the documentation, Spark Structured Streaming is a scalable and fault-tolerant stream processing engine built on the Spark SQL engine. You can express your streaming computation the same way you would express a batch computation on static data. The Spark SQL engine will run it incrementally and continuously and update the final result as streaming data continues to arrive. In short, Structured Streaming provides fast, scalable, fault-tolerant, end-to-end, exactly-once stream processing without the user having to reason about streaming.
Amazon EMR
According to the documentation, Amazon EMR (fka Amazon Elastic MapReduce) is a cloud-based big data platform for processing vast amounts of data using open source tools such as Apache Spark, Hadoop, Hive, HBase, Flink, and Hudi, and Presto. Amazon EMR is a fully managed AWS service that makes it easy to set up, operate, and scale your big data environments by automating time-consuming tasks like provisioning capacity and tuning clusters.
A deployment option for Amazon EMR since December 2020, Amazon EMR on EKS, allows you to run Amazon EMR on Amazon Elastic Kubernetes Service (Amazon EKS). With the EKS deployment option, you can focus on running analytics workloads while Amazon EMR on EKS builds, configures, and manages containers for open-source applications.
If you are new to Amazon EMR for Spark, specifically PySpark, I recommend an earlier two-part series of posts, Running PySpark Applications on Amazon EMR: Methods for Interacting with PySpark on Amazon Elastic MapReduce.
Apache Kafka
According to the documentation, Apache Kafka is an open-source distributed event streaming platform used by thousands of companies for high-performance data pipelines, streaming analytics, data integration, and mission-critical applications.
Amazon MSK
Apache Kafka clusters are challenging to set up, scale, and manage in production. According to the documentation, Amazon MSK is a fully managed AWS service that makes it easy for you to build and run applications that use Apache Kafka to process streaming data. With Amazon MSK, you can use native Apache Kafka APIs to populate data lakes, stream changes to and from databases, and power machine learning and analytics applications.
Prerequisites
This post will focus primarily on configuring and running Apache Spark jobs on Amazon EMR. To follow along, you will need the following resources deployed and configured on AWS:
- Amazon S3 bucket (holds Spark resources and output);
- Amazon MSK cluster (using IAM Access Control);
- Amazon EKS container or an EC2 instance with the Kafka APIs installed and capable of connecting to Amazon MSK;
- Connectivity between the Amazon EKS cluster or EC2 and Amazon MSK cluster;
- Ensure the Amazon MSK Configuration has
auto.create.topics.enable=true
; this setting isfalse
by default;
As shown in the architectural diagram above, the demonstration uses three separate VPCs within the same AWS account and AWS Region, us-east-1
, for Amazon EMR, Amazon MSK, and Amazon EKS. The three VPCs are connected using VPC Peering. Ensure you expose the correct ingress ports and the corresponding CIDR ranges within your Amazon EMR, Amazon MSK, and Amazon EKS Security Groups. For additional security and cost savings, use a VPC endpoint for private communications between Amazon EMR and Amazon S3.
Source Code
All source code for this post and the two previous posts in the Amazon MSK series, including the Python/PySpark scripts demonstrated here, are open-sourced and located on GitHub.
PySpark Scripts
According to the Apache Spark documentation, PySpark is an interface for Apache Spark in Python. It allows you to write Spark applications using Python API. PySpark supports most of Spark’s features such as Spark SQL, DataFrame, Streaming, MLlib (Machine Learning), and Spark Core.
There are nine Python/PySpark scripts covered in this post:
- Initial sales data published to Kafka
01_seed_sales_kafka.py - Batch query of Kafka
02_batch_read_kafka.py - Streaming query of Kafka using grouped aggregation
03_streaming_read_kafka_console.py - Streaming query using sliding event-time window
04_streaming_read_kafka_console_window.py - Incremental sales data published to Kafka
05_incremental_sales_kafka.py - Streaming query from/to Kafka using grouped aggregation
06_streaming_read_kafka_kafka.py - Batch query of streaming query results in Kafka
07_batch_read_kafka.py - Streaming query using static join and sliding window
08_streaming_read_kafka_join_window.py - Streaming query using static join and grouped aggregation
09_streaming_read_kafka_join.py
Amazon MSK Authentication and Authorization
Amazon MSK provides multiple authentication and authorization methods to interact with the Apache Kafka APIs. For this post, the PySpark scripts use Kafka connection properties specific to IAM Access Control. You can use IAM to authenticate clients and to allow or deny Apache Kafka actions. Alternatively, you can use TLS or SASL/SCRAM to authenticate clients and Apache Kafka ACLs to allow or deny actions. In a recent post, I demonstrated the use of SASL/SCRAM and Kafka ACLs with Amazon MSK:Securely Decoupling Applications on Amazon EKS using Kafka with SASL/SCRAM.
Language Choice
According to the latest Spark 3.1.2 documentation, Spark runs on Java 8/11, Scala 2.12, Python 3.6+, and R 3.5+. The Spark documentation contains code examples written in all four languages and provides sample code on GitHub for Scala, Java, Python, and R. Spark is written in Scala.

There are countless posts and industry opinions on choosing the best language for Spark. Taking no sides, I have selected the language I use most frequently for data analytics, Python using PySpark. Compared to Scala, these two languages exhibit some of the significant differences: compiled versus interpreted, statically-typed versus dynamically-typed, JVM- versus non-JVM-based, Scala’s support for concurrency and true multi-threading, and Scala’s 10x raw performance versus the perceived ease-of-use, larger community, and relative maturity of Python.
Preparation
Amazon S3
We will start by gathering and copying the necessary files to your Amazon S3 bucket. The bucket will serve as the location for the Amazon EMR bootstrap script, additional JAR files required by Spark, PySpark scripts, CSV-format data files, and eventual output from the Spark jobs.
There are a small set of additional JAR files required by the Spark jobs we will be running. Download the JARs from Maven Central and GitHub, and place them in the emr_jars
project directory. The JARs will include AWS MSK IAM Auth, AWS SDK, Kafka Client, Spark SQL for Kafka, Spark Streaming, and other dependencies.
cd ./pyspark/emr_jars/
wget https://github.com/aws/aws-msk-iam-auth/releases/download/1.1.0/aws-msk-iam-auth-1.1.0-all.jar
wget https://repo1.maven.org/maven2/software/amazon/awssdk/bundle/2.17.28/bundle-2.17.28.jar
wget https://repo1.maven.org/maven2/org/apache/commons/commons-pool2/2.11.0/commons-pool2-2.11.0.jar
wget https://repo1.maven.org/maven2/org/apache/kafka/kafka-clients/2.8.0/kafka-clients-2.8.0.jar
wget https://repo1.maven.org/maven2/org/apache/spark/spark-sql-kafka-0-10_2.12/3.1.2/spark-sql-kafka-0-10_2.12-3.1.2.jar
wget https://repo1.maven.org/maven2/org/apache/spark/spark-streaming_2.12/3.1.2/spark-streaming_2.12-3.1.2.jar
wget https://repo1.maven.org/maven2/org/apache/spark/spark-tags_2.12/3.1.2/spark-tags_2.12-3.1.2.jar
wget https://repo1.maven.org/maven2/org/apache/spark/spark-token-provider-kafka-0-10_2.12/3.1.2/spark-token-provider-kafka-0-10_2.12-3.1.2.jar
Next, update the SPARK_BUCKET
environment variable, then upload the JARs and all necessary project files from your copy of the GitHub project repository to your Amazon S3 bucket using the AWS s3
API.
cd ./pyspark/
export SPARK_BUCKET="<your-bucket-111222333444-us-east-1>"
aws s3 cp emr_jars/ \
"s3://${SPARK_BUCKET}/jars/" --recursive
aws s3 cp pyspark_scripts/ \
"s3://${SPARK_BUCKET}/spark/" --recursive
aws s3 cp emr_bootstrap/ \
"s3://${SPARK_BUCKET}/spark/" --recursive
aws s3 cp data/ \
"s3://${SPARK_BUCKET}/spark/" --recursive
Amazon EMR
The GitHub project repository includes a sample AWS CloudFormation template and an associated JSON-format CloudFormation parameters file. The template, stack.yml
, accepts several parameters. To match your environment, you will need to update the parameter values such as SSK key, Subnet, and S3 bucket. The template will build a minimally-sized Amazon EMR cluster with one master and two core nodes in an existing VPC. The template can be easily modified to meet your requirements and budget.
aws cloudformation deploy \
--stack-name spark-kafka-demo-dev \
--template-file ./cloudformation/stack.yml \
--parameter-overrides file://cloudformation/dev.json \
--capabilities CAPABILITY_NAMED_IAM
Whether you decide to use the CloudFormation template, two essential Spark configuration items in the EMR template are the list of applications to install and the bootstrap script deployment.
Below, we see the EMR bootstrap shell script, bootstrap_actions.sh, deployed and executed on the cluster’s nodes.
The script performed several tasks, including deploying the additional JAR files we copied to Amazon S3 earlier.

AWS Systems Manager Parameter Store
The PySpark scripts in this demonstration will obtain two parameters from the AWS Systems Manager (AWS SSM) Parameter Store. They include the Amazon MSK bootstrap brokers and the Amazon S3 bucket that contains the Spark assets. Using the Parameter Store ensures that no sensitive or environment-specific configuration is hard-coded into the PySpark scripts. Modify and execute the ssm_params.sh
script to create two AWS SSM Parameter Store parameters.
aws ssm put-parameter \
--name /kafka_spark_demo/kafka_servers \
--type String \
--value "<b-1.your-brokers.kafka.us-east-1.amazonaws.com:9098,b-2.your-brokers.kafka.us-east-1.amazonaws.com:9098>" \
--description "Amazon MSK Kafka broker list" \
--overwrite
aws ssm put-parameter \
--name /kafka_spark_demo/kafka_demo_bucket \
--type String \
--value "<your-bucket-111222333444-us-east-1>" \
--description "Amazon S3 bucket" \
--overwrite
Spark Submit Options with Amazon EMR
Amazon EMR provides multiple options to run Spark jobs. The recommended method for PySpark scripts is to use Amazon EMR Steps from the EMR console or AWS CLI to submit work to Spark installed on an EMR cluster. In the console and CLI, you do this using a Spark application step, which runs the spark-submit
script as a step on your behalf. With the API, you use a Step to invoke spark-submit
using command-runner.jar
. Alternately, you can SSH into the EMR cluster’s master node and run spark-submit
. We will employ both techniques to run the PySpark jobs.
Securely Accessing Amazon MSK from Spark
Each of the PySpark scripts demonstrated in this post uses a common pattern for accessing Amazon MSK from Amazon EMR using IAM Authentication. Whether producing or consuming messages from Kafka, the same security-related options are used to configure Spark (starting at line 10, below). The details behind each option are outlined in the Security section of the Spark Structured Streaming + Kafka Integration Guide and the Configure clients for IAM access control section of the Amazon MSK IAM access control documentation.
Data Source and Analysis Objective
For this post, we will continue to use data from PostgreSQL’s sample Pagila database. The database contains simulated movie rental data. The dataset is fairly small, making it less than ideal for ‘big data’ use cases but small enough to quickly install and minimize data storage and analytical query costs.
According to mastersindatascience.org, data analytics is “…the process of analyzing raw data to find trends and answer questions…” Using Spark, we can analyze the movie rental sales data as a batch or in near-real-time using Structured Streaming to answer different questions. For example, using batch computations on static data, we could answer the question, how do the current total all-time sales for France compare to the rest of Europe? Or, what were the total sales for India during August? Using streaming computations, we can answer questions like, what are the sales volumes for the United States during this current four-hour marketing promotional period? Or, are sales to North America beginning to slow as the Olympics are aired during prime time?
Data analytics — the process of analyzing raw data to find trends and answer questions. (mastersindatascience.org)
Batch Queries
Before exploring the more advanced topic of streaming computations with Spark Structured Streaming, let’s first use a simple batch query and a batch computation to consume messages from the Kafka topic, perform a basic aggregation, and write the output to both the console and Amazon S3.
PySpark Job 1: Initial Sales Data
Kafka supports Protocol Buffers, JSON Schema, and Avro. However, to keep things simple in this first post, we will use JSON. We will seed a new Kafka topic with an initial batch of 250 JSON-format messages. This first batch of messages represents previous online movie rental sale transaction records. We will use these sales transactions for both batch and streaming queries.
The PySpark script, 01_seed_sales_kafka.py
, and the seed data file, sales_seed.csv
, are both read from Amazon S3 by Spark, running on Amazon EMR. The location of the Amazon S3 bucket name and the Amazon MSK’s broker list values are pulled from AWS SSM Parameter Store using the parameters created earlier. The Kafka topic that stores the sales data, pagila.sales.spark.streaming
, is created automatically by the script the first time it runs.
Update the two environment variables, then submit your first Spark job as an Amazon EMR Step using the AWS CLI and the emr
API:

From the Amazon EMR console, we should observe the Spark job has been completed successfully in about 30–90 seconds.

The Kafka Consumer API allows applications to read streams of data from topics in the Kafka cluster. Using the Kafka Consumer API, from within a Kubernetes container running on Amazon EKS or an EC2 instance, we can observe that the new Kafka topic has been successfully created and that messages (initial sales data) have been published to the new Kafka topic.
export BBROKERS="b-1.your-cluster.kafka.us-east-1.amazonaws.com:9098,b-2.your-cluster.kafka.us-east-1.amazonaws.com:9098, ..."
bin/kafka-console-consumer.sh \
--topic pagila.sales.spark.streaming \
--from-beginning \
--property print.key=true \
--property print.value=true \
--property print.offset=true \
--property print.partition=true \
--property print.headers=true \
--property print.timestamp=true \
--bootstrap-server $BBROKERS \
--consumer.config config/client-iam.properties

PySpark Job 2: Batch Query of Amazon MSK Topic
The PySpark script, 02_batch_read_kafka.py
, performs a batch query of the initial 250 messages in the Kafka topic. When run, the PySpark script parses the JSON-format messages, then aggregates the data by both total sales and order count, by country, and finally, sorts by total sales.
window = Window.partitionBy("country").orderBy("amount")
window_agg = Window.partitionBy("country")
.withColumn("row", F.row_number().over(window)) \
.withColumn("orders", F.count(F.col("amount")).over(window_agg)) \
.withColumn("sales", F.sum(F.col("amount")).over(window_agg)) \
.where(F.col("row") == 1).drop("row") \
The results are written to both the console as stdout
and to Amazon S3 in CSV format.
Again, submit this job as an Amazon EMR Step using the AWS CLI and the emr
API:
To view the console output, click on ‘View logs’ in the Amazon EMR console, then click on the stdout
logfile, as shown below.

The stdout
logfile should contain the top 25 total sales and order counts, by country, based on the initial 250 sales records.
+------------------+------+------+
|country |sales |orders|
+------------------+------+------+
|India |138.80|20 |
|China |133.80|20 |
|Mexico |106.86|14 |
|Japan |100.86|14 |
|Brazil |96.87 |13 |
|Russian Federation|94.87 |13 |
|United States |92.86 |14 |
|Nigeria |58.93 |7 |
|Philippines |58.92 |8 |
|South Africa |46.94 |6 |
|Argentina |42.93 |7 |
|Germany |39.96 |4 |
|Indonesia |38.95 |5 |
|Italy |35.95 |5 |
|Iran |33.95 |5 |
|South Korea |33.94 |6 |
|Poland |30.97 |3 |
|Pakistan |25.97 |3 |
|Taiwan |25.96 |4 |
|Mozambique |23.97 |3 |
|Ukraine |23.96 |4 |
|Vietnam |23.96 |4 |
|Venezuela |22.97 |3 |
|France |20.98 |2 |
|Peru |19.98 |2 |
+------------------+------+------+
only showing top 25 rows
The PySpark script also wrote the same results to Amazon S3 in CSV format.

The total sales and order count for 69 countries were computed, sorted, and coalesced into a single CSV file.
Streaming Queries
To demonstrate streaming queries with Spark Structured Streaming, we will use a combination of two PySpark scripts. The first script, 03_streaming_read_kafka_console.py
, will perform a streaming query and computation of messages in the Kafka topic, aggregating the total sales and number of orders. Concurrently, the second PySpark script, 04_incremental_sales_kafka.py
, will read additional Pagila sales data from a CSV file located on Amazon S3 and write messages to the Kafka topic at a rate of two messages per second. The first script, 03_streaming_read_kafka_console.py
, will stream aggregations in micro-batches of one-minute increments to the console. Spark Structured Streaming queries are processed using a micro-batch processing engine, which processes data streams as a series of small, batch jobs.
Note that this first script performs grouped aggregations as opposed to aggregations over a sliding event-time window. The aggregated results represent the total, all-time sales at a point in time, based on all the messages currently in the topic when the micro-batch was computed.
To follow along with this part of the demonstration, you can run the two Spark jobs as concurrent steps on the existing Amazon EMR cluster, or create a second EMR cluster, identically configured to the existing cluster, to run the second PySpark script, 04_incremental_sales_kafka.py
. Using a second cluster, you can use a minimally-sized single master node cluster with no core nodes to save cost.
PySpark Job 3: Streaming Query to Console
The first PySpark scripts, 03_streaming_read_kafka_console.py
, performs a streaming query of messages in the Kafka topic. The script then aggregates the data by both total sales and order count, by country, and finally, sorts by total sales.
.groupBy("country") \
.agg(F.count("amount"), F.sum("amount")) \
.orderBy(F.col("sum(amount)").desc()) \
.select("country",
(F.format_number(F.col("sum(amount)"), 2)).alias("sales"),
(F.col("count(amount)")).alias("orders")) \
The results are streamed to the console using the processingTime
trigger parameter. A trigger defines how often a streaming query should be executed and emit new data. The processingTime
parameter sets a trigger that runs a micro-batch query periodically based on the processing time (e.g. ‘5 minutes’ or ‘1 hour’). The trigger is currently set to a minimal processing time of one minute for ease of demonstration.
.trigger(processingTime="1 minute") \
.outputMode("complete") \
.format("console") \
.option("numRows", 25) \
For demonstration purposes, we will run the Spark job directly from the master node of the EMR Cluster. This method will allow us to easily view the micro-batches and associated logs events as they are output to the console. The console is normally used for testing purposes. Submitting the PySpark script from the cluster’s master node is an alternative to submitting an Amazon EMR Step. Connect to the master node of the Amazon EMR cluster using SSH, as the hadoop
user:
export EMR_MASTER=<your-emr-master-dns.compute-1.amazonaws.com>
export EMR_KEY_PATH=path/to/key/<your-ssk-key.pem>
ssh -i ${EMR_KEY_PATH} hadoop@${EMR_MASTER}
Submit the PySpark script, 03_streaming_read_kafka_console.py
, to Spark:
export SPARK_BUCKET="<your-bucket-111222333444-us-east-1>"
spark-submit s3a://${SPARK_BUCKET}/spark/03_streaming_read_kafka_console
.py
Before running the second PySpark script, 04_incremental_sales_kafka.py
, let the first script run long enough to pick up the existing sales data in the Kafka topic. Within about two minutes, you should see the first micro-batch of aggregated sales results, labeled ‘Batch: 0’ output to the console. This initial micro-batch should contain the aggregated results of the existing 250 messages from Kafka. The streaming query’s first micro-batch results should be identical to the previous batch query results.
-------------------------------------------
Batch: 0
-------------------------------------------
+------------------+------+------+
|country |sales |orders|
+------------------+------+------+
|India |138.80|20 |
|China |133.80|20 |
|Mexico |106.86|14 |
|Japan |100.86|14 |
|Brazil |96.87 |13 |
|Russian Federation|94.87 |13 |
|United States |92.86 |14 |
|Nigeria |58.93 |7 |
|Philippines |58.92 |8 |
|South Africa |46.94 |6 |
|Argentina |42.93 |7 |
|Germany |39.96 |4 |
|Indonesia |38.95 |5 |
|Italy |35.95 |5 |
|Iran |33.95 |5 |
|South Korea |33.94 |6 |
|Poland |30.97 |3 |
|Pakistan |25.97 |3 |
|Taiwan |25.96 |4 |
|Mozambique |23.97 |3 |
|Ukraine |23.96 |4 |
|Vietnam |23.96 |4 |
|Venezuela |22.97 |3 |
|France |20.98 |2 |
|Peru |19.98 |2 |
+------------------+------+------+
only showing top 25 rows
Immediately below the batch output, there will be a log entry containing information about the batch. In the log entry snippet below, note the starting and ending offsets of the topic for the Spark job’s Kafka consumer group, 0 (null) to 250, representing the initial sales data.
PySpark Job 4: Incremental Sales Data
As described earlier, the second PySpark script, 04_incremental_sales_kafka.py
, reads 1,800 additional sales records from a second CSV file located on Amazon S3, sales_incremental_large.csv
. The script then publishes messages to the Kafka topic at a deliberately throttled rate of two messages per second. Concurrently, the first PySpark job, still running and performing a streaming query, will consume the new Kafka messages and stream aggregated total sales and orders in micro-batches of one-minute increments to the console over a period of about 15 minutes.
Submit the second PySpark script as a concurrent Amazon EMR Step to the first EMR cluster, or submit as a step to the second Amazon EMR cluster.
The job sends a total of 1,800 messages to Kafka at a rate of two messages per second for 15 minutes. The total runtime of the job should be approximately 19 minutes, given a few minutes for startup and shutdown. Why run for so long? We want to make sure the job’s runtime will span multiple, overlapping, sliding event-time windows.
After about two minutes, return to the terminal output of the first Spark job, 03_streaming_read_kafka_console.py
, running on the master node of the first cluster. As long as new messages are consumed every minute, you should see a new micro-batch of aggregated sales results stream to the console. Below we see an example of Batch 3, which reflects additional sales compared to Batch 0, shown previously. The results reflect the current all-time sales by country in real-time as the sales are published to Kafka.
-------------------------------------------
Batch: 5
-------------------------------------------
+------------------+------+------+
|country |sales |orders|
+------------------+------+------+
|China |473.35|65 |
|India |393.44|56 |
|Japan |292.60|40 |
|Mexico |262.64|36 |
|United States |252.65|35 |
|Russian Federation|243.65|35 |
|Brazil |220.69|31 |
|Philippines |191.75|25 |
|Indonesia |142.81|19 |
|South Africa |110.85|15 |
|Nigeria |108.86|14 |
|Argentina |89.86 |14 |
|Germany |85.89 |11 |
|Israel |68.90 |10 |
|Ukraine |65.92 |8 |
|Turkey |58.91 |9 |
|Iran |58.91 |9 |
|Saudi Arabia |56.93 |7 |
|Poland |50.94 |6 |
|Pakistan |50.93 |7 |
|Italy |48.93 |7 |
|French Polynesia |47.94 |6 |
|Peru |45.95 |5 |
|United Kingdom |45.94 |6 |
|Colombia |44.94 |6 |
+------------------+------+------+
only showing top 25 rows
If we fast forward to a later micro-batch, sometime after the second incremental sales job is completed, we should see the top 25 aggregated sales by country of 2,050 messages — 250 seed plus 1,800 incremental messages.
-------------------------------------------
Batch: 20
-------------------------------------------
+------------------+--------+------+
|country |sales |orders|
+------------------+--------+------+
|China |1,379.05|195 |
|India |1,338.10|190 |
|United States |915.69 |131 |
|Mexico |855.80 |120 |
|Japan |831.88 |112 |
|Russian Federation|723.95 |105 |
|Brazil |613.12 |88 |
|Philippines |528.27 |73 |
|Indonesia |381.46 |54 |
|Turkey |350.52 |48 |
|Argentina |298.57 |43 |
|Nigeria |294.61 |39 |
|South Africa |279.61 |39 |
|Taiwan |221.67 |33 |
|Germany |199.73 |27 |
|United Kingdom |196.75 |25 |
|Poland |182.77 |23 |
|Spain |170.77 |23 |
|Ukraine |160.79 |21 |
|Iran |160.76 |24 |
|Italy |156.79 |21 |
|Pakistan |152.78 |22 |
|Saudi Arabia |146.81 |19 |
|Venezuela |145.79 |21 |
|Colombia |144.78 |22 |
+------------------+--------+------+
only showing top 25 rows
Compare the informational output below for Batch 20 to Batch 0, previously. Note the starting offset of the Kafka consumer group on the topic is 1986, and the ending offset is 2050. This is because all messages have been consumed from the topic and aggregated. If additional messages were streamed to Kafka while the streaming job is still running, additional micro-batches would continue to be streamed to the console every one minute.
PySpark Job 5: Aggregations over Sliding Event-time Window
In the previous example, we analyzed total all-time sales in real-time (e.g., show me the current, total, all-time sales for France compared to the rest of Europe, at regular intervals). This approach is opposed to sales made during a sliding event-time window (e.g., are the total sales for the United States trending better during this current four-hour marketing promotional period than the previous promotional period). In many cases, real-time sales during a distinct period or event window is probably a more commonly tracked KPI than total all-time sales.
If we add a sliding event-time window to the PySpark script, we can easily observe the total sales and order counts made during the sliding event-time window in real-time.
.withWatermark("timestamp", "10 minutes") \
.groupBy("country",
F.window("timestamp", "10 minutes", "5 minutes")) \
.agg(F.count("amount"), F.sum("amount")) \
.orderBy(F.col("window").desc(),
F.col("sum(amount)").desc()) \
Windowed totals would not include sales (messages) present in the Kafka topic before the streaming query beginning, nor in previous sliding windows. Constructing the correct query always starts with a clear understanding of the question you are trying to answer.
Below, in the abridged console output of the micro-batch from the script, 05_streaming_read_kafka_console_window.py
, we see the results of three ten-minute sliding event-time windows with a five-minute overlap. The sales and order totals represent the volume sold during that window, with this micro-batch falling within the active current window, 19:30 to 19:40 UTC.
Plotting the total sales over time using sliding event-time windows, we will observe the results do not reflect a running total. Total sales only accumulate within a sliding window.

Compare these results to the results of the previous script, whose total sales reflect a running total.

PySpark Job 6: Streaming Query from/to Amazon MSK
The PySpark script, 06_streaming_read_kafka_kafka.py
, performs the same streaming query and grouped aggregation as the previous script, 03_streaming_read_kafka_console.py
. However, instead of outputting results to the console, the results of this job will be written to a new Kafka topic on Amazon MSK.
.format("kafka") \
.options(**options_write) \
.option("checkpointLocation", "/checkpoint/kafka/") \
Repeat the same process used with the previous script. Re-run the seed data script, 01_seed_sales_kafka.py
, but update the input topic to a new name, such as pagila.sales.spark.streaming.in
. Next, run the new script, 06_streaming_read_kafka_kafka.py
. Give the script time to start and consume the 250 seed messages from Kafka. Then, update the input topic name and re-run the incremental data PySpark script, 04_incremental_sales_kafka.py
, concurrent to the new script on the same cluster or run on the second cluster.
When run, the script, 06_streaming_read_kafka_kafka.py
, will continuously consume messages from the new pagila.sales.spark.streaming.in
topic and publish grouped aggregation results to a new topic, pagila.sales.spark.streaming.out
.
Use the Kafka Consumer API to view new messages as the Spark job publishes them in near real-time to Kafka.
export BBROKERS="b-1.your-cluster.kafka.us-east-1.amazonaws.com:9098,b-2.your-cluster.kafka.us-east-1.amazonaws.com:9098, ..."
bin/kafka-console-consumer.sh \
--topic pagila.sales.spark.streaming.out \
--from-beginning \
--property print.key=true \
--property print.value=true \
--property print.offset=true \
--property print.partition=true \
--property print.headers=true \
--property print.timestamp=true \
--bootstrap-server $BBROKERS \
--consumer.config config/client-iam.properties

PySpark Job 7: Batch Query of Streaming Results from MSK
When run, the previous script produces Kafka messages containing non-windowed sales aggregations to the Kafka topic every minute. Using the next PySpark script, 07_batch_read_kafka.py
, we can consume those aggregated messages using a batch query and display the most recent sales totals to the console. Each country’s most recent all-time sales totals and order counts should be identical to the previous script’s results, representing the aggregation of all 2,050 Kafka messages — 250 seed plus 1,800 incremental messages.
To get the latest total sales by country, we will consume all the messages from the output topic, group the results by country, find the maximum (max
) value from the sales column for each country, and finally, display the results sorted sales in descending order.
window = Window.partitionBy("country") \
.orderBy(F.col("timestamp").desc())
.withColumn("row", F.row_number().over(window)) \
.where(F.col("row") == 1).drop("row") \
.select("country", "sales", "orders") \
Writing the top 25 results to the console, we should see the same results as we saw in the final micro-batch (Batch 20, shown above) of the PySpark script, 03_streaming_read_kafka_console.py
.
+------------------+------+------+
|country |sales |orders|
+------------------+------+------+
|India |948.63|190 |
|China |936.67|195 |
|United States |915.69|131 |
|Mexico |855.80|120 |
|Japan |831.88|112 |
|Russian Federation|723.95|105 |
|Brazil |613.12|88 |
|Philippines |528.27|73 |
|Indonesia |381.46|54 |
|Turkey |350.52|48 |
|Argentina |298.57|43 |
|Nigeria |294.61|39 |
|South Africa |279.61|39 |
|Taiwan |221.67|33 |
|Germany |199.73|27 |
|United Kingdom |196.75|25 |
|Poland |182.77|23 |
|Spain |170.77|23 |
|Ukraine |160.79|21 |
|Iran |160.76|24 |
|Italy |156.79|21 |
|Pakistan |152.78|22 |
|Saudi Arabia |146.81|19 |
|Venezuela |145.79|21 |
|Colombia |144.78|22 |
+------------------+------+------+
only showing top 25 rows
PySpark Job 8: Streaming Query with Static Join and Sliding Window
The PySpark script, 08_streaming_read_kafka_join_window.py
, performs the same streaming query and computations over sliding event-time windows as the previous script, 05_streaming_read_kafka_console_window.py
. However, instead of totaling sales and orders by country, the script totals by sales and orders sales region. A sales region is composed of multiple countries in the same geographical area. The PySpark script reads in a static list of sales regions and countries from Amazon S3, sales_regions.csv
.
The script then performs a join operation between the results of the streaming query and the static list of regions, joining on country
. Using the join, the streaming sales data from Kafka is enriched with the sales category. Any sales record whose country does not have an assigned sales region is categorized as ‘Unassigned.’
.join(df_regions, on=["country"], how="leftOuter") \
.na.fill("Unassigned") \
Sales and orders are then aggregated by sales region, and the top 25 are output to the console every minute.
To run the job, repeat the previous process of renaming the topic (e.g., pagila.sales.spark.streaming.region)
, then running the initial sales data job, this script, and finally, concurrent with this script, the incremental sales data job. Below, we see a later micro-batch output to the console from the Spark job. We see three sets of sales results, by sales region, from three different ten-minute sliding event-time windows with a five-minute overlap.
PySpark Script 9: Static Join with Grouped Aggregations
As a comparison, we can exclude the sliding event-time window operations from the previous streaming query script, 08_streaming_read_kafka_join_window.py
, to obtain the current, total, all-time sales by sales region. See the script, 09_streaming_read_kafka_join.py
, in the project repository for details.
-------------------------------------------
Batch: 20
-------------------------------------------
+--------------+--------+------+
|sales_region |sales |orders|
+--------------+--------+------+
|Asia & Pacific|5,780.88|812 |
|Europe |3,081.74|426 |
|Latin America |2,545.34|366 |
|Africa |1,029.59|141 |
|North America |997.57 |143 |
|Middle east |541.23 |77 |
|Unassigned |352.47 |53 |
|Arab States |244.68 |32 |
+--------------+--------+------+
Conclusion
In this post, we learned how to get started with Spark Structured Streaming on Amazon EMR. First, we explored how to run jobs written in Python with PySpark on Amazon EMR as Steps and directly from the EMR cluster’s master node. Next, we discovered how to produce and consume messages with Apache Kafka on Amazon MSK, using batch and streaming queries. Finally, we learned about aggregations over a sliding event-time window compared to grouped aggregations and how Structured Streaming queries are processed using a micro-batch.
In a subsequent post, we will learn how to use Apache Avro and the Apicurio Registry with PySpark on Amazon EMR to read and write Apache Avro format messages to Amazon MSK.
This blog represents my own viewpoints and not of my employer, Amazon Web Services (AWS). All product names, logos, and brands are the property of their respective owners.